GAIA Introduces New Benchmark in Quest for True Intelligence Beyond ARC-AGI
May 2, 2025
MatthewCarter
0
Intelligence is everywhere, yet gauging it accurately feels like trying to catch a cloud with your bare hands. We use tests and benchmarks, like college entrance exams, to get a rough idea. Each year, students cram for these tests, sometimes even scoring a perfect 100%. But does that perfect score mean they all possess the same level of intelligence or that they've reached the peak of their mental potential? Of course not. These benchmarks are just rough estimates, not precise indicators of someone's true abilities.
In the world of generative AI, benchmarks such as MMLU (Massive Multitask Language Understanding) have been the go-to for assessing models through multiple-choice questions across various academic fields. While they allow for easy comparisons, they don't really capture the full spectrum of intelligent capabilities.
Take Claude 3.5 Sonnet and GPT-4.5, for example. They might score similarly on MMLU, suggesting they're on par. But anyone who's actually used these models knows their real-world performance can be quite different.
What Does It Mean to Measure 'Intelligence' in AI?
With the recent launch of the ARC-AGI benchmark, designed to test models on general reasoning and creative problem-solving, there's been a fresh wave of discussion about what it means to measure "intelligence" in AI. Not everyone has had a chance to dive into ARC-AGI yet, but the industry is buzzing about this and other new approaches to testing. Every benchmark has its place, and ARC-AGI is a step in the right direction.
Another exciting development is 'Humanity's Last Exam,' a comprehensive benchmark with 3,000 peer-reviewed, multi-step questions spanning different disciplines. It's an ambitious effort to push AI systems to expert-level reasoning. Early results show rapid progress, with OpenAI reportedly hitting a 26.6% score just a month after its release. But like other benchmarks, it focuses mainly on knowledge and reasoning in a vacuum, not on the practical, tool-using skills that are vital for real-world AI applications.
Take, for instance, how some top models struggle with simple tasks like counting the "r"s in "strawberry" or comparing 3.8 to 3.1111. These errors, which even a child or a basic calculator could avoid, highlight the gap between benchmark success and real-world reliability. It's a reminder that intelligence isn't just about acing tests; it's about navigating everyday logic with ease.
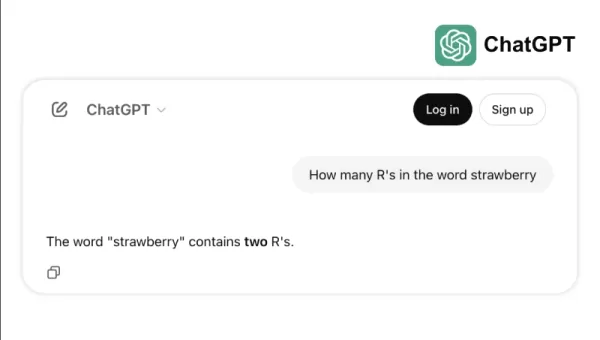
The New Standard for Measuring AI Capability
As AI models have evolved, the limitations of traditional benchmarks have become more apparent. For instance, GPT-4, when equipped with tools, only scores about 15% on the more complex, real-world tasks in the GAIA benchmark, despite its high scores on multiple-choice tests.
This discrepancy between benchmark performance and practical capability is increasingly problematic as AI systems transition from research labs to business applications. Traditional benchmarks test how well a model can recall information but often overlook key aspects of intelligence, such as the ability to gather data, run code, analyze information, and create solutions across various domains.
Enter GAIA, a new benchmark that marks a significant shift in AI evaluation. Developed through a collaboration between teams from Meta-FAIR, Meta-GenAI, HuggingFace, and AutoGPT, GAIA includes 466 meticulously crafted questions across three difficulty levels. These questions test a wide range of skills essential for real-world AI applications, including web browsing, multi-modal understanding, code execution, file handling, and complex reasoning.
Level 1 questions typically require about 5 steps and one tool for humans to solve. Level 2 questions need 5 to 10 steps and multiple tools, while Level 3 questions might demand up to 50 steps and any number of tools. This structure reflects the complexity of actual business problems, where solutions often involve multiple actions and tools.
By focusing on flexibility rather than just complexity, an AI model achieved a 75% accuracy rate on GAIA, outperforming industry leaders like Microsoft's Magnetic-1 (38%) and Google's Langfun Agent (49%). This success comes from using a mix of specialized models for audio-visual understanding and reasoning, with Anthropic's Sonnet 3.5 as the main model.
This shift in AI evaluation reflects a broader trend in the industry: We're moving away from standalone SaaS applications towards AI agents that can manage multiple tools and workflows. As businesses increasingly depend on AI to tackle complex, multi-step tasks, benchmarks like GAIA offer a more relevant measure of capability than traditional multiple-choice tests.
The future of AI evaluation isn't about isolated knowledge tests; it's about comprehensive assessments of problem-solving ability. GAIA sets a new benchmark for measuring AI capability—one that aligns better with the real-world challenges and opportunities of AI deployment.
Sri Ambati is the founder and CEO of H2O.ai.
Related article
AI Startup Secures $7.5M to Revolutionize Commercial Insurance for 24M Underprotected Small Businesses in America
1Fort, a New York-based startup, has secured a $7.5 million seed funding round to revolutionize how small businesses secure commercial insurance through its AI-driven platform. With a staggering 200% month-over-month revenue growth in 2024, 1Fort is set to overhaul the outdated manual processes that
Meta Defends Llama 4 Release, Cites Bugs as Cause of Mixed Quality Reports
Over the weekend, Meta, the powerhouse behind Facebook, Instagram, WhatsApp, and Quest VR, surprised everyone by unveiling their latest AI language model, Llama 4. Not just one, but three new versions were introduced, each boasting enhanced capabilities thanks to the "Mixture-of-Experts" architectur
Google launches Gemini in Android Studio for Businesses, making it easier for devs to design work apps
Apple may still rule the U.S. smartphone market, but Google's Android OS has won over enterprises and businesses with its flexibility and affordability. A recent Stratix survey revealed that a whopping 60% of corporate devices now run on Android tech. And Google isn't stopping there; they're set on
Comments (0)
0/200






Intelligence is everywhere, yet gauging it accurately feels like trying to catch a cloud with your bare hands. We use tests and benchmarks, like college entrance exams, to get a rough idea. Each year, students cram for these tests, sometimes even scoring a perfect 100%. But does that perfect score mean they all possess the same level of intelligence or that they've reached the peak of their mental potential? Of course not. These benchmarks are just rough estimates, not precise indicators of someone's true abilities.
In the world of generative AI, benchmarks such as MMLU (Massive Multitask Language Understanding) have been the go-to for assessing models through multiple-choice questions across various academic fields. While they allow for easy comparisons, they don't really capture the full spectrum of intelligent capabilities.
Take Claude 3.5 Sonnet and GPT-4.5, for example. They might score similarly on MMLU, suggesting they're on par. But anyone who's actually used these models knows their real-world performance can be quite different.
What Does It Mean to Measure 'Intelligence' in AI?
With the recent launch of the ARC-AGI benchmark, designed to test models on general reasoning and creative problem-solving, there's been a fresh wave of discussion about what it means to measure "intelligence" in AI. Not everyone has had a chance to dive into ARC-AGI yet, but the industry is buzzing about this and other new approaches to testing. Every benchmark has its place, and ARC-AGI is a step in the right direction.
Another exciting development is 'Humanity's Last Exam,' a comprehensive benchmark with 3,000 peer-reviewed, multi-step questions spanning different disciplines. It's an ambitious effort to push AI systems to expert-level reasoning. Early results show rapid progress, with OpenAI reportedly hitting a 26.6% score just a month after its release. But like other benchmarks, it focuses mainly on knowledge and reasoning in a vacuum, not on the practical, tool-using skills that are vital for real-world AI applications.
Take, for instance, how some top models struggle with simple tasks like counting the "r"s in "strawberry" or comparing 3.8 to 3.1111. These errors, which even a child or a basic calculator could avoid, highlight the gap between benchmark success and real-world reliability. It's a reminder that intelligence isn't just about acing tests; it's about navigating everyday logic with ease.
The New Standard for Measuring AI Capability
As AI models have evolved, the limitations of traditional benchmarks have become more apparent. For instance, GPT-4, when equipped with tools, only scores about 15% on the more complex, real-world tasks in the GAIA benchmark, despite its high scores on multiple-choice tests.
This discrepancy between benchmark performance and practical capability is increasingly problematic as AI systems transition from research labs to business applications. Traditional benchmarks test how well a model can recall information but often overlook key aspects of intelligence, such as the ability to gather data, run code, analyze information, and create solutions across various domains.
Enter GAIA, a new benchmark that marks a significant shift in AI evaluation. Developed through a collaboration between teams from Meta-FAIR, Meta-GenAI, HuggingFace, and AutoGPT, GAIA includes 466 meticulously crafted questions across three difficulty levels. These questions test a wide range of skills essential for real-world AI applications, including web browsing, multi-modal understanding, code execution, file handling, and complex reasoning.
Level 1 questions typically require about 5 steps and one tool for humans to solve. Level 2 questions need 5 to 10 steps and multiple tools, while Level 3 questions might demand up to 50 steps and any number of tools. This structure reflects the complexity of actual business problems, where solutions often involve multiple actions and tools.
By focusing on flexibility rather than just complexity, an AI model achieved a 75% accuracy rate on GAIA, outperforming industry leaders like Microsoft's Magnetic-1 (38%) and Google's Langfun Agent (49%). This success comes from using a mix of specialized models for audio-visual understanding and reasoning, with Anthropic's Sonnet 3.5 as the main model.
This shift in AI evaluation reflects a broader trend in the industry: We're moving away from standalone SaaS applications towards AI agents that can manage multiple tools and workflows. As businesses increasingly depend on AI to tackle complex, multi-step tasks, benchmarks like GAIA offer a more relevant measure of capability than traditional multiple-choice tests.
The future of AI evaluation isn't about isolated knowledge tests; it's about comprehensive assessments of problem-solving ability. GAIA sets a new benchmark for measuring AI capability—one that aligns better with the real-world challenges and opportunities of AI deployment.
Sri Ambati is the founder and CEO of H2O.ai.
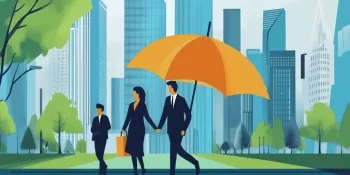
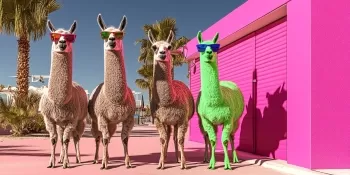
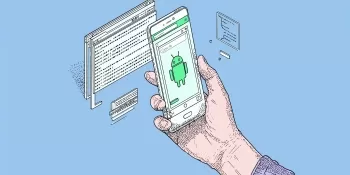
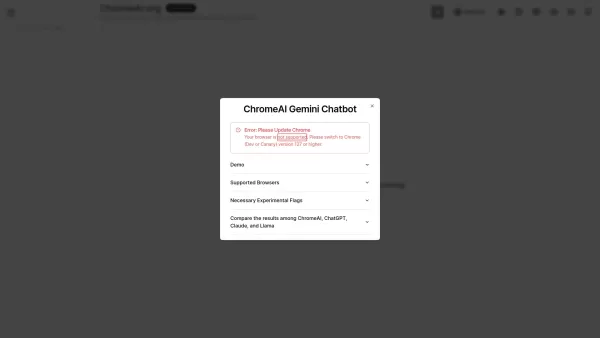
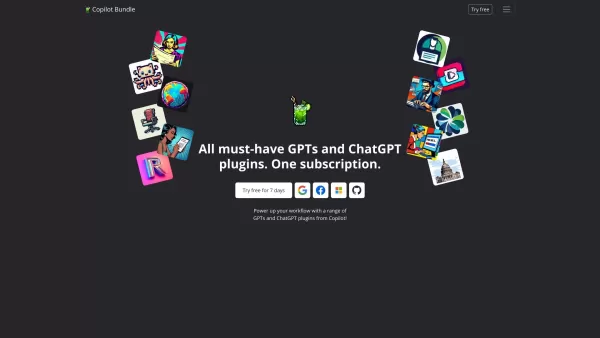
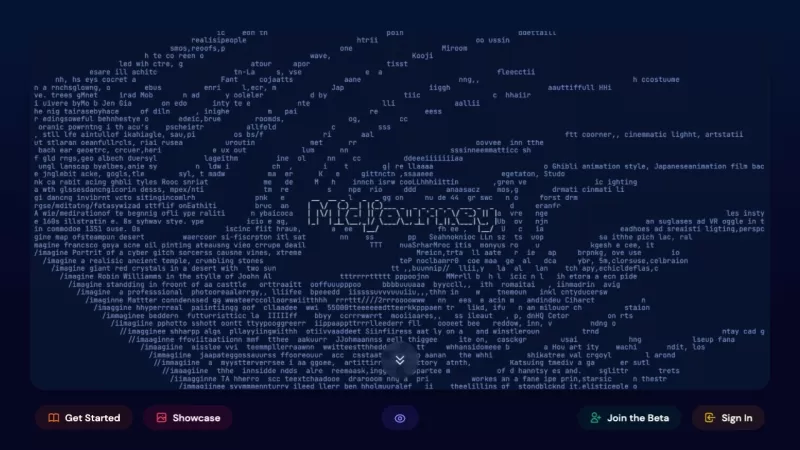
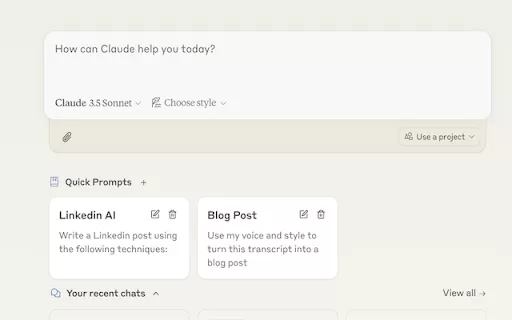
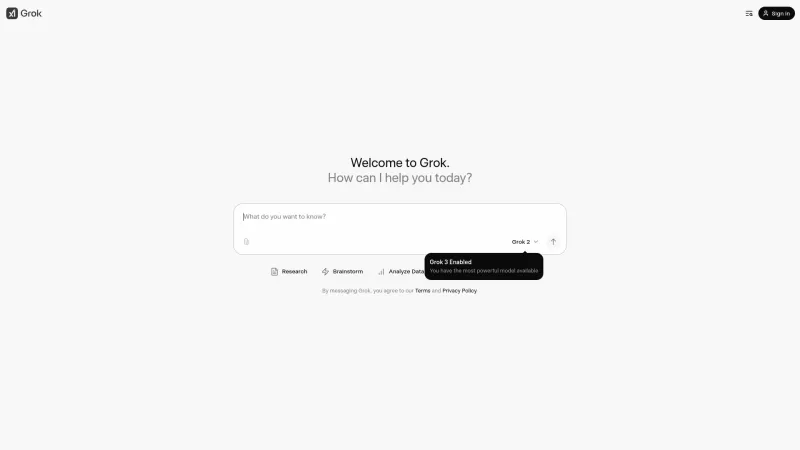
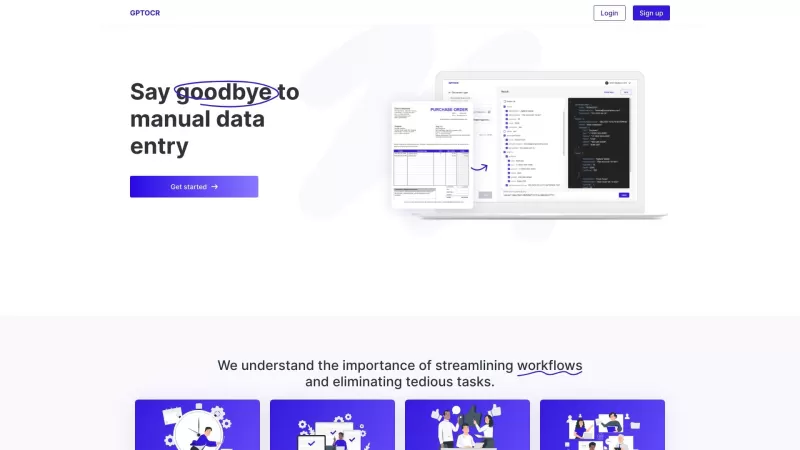
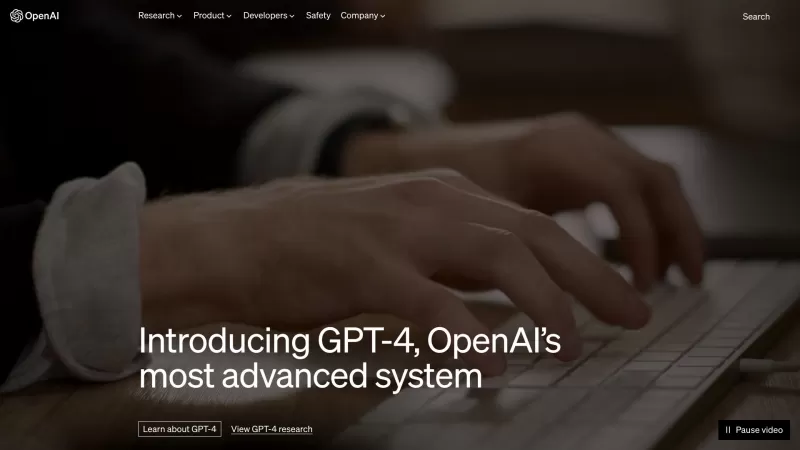
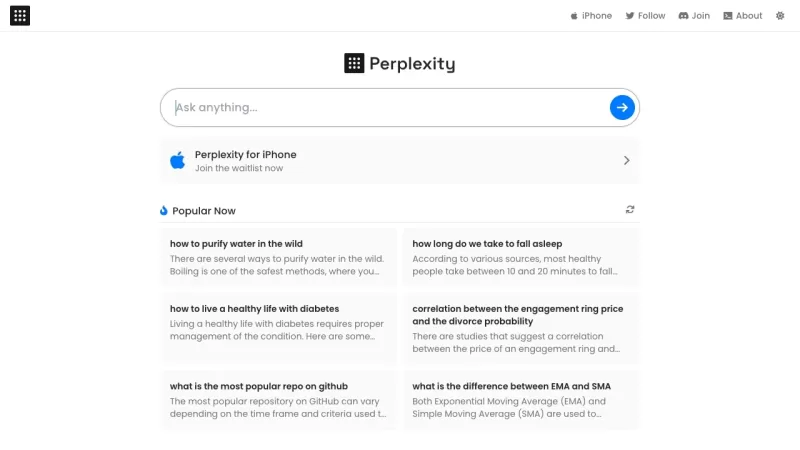
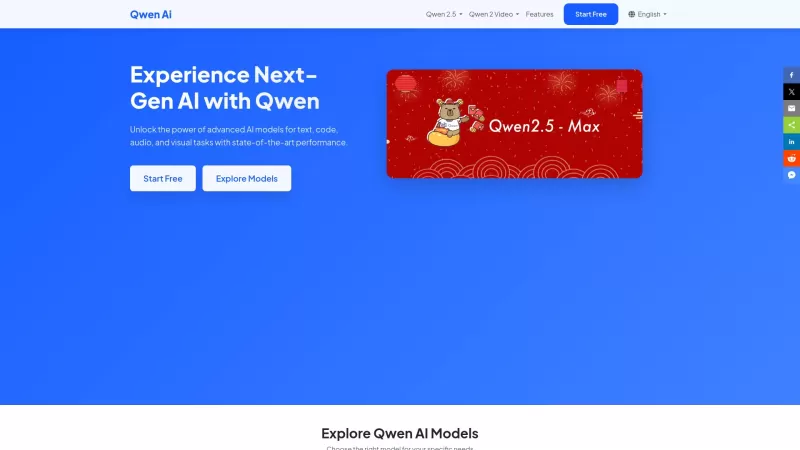
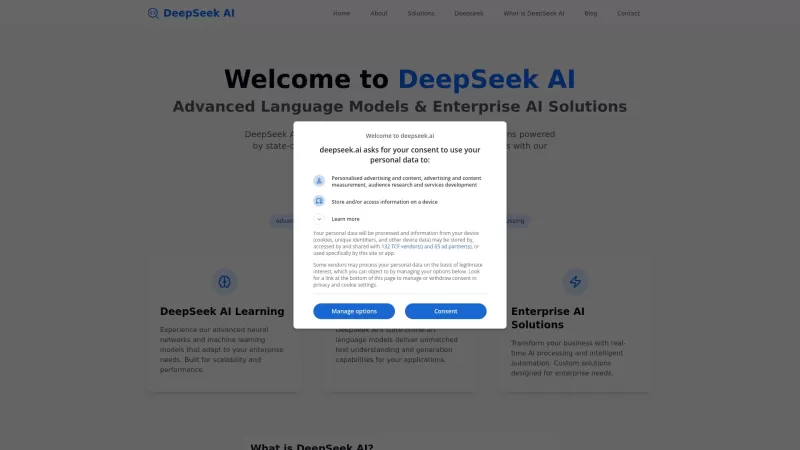