'Degraded' Synthetic Faces May Enhance Facial Recognition Technology
Researchers at Michigan State University have come up with an innovative way to use synthetic faces for a noble cause—enhancing the accuracy of image recognition systems. Instead of contributing to the deepfakes phenomenon, these synthetic faces are designed to mimic the imperfections found in real-world video surveillance footage.
The team has developed a Controllable Face Synthesis Module (CFSM) that can regenerate faces in a style that reflects the typical flaws of CCTV systems, such as facial blur, low resolution, and sensor noise. This approach differs from using high-quality celebrity images from popular datasets, which don't capture the real-world challenges faced by facial recognition systems.
*Conceptual architecture for the Controllable Face Synthesis Module (CFSM).* Source: http://cvlab.cse.msu.edu/pdfs/Liu_Kim_Jain_Liu_ECCV2022.pdf
Unlike deepfake systems that focus on replicating head poses and expressions, CFSM aims to generate alternative views that match the style of the target recognition system through style transfer. This module is particularly useful for adapting to legacy systems that are unlikely to be upgraded due to cost constraints but still need to contribute to modern facial recognition technologies.
When testing CFSM, the researchers observed significant improvements in image recognition systems dealing with low-quality data. They also discovered an unexpected benefit: the ability to characterize and compare target datasets, which simplifies the process of benchmarking and creating tailored datasets for various CCTV systems.
*Training the facial recognition models to adapt to the limitations of the target systems.* Source: http://cvlab.cse.msu.edu/pdfs/Liu_Kim_Jain_Liu_ECCV2022_supp.pdf
The method can also be applied to existing datasets, effectively performing domain adaptation to make them more suitable for facial recognition. The research, titled **Controllable and Guided Face Synthesis for Unconstrained Face Recognition**, is partially supported by the US Office of the Director of National Intelligence (ODNI, at IARPA) and involves four researchers from MSU's Computer Science & Engineering department.
Low-Quality Face Recognition: A Growing Field
Over the past few years, low-quality face recognition (LQFR) has emerged as a significant area of study. Many older video surveillance systems, built to be durable and long-lasting, have become outdated and struggle to serve as effective data sources for machine learning due to technical debt.
Varying levels of facial resolution across a range of historic and more recent video surveillance systems. Source: https://arxiv.org/pdf/1805.11519.pdf
F fortunately, diffusion models and other noise-based models are well-suited to address this issue. Many of the latest image synthesis systems include upscaling low-resolution images as part of their process, which is also crucial for neural compression techniques.
The challenge in facial recognition is to maximize accuracy with the fewest possible features extracted from low-resolution images. This is not only useful for identifying faces at low resolution but also necessary due to limitations on image size in the training models' latent space.
In computer vision, 'features' refer to distinguishing characteristics from any image, not just faces. With the advancement in upscaling algorithms, various methods have been proposed to enhance low-resolution surveillance footage, potentially making it usable for legal purposes like crime scene investigations.
However, there's a risk of misidentification, and ideally, facial recognition systems should not require high-resolution images to make accurate identifications. Such transformations are costly and raise questions about their validity and legality.
The Need for More 'Down-At-Heel' Celebrities
It would be more beneficial if facial recognition systems could extract features directly from the output of legacy systems without needing to transform the images. This requires a better understanding of the relationship between high-resolution identities and the degraded images from existing surveillance systems.
The problem lies in the standards: datasets like MS-Celeb-1M and WebFace260M are widely used because they provide consistent benchmarks. However, the authors argue that facial recognition algorithms trained on these datasets are not suitable for the visual domains of older surveillance systems.
*Examples from Microsoft's popular MS-Celeb1m dataset.* Source: https://www.microsoft.com/en-us/research/project/ms-celeb-1m-challenge-recognizing-one-million-celebrities-real-world/
The paper highlights that state-of-the-art facial recognition models struggle with real-world surveillance imagery due to domain shift issues. These models are trained on semi-constrained datasets that lack the variations found in real-world scenarios, such as sensor noise and motion blur.
Previous methods have tried to match the outputs of historical or low-cost surveillance systems, but these were 'blind' augmentations. In contrast, CFSM uses direct feedback from the target system during training and adapts via style transfer to mimic that domain.
*Actress Natalie Portman, no stranger to the handful of datasets that dominate the computer vision community, features among the identities in this example of CFSM performing style-matched domain adaptation based on feedback from the domain of the actual target model.*
The authors' architecture uses the Fast Gradient Sign Method (FGSM) to import styles and characteristics from the target system's output. As training progresses, the image generation part of the pipeline becomes more faithful to the target system, improving the facial recognition performance and generalization capabilities.
Tests and Results
The researchers tested CFSM using MSU's prior work as a template, employing MS-Celeb-1m and MS1M-V2 as training datasets. The target data was the WiderFace dataset from the Chinese University of Hong Kong, designed for face detection in challenging situations.
The system was evaluated against four face recognition benchmarks: IJB-B, IJB-C, IJB-S, and TinyFace. CFSM was trained with about 10% of MS-Celeb-1m data, around 0.4 million images, for 125,000 iterations at a batch size of 32 using the Adam optimizer with a learning rate of 1e-4.
The target facial recognition model used a modified ResNet-50 with ArcFace loss function. An additional model was trained with CFSM for comparison, labeled as 'ArcFace' in the results.
*Results from the primary tests for CFSM. Higher numbers are better.*
The results showed that the ArcFace model, enhanced by CFSM, outperformed all baselines in both face identification and verification tasks, achieving new state-of-the-art performance.
The ability to extract domains from various characteristics of legacy surveillance systems also allows for comparing and evaluating the distribution similarity among these systems, presenting each in terms of a visual style that can be leveraged in future work.
*Examples from various datasets exhibit clear differences in style.*
The authors also noted that CFSM demonstrates how adversarial manipulation can be used to increase recognition accuracies in vision tasks. They introduced a dataset similarity metric based on learned style bases, capturing style differences in a label or predictor-agnostic way.
The research underscores the potential of controllable and guided face synthesis models for unconstrained facial recognition and provides insights into dataset differences.
Related article
專注於實惠增強現實的真實對焦系統
顛覆基於投影的增強現實技術來自著名機構電機電子工程師學會(IEEE)的研究人員在基於投影的增強現實領域取得了突破性的進展。他們的解決方案?配備了電控可變焦鏡片(ETL)的特殊眼鏡,這些鏡片模擬人類眼睛自然感知深度的方式。這種創新的方法解決了使投影系統在受控環境中真正實用的主要障礙。想像一下走進一個房間,其中投影的3D物體看起來就像周圍的家具一樣真實。這就是該
我們如何使用AI來幫助城市應對極端熱量
看起來2024年可能會打破迄今為止最熱的一年的記錄,超過了2023年。這種趨勢對生活在城市熱島的人們來說尤為艱難,這些景點是在混凝土和瀝青浸泡太陽射線,然後散發出熱量的城市中的那些景點。這些區域可以溫暖
Comments (10)
0/200
LarryWilliams
April 25, 2025 at 12:00:00 AM GMT
This tool is pretty cool! Using synthetic faces to improve facial recognition? Genius! It's nice to see tech being used for good instead of deepfakes. Only wish it was a bit more user-friendly, but still, thumbs up for the innovation! 🤓
0
FrankJackson
April 26, 2025 at 12:00:00 AM GMT
合成顔を使って顔認識技術を向上させるなんて、素晴らしいアイデアだと思う!深偽ではなく、良い目的に使われる技術は嬉しいね。ただ、もう少し使いやすければ完璧だったのに。でも、革新性には拍手を送りたい!👏
0
MatthewGonzalez
April 27, 2025 at 12:00:00 AM GMT
Que ideia genial usar faces sintéticas para melhorar o reconhecimento facial! Adoro que a tecnologia esteja sendo usada para o bem, e não para deepfakes. A única coisa é que poderia ser mais fácil de usar, mas ainda assim, inovação top! 👍
0
CharlesJohnson
April 27, 2025 at 12:00:00 AM GMT
¡Qué genial usar caras sintéticas para mejorar el reconocimiento facial! Me encanta que la tecnología se use para algo bueno y no para deepfakes. Lo único es que podría ser más fácil de usar, pero de todos modos, ¡innovación de primera! 👌
0
HarryMartínez
April 26, 2025 at 12:00:00 AM GMT
Synthetische Gesichter zur Verbesserung der Gesichtserkennung zu nutzen, ist eine geniale Idee! Es ist schön zu sehen, dass Technologie für etwas Gutes genutzt wird und nicht für Deepfakes. Es wäre nur schön, wenn es benutzerfreundlicher wäre, aber trotzdem, tolle Innovation! 👍
0
LawrencePerez
April 26, 2025 at 12:00:00 AM GMT
This sounds super interesting! Using synthetic faces to improve facial recognition tech could be a big deal. It's cool they're focusing on real-world imperfections instead of making fake stuff. I wonder how effective it'll be in practical situations though. 🤔✨
0
Researchers at Michigan State University have come up with an innovative way to use synthetic faces for a noble cause—enhancing the accuracy of image recognition systems. Instead of contributing to the deepfakes phenomenon, these synthetic faces are designed to mimic the imperfections found in real-world video surveillance footage.
The team has developed a Controllable Face Synthesis Module (CFSM) that can regenerate faces in a style that reflects the typical flaws of CCTV systems, such as facial blur, low resolution, and sensor noise. This approach differs from using high-quality celebrity images from popular datasets, which don't capture the real-world challenges faced by facial recognition systems.
*Conceptual architecture for the Controllable Face Synthesis Module (CFSM).* Source: http://cvlab.cse.msu.edu/pdfs/Liu_Kim_Jain_Liu_ECCV2022.pdf
Unlike deepfake systems that focus on replicating head poses and expressions, CFSM aims to generate alternative views that match the style of the target recognition system through style transfer. This module is particularly useful for adapting to legacy systems that are unlikely to be upgraded due to cost constraints but still need to contribute to modern facial recognition technologies.
When testing CFSM, the researchers observed significant improvements in image recognition systems dealing with low-quality data. They also discovered an unexpected benefit: the ability to characterize and compare target datasets, which simplifies the process of benchmarking and creating tailored datasets for various CCTV systems.
*Training the facial recognition models to adapt to the limitations of the target systems.* Source: http://cvlab.cse.msu.edu/pdfs/Liu_Kim_Jain_Liu_ECCV2022_supp.pdf
The method can also be applied to existing datasets, effectively performing domain adaptation to make them more suitable for facial recognition. The research, titled **Controllable and Guided Face Synthesis for Unconstrained Face Recognition**, is partially supported by the US Office of the Director of National Intelligence (ODNI, at IARPA) and involves four researchers from MSU's Computer Science & Engineering department.
Low-Quality Face Recognition: A Growing Field
Over the past few years, low-quality face recognition (LQFR) has emerged as a significant area of study. Many older video surveillance systems, built to be durable and long-lasting, have become outdated and struggle to serve as effective data sources for machine learning due to technical debt.
Varying levels of facial resolution across a range of historic and more recent video surveillance systems. Source: https://arxiv.org/pdf/1805.11519.pdf
F fortunately, diffusion models and other noise-based models are well-suited to address this issue. Many of the latest image synthesis systems include upscaling low-resolution images as part of their process, which is also crucial for neural compression techniques.
The challenge in facial recognition is to maximize accuracy with the fewest possible features extracted from low-resolution images. This is not only useful for identifying faces at low resolution but also necessary due to limitations on image size in the training models' latent space.
In computer vision, 'features' refer to distinguishing characteristics from any image, not just faces. With the advancement in upscaling algorithms, various methods have been proposed to enhance low-resolution surveillance footage, potentially making it usable for legal purposes like crime scene investigations.
However, there's a risk of misidentification, and ideally, facial recognition systems should not require high-resolution images to make accurate identifications. Such transformations are costly and raise questions about their validity and legality.
The Need for More 'Down-At-Heel' Celebrities
It would be more beneficial if facial recognition systems could extract features directly from the output of legacy systems without needing to transform the images. This requires a better understanding of the relationship between high-resolution identities and the degraded images from existing surveillance systems.
The problem lies in the standards: datasets like MS-Celeb-1M and WebFace260M are widely used because they provide consistent benchmarks. However, the authors argue that facial recognition algorithms trained on these datasets are not suitable for the visual domains of older surveillance systems.
*Examples from Microsoft's popular MS-Celeb1m dataset.* Source: https://www.microsoft.com/en-us/research/project/ms-celeb-1m-challenge-recognizing-one-million-celebrities-real-world/
The paper highlights that state-of-the-art facial recognition models struggle with real-world surveillance imagery due to domain shift issues. These models are trained on semi-constrained datasets that lack the variations found in real-world scenarios, such as sensor noise and motion blur.
Previous methods have tried to match the outputs of historical or low-cost surveillance systems, but these were 'blind' augmentations. In contrast, CFSM uses direct feedback from the target system during training and adapts via style transfer to mimic that domain.
*Actress Natalie Portman, no stranger to the handful of datasets that dominate the computer vision community, features among the identities in this example of CFSM performing style-matched domain adaptation based on feedback from the domain of the actual target model.*
The authors' architecture uses the Fast Gradient Sign Method (FGSM) to import styles and characteristics from the target system's output. As training progresses, the image generation part of the pipeline becomes more faithful to the target system, improving the facial recognition performance and generalization capabilities.
Tests and Results
The researchers tested CFSM using MSU's prior work as a template, employing MS-Celeb-1m and MS1M-V2 as training datasets. The target data was the WiderFace dataset from the Chinese University of Hong Kong, designed for face detection in challenging situations.
The system was evaluated against four face recognition benchmarks: IJB-B, IJB-C, IJB-S, and TinyFace. CFSM was trained with about 10% of MS-Celeb-1m data, around 0.4 million images, for 125,000 iterations at a batch size of 32 using the Adam optimizer with a learning rate of 1e-4.
The target facial recognition model used a modified ResNet-50 with ArcFace loss function. An additional model was trained with CFSM for comparison, labeled as 'ArcFace' in the results.
*Results from the primary tests for CFSM. Higher numbers are better.*
The results showed that the ArcFace model, enhanced by CFSM, outperformed all baselines in both face identification and verification tasks, achieving new state-of-the-art performance.
The ability to extract domains from various characteristics of legacy surveillance systems also allows for comparing and evaluating the distribution similarity among these systems, presenting each in terms of a visual style that can be leveraged in future work.
*Examples from various datasets exhibit clear differences in style.*
The authors also noted that CFSM demonstrates how adversarial manipulation can be used to increase recognition accuracies in vision tasks. They introduced a dataset similarity metric based on learned style bases, capturing style differences in a label or predictor-agnostic way.
The research underscores the potential of controllable and guided face synthesis models for unconstrained facial recognition and provides insights into dataset differences.
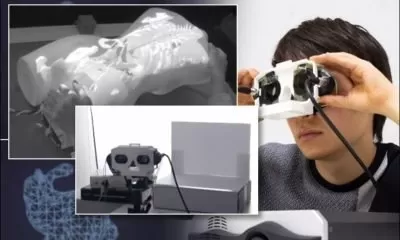
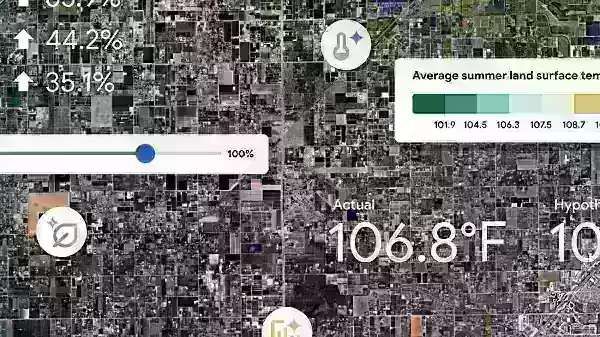

This tool is pretty cool! Using synthetic faces to improve facial recognition? Genius! It's nice to see tech being used for good instead of deepfakes. Only wish it was a bit more user-friendly, but still, thumbs up for the innovation! 🤓




合成顔を使って顔認識技術を向上させるなんて、素晴らしいアイデアだと思う!深偽ではなく、良い目的に使われる技術は嬉しいね。ただ、もう少し使いやすければ完璧だったのに。でも、革新性には拍手を送りたい!👏




Que ideia genial usar faces sintéticas para melhorar o reconhecimento facial! Adoro que a tecnologia esteja sendo usada para o bem, e não para deepfakes. A única coisa é que poderia ser mais fácil de usar, mas ainda assim, inovação top! 👍




¡Qué genial usar caras sintéticas para mejorar el reconocimiento facial! Me encanta que la tecnología se use para algo bueno y no para deepfakes. Lo único es que podría ser más fácil de usar, pero de todos modos, ¡innovación de primera! 👌




Synthetische Gesichter zur Verbesserung der Gesichtserkennung zu nutzen, ist eine geniale Idee! Es ist schön zu sehen, dass Technologie für etwas Gutes genutzt wird und nicht für Deepfakes. Es wäre nur schön, wenn es benutzerfreundlicher wäre, aber trotzdem, tolle Innovation! 👍




This sounds super interesting! Using synthetic faces to improve facial recognition tech could be a big deal. It's cool they're focusing on real-world imperfections instead of making fake stuff. I wonder how effective it'll be in practical situations though. 🤔✨



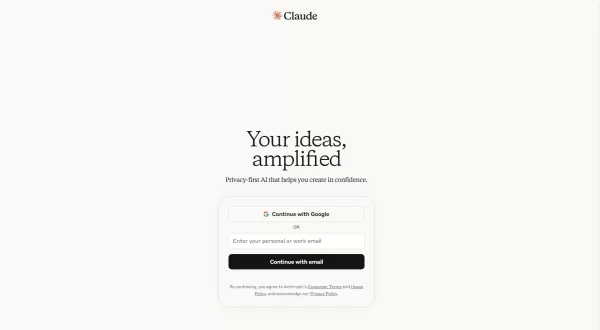
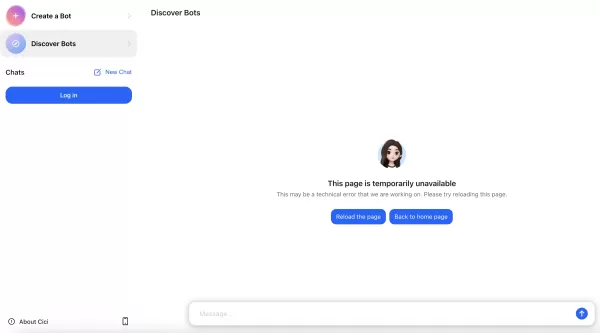
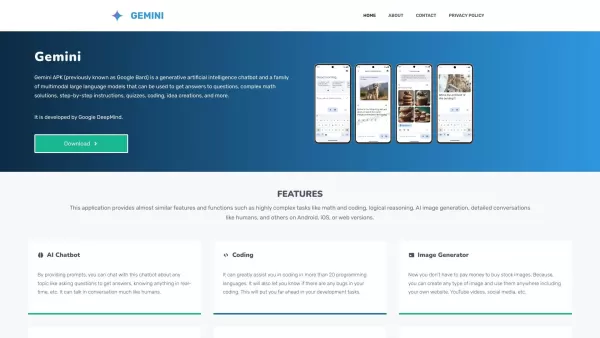
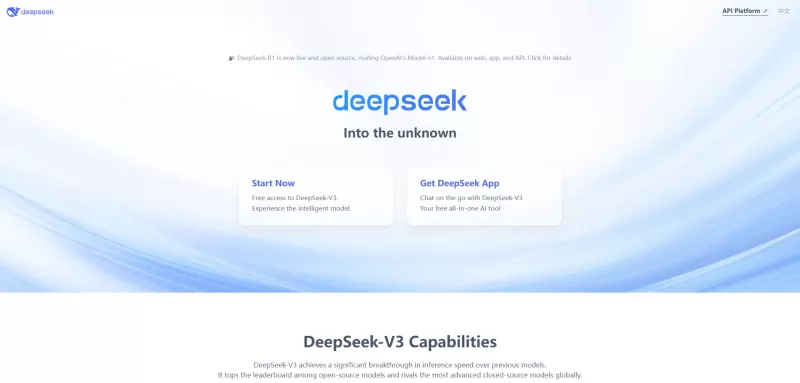
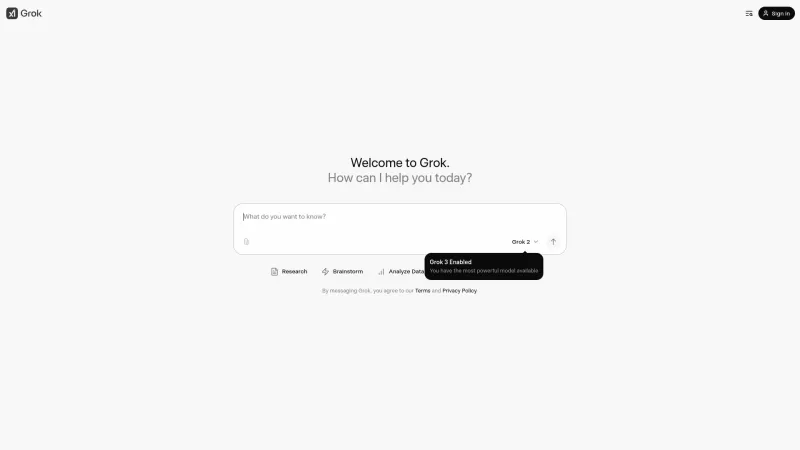
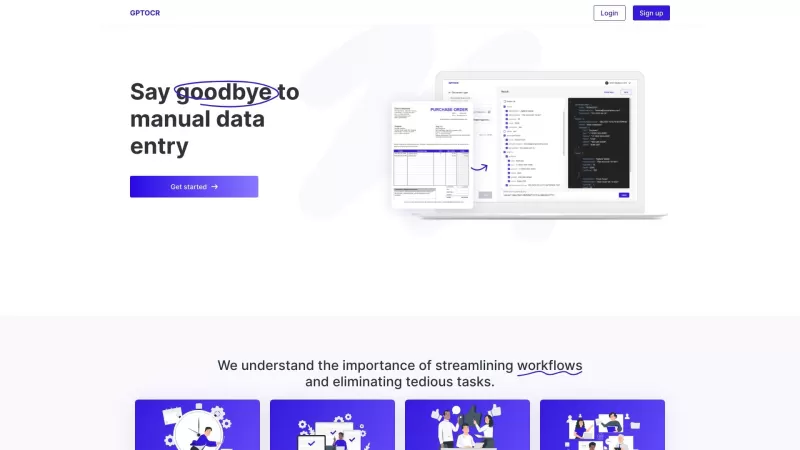
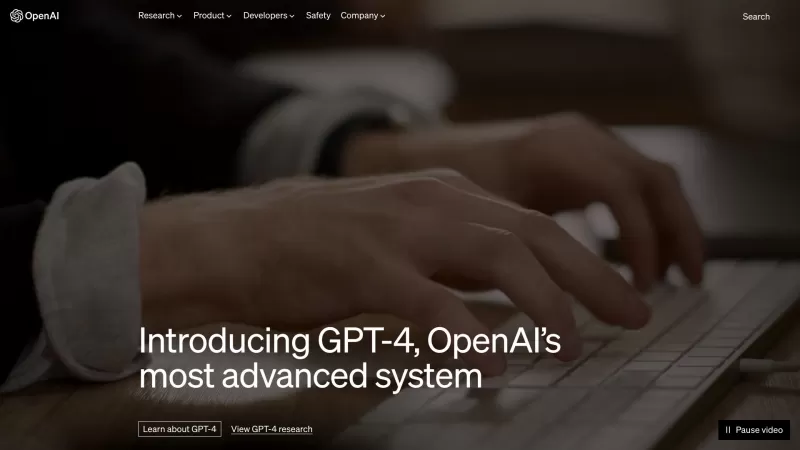
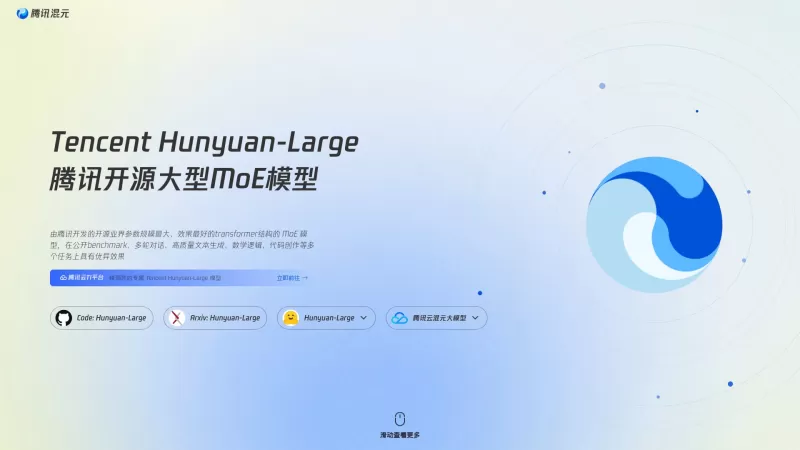
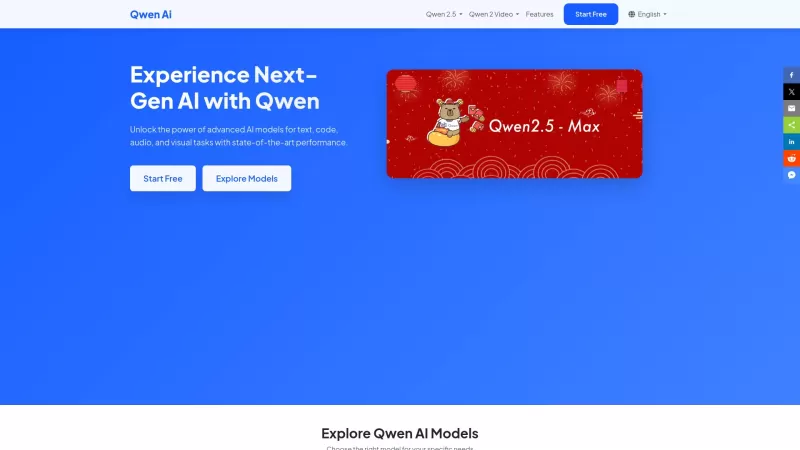
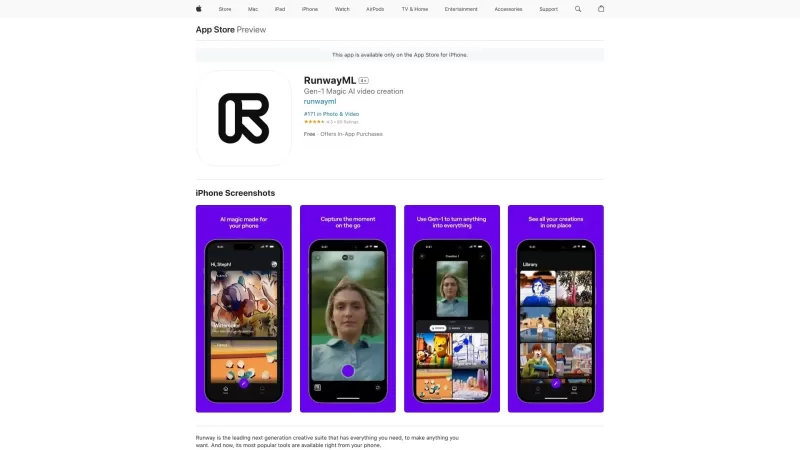