Experts Highlight Serious Flaws in Crowdsourced AI Benchmarks
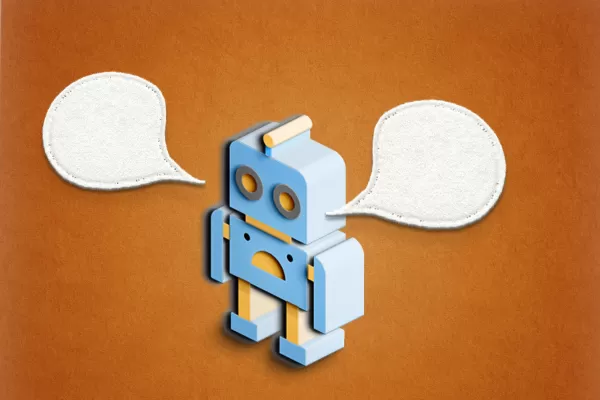
Os laboratórios de IA estão cada vez mais recorrendo a plataformas de benchmarking de crowdsourcing, como o Chatbot Arena, para avaliar as capacidades de seus modelos mais recentes. No entanto, alguns especialistas argumentam que esse método levanta preocupações éticas e acadêmicas significativas.
Nos últimos anos, grandes players como OpenAi, Google e Meta utilizaram plataformas que envolvem os usuários para avaliar o desempenho de seus próximos modelos. Uma pontuação alta nessas plataformas é frequentemente destacada pelos laboratórios como uma prova do avanço de seu modelo. No entanto, essa abordagem não deixa de ter seus críticos.
A crítica do benchmarking de crowdsourcing
Emily Bender, professora de linguística da Universidade de Washington e co-autor de "The Ai Con", manifestou preocupações sobre a validade de tais benchmarks, particularmente chatbot arena. Essa plataforma envolve voluntários comparando respostas de dois modelos anônimos e escolhendo o preferido. Bender argumenta que, para que uma referência seja eficaz, ele deve medir algo específico e demonstrar validade de construção, o que significa que a medição deve refletir com precisão o construto que está sendo avaliado. Ela afirma que o Chatbot Arena carece de evidências de que as preferências do usuário por uma saída sobre outra se correlacionam genuinamente com qualquer critério definido.
Asmelash Teka Hadgu, co-fundadora da empresa de IA Lesan e bolsista do Instituto de Pesquisa Distribuído da IA, sugere que esses parâmetros de referência estão sendo explorados pela IA Labs para fazer reivindicações exageradas sobre seus modelos. Ele citou um incidente recente com o modelo Maverick da Meta Llama 4, onde meta ajustou uma versão para ter um bom desempenho na Chatbot Arena, mas optou por lançar uma versão menos eficaz. O Hadgu defende os benchmarks a serem dinâmicos, distribuídos em várias entidades independentes e adaptadas a casos de uso específicos em campos como educação e saúde por profissionais que usam esses modelos em seu trabalho.
A chamada para métodos de remuneração justa e avaliação mais amplos
Hadgu e Kristine Gloria, ex -líder da iniciativa emergente e inteligente de tecnologias do Aspen Institute, argumentam que os avaliadores devem ser compensados por seu trabalho, atraindo paralelos à indústria de rotulagem de dados frequentemente exploradores. A Gloria vê o benchmarking de crowdsourcing como valioso, semelhante às iniciativas científicas do cidadão, mas enfatiza que os benchmarks não devem ser a única métrica para avaliação, especialmente devido ao rápido ritmo da inovação da indústria.
Matt Fredrikson, CEO da Gray Swan AI, que conduz campanhas de equipes Red Red, reconhece o apelo de tais plataformas para voluntários que procuram aprender e praticar novas habilidades. No entanto, ele enfatiza que os benchmarks públicos não podem substituir as avaliações mais aprofundadas fornecidas por avaliações privadas pagas. Fredrikson sugere que os desenvolvedores também devem confiar em benchmarks internos, equipes vermelhas algorítmicas e especialistas contratados que podem oferecer informações mais abertas e específicas de domínio.
Perspectivas da indústria sobre benchmarking
Alex Atallah, CEO da Model Marketplace OpenRouter, e Wei-Lin Chiang, um estudante de doutorado da AI da UC Berkeley e um dos fundadores da Lmarena (que gerencia o Chatbot Arena), concorda que testes abertos e benchmarking somente são insuficientes. Chiang enfatiza que o objetivo de Lmarena é fornecer um espaço aberto e confiável para medir preferências da comunidade sobre diferentes modelos de IA.
Abordando a controvérsia em torno da referência do Maverick, Chiang esclarece que esses incidentes não são devidos a falhas no design da Chatbot Arena, mas sim interpretações errôneas de suas políticas pelos laboratórios. Desde então, a Lmarena atualizou suas políticas para garantir avaliações justas e reproduzíveis. Chiang ressalta que a comunidade da plataforma não é apenas um grupo de voluntários ou testadores, mas um grupo engajado que fornece feedback coletivo sobre os modelos de IA.
O debate em andamento sobre o uso de plataformas de benchmarking de crowdsourcing destaca a necessidade de uma abordagem mais sutil à avaliação do modelo de IA, que combina informações públicas com avaliações profissionais rigorosas para garantir a precisão e a justiça.
Related article
Online Poker Strategy: How AI is Changing the Game & Expert Tips to Win
Online Poker in 2025: Is AI Taking Over? A Complete GuideThe online poker world is changing fast. With AI like ChatGPT becoming more advanced, players are asking: Is poker getting easier, or are bots taking over? This guide cuts through the noise, examining whether AI is a real threat and providing
Amazon Rufus: Revolutionizes Online Shopping as Your Personal AI Assistant
Amazon Rufus: How AI is Revolutionizing Online ShoppingThe e-commerce landscape is changing at lightning speed, and artificial intelligence (AI) is leading the charge. Amazon, always at the cutting edge of online retail, has introduced Rufus—an AI-powered shopping assistant designed to transform how
AI Voice Model Jobs: Safeguarding Your Vocal Persona as a Voice Actor
AI Voice Models & the Ethical Battle for Voice Actors’ RightsThe rapid advancement of AI voice technology is reshaping the entertainment industry—but at what cost? While AI-generated voices open new creative possibilities, they also raise urgent ethical questions about consent, fair compensation, an
Comments (10)
0/200
RogerRodriguez
April 27, 2025 at 12:00:00 AM GMT
I've been following the debate on crowdsourced AI benchmarks and honestly, it's a mess. Experts are right to point out the flaws, but what's the alternative? It's like trying to fix a leaky boat with more holes. Still, it's an interesting read and definitely makes you think about the future of AI ethics. Give it a go if you're into that kinda stuff! 😅
0
BruceClark
April 26, 2025 at 12:00:00 AM GMT
クラウドソーシングAIベンチマークの問題点についての議論を追っていますが、正直、混乱しています。専門家が指摘する欠陥は正しいと思いますが、代替案は何ですか?漏れ続ける船をさらに穴だらけにするようなものです。それでも、興味深い読み物で、AI倫理の未来について考えさせられます。これに興味があるなら、試してみてください!😅
0
WillLopez
April 26, 2025 at 12:00:00 AM GMT
크라우드소싱 AI 벤치마크의 문제점에 대한 논의를 따라가고 있는데, 솔직히 혼란스럽습니다. 전문가들이 지적하는 결함은 맞다고 생각하지만, 대안은 무엇인가요? 구멍이 더 나는 배를 고치는 것 같아요. 그래도 흥미로운 읽을거리이고, AI 윤리의 미래에 대해 생각하게 합니다. 이런 것에 관심이 있으면 해보세요! 😅
0
JonathanAllen
April 27, 2025 at 12:00:00 AM GMT
Estou acompanhando o debate sobre benchmarks de IA crowdsourced e, honestamente, é uma bagunça. Os especialistas têm razão ao apontar as falhas, mas qual é a alternativa? É como tentar consertar um barco que vaza com mais buracos. Ainda assim, é uma leitura interessante e certamente faz você pensar sobre o futuro da ética em IA. Experimente se você gosta desse tipo de coisa! 😅
0
AlbertLee
April 25, 2025 at 12:00:00 AM GMT
He estado siguiendo el debate sobre los benchmarks de IA crowdsourced y, sinceramente, es un desastre. Los expertos tienen razón al señalar las fallas, pero ¿cuál es la alternativa? Es como intentar arreglar un barco que hace agua con más agujeros. Aún así, es una lectura interesante y definitivamente te hace pensar en el futuro de la ética en IA. Pruébalo si te interesa este tipo de cosas! 😅
0
NoahGreen
April 26, 2025 at 12:00:00 AM GMT
I get the concerns about crowdsourced benchmarks, but this tool really lays it out there. It's eye-opening to see the flaws in these systems, though it's a bit heavy on the ethical side. Could use more on how to improve them, but definitely a must-read for anyone in AI! 📚
0
Os laboratórios de IA estão cada vez mais recorrendo a plataformas de benchmarking de crowdsourcing, como o Chatbot Arena, para avaliar as capacidades de seus modelos mais recentes. No entanto, alguns especialistas argumentam que esse método levanta preocupações éticas e acadêmicas significativas.
Nos últimos anos, grandes players como OpenAi, Google e Meta utilizaram plataformas que envolvem os usuários para avaliar o desempenho de seus próximos modelos. Uma pontuação alta nessas plataformas é frequentemente destacada pelos laboratórios como uma prova do avanço de seu modelo. No entanto, essa abordagem não deixa de ter seus críticos.
A crítica do benchmarking de crowdsourcing
Emily Bender, professora de linguística da Universidade de Washington e co-autor de "The Ai Con", manifestou preocupações sobre a validade de tais benchmarks, particularmente chatbot arena. Essa plataforma envolve voluntários comparando respostas de dois modelos anônimos e escolhendo o preferido. Bender argumenta que, para que uma referência seja eficaz, ele deve medir algo específico e demonstrar validade de construção, o que significa que a medição deve refletir com precisão o construto que está sendo avaliado. Ela afirma que o Chatbot Arena carece de evidências de que as preferências do usuário por uma saída sobre outra se correlacionam genuinamente com qualquer critério definido.
Asmelash Teka Hadgu, co-fundadora da empresa de IA Lesan e bolsista do Instituto de Pesquisa Distribuído da IA, sugere que esses parâmetros de referência estão sendo explorados pela IA Labs para fazer reivindicações exageradas sobre seus modelos. Ele citou um incidente recente com o modelo Maverick da Meta Llama 4, onde meta ajustou uma versão para ter um bom desempenho na Chatbot Arena, mas optou por lançar uma versão menos eficaz. O Hadgu defende os benchmarks a serem dinâmicos, distribuídos em várias entidades independentes e adaptadas a casos de uso específicos em campos como educação e saúde por profissionais que usam esses modelos em seu trabalho.
A chamada para métodos de remuneração justa e avaliação mais amplos
Hadgu e Kristine Gloria, ex -líder da iniciativa emergente e inteligente de tecnologias do Aspen Institute, argumentam que os avaliadores devem ser compensados por seu trabalho, atraindo paralelos à indústria de rotulagem de dados frequentemente exploradores. A Gloria vê o benchmarking de crowdsourcing como valioso, semelhante às iniciativas científicas do cidadão, mas enfatiza que os benchmarks não devem ser a única métrica para avaliação, especialmente devido ao rápido ritmo da inovação da indústria.
Matt Fredrikson, CEO da Gray Swan AI, que conduz campanhas de equipes Red Red, reconhece o apelo de tais plataformas para voluntários que procuram aprender e praticar novas habilidades. No entanto, ele enfatiza que os benchmarks públicos não podem substituir as avaliações mais aprofundadas fornecidas por avaliações privadas pagas. Fredrikson sugere que os desenvolvedores também devem confiar em benchmarks internos, equipes vermelhas algorítmicas e especialistas contratados que podem oferecer informações mais abertas e específicas de domínio.
Perspectivas da indústria sobre benchmarking
Alex Atallah, CEO da Model Marketplace OpenRouter, e Wei-Lin Chiang, um estudante de doutorado da AI da UC Berkeley e um dos fundadores da Lmarena (que gerencia o Chatbot Arena), concorda que testes abertos e benchmarking somente são insuficientes. Chiang enfatiza que o objetivo de Lmarena é fornecer um espaço aberto e confiável para medir preferências da comunidade sobre diferentes modelos de IA.
Abordando a controvérsia em torno da referência do Maverick, Chiang esclarece que esses incidentes não são devidos a falhas no design da Chatbot Arena, mas sim interpretações errôneas de suas políticas pelos laboratórios. Desde então, a Lmarena atualizou suas políticas para garantir avaliações justas e reproduzíveis. Chiang ressalta que a comunidade da plataforma não é apenas um grupo de voluntários ou testadores, mas um grupo engajado que fornece feedback coletivo sobre os modelos de IA.
O debate em andamento sobre o uso de plataformas de benchmarking de crowdsourcing destaca a necessidade de uma abordagem mais sutil à avaliação do modelo de IA, que combina informações públicas com avaliações profissionais rigorosas para garantir a precisão e a justiça.
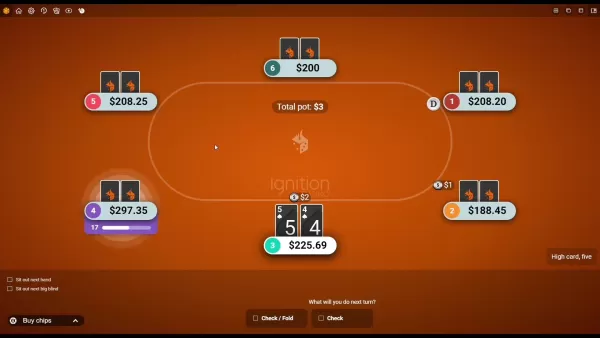
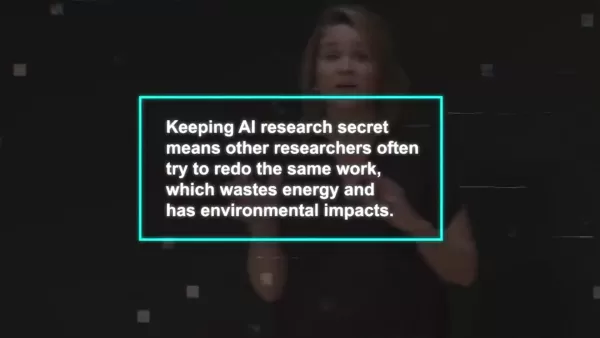
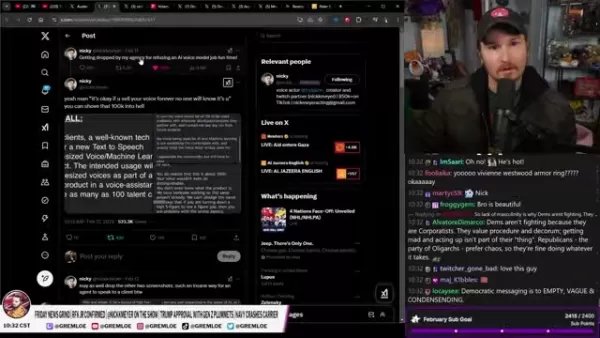

I've been following the debate on crowdsourced AI benchmarks and honestly, it's a mess. Experts are right to point out the flaws, but what's the alternative? It's like trying to fix a leaky boat with more holes. Still, it's an interesting read and definitely makes you think about the future of AI ethics. Give it a go if you're into that kinda stuff! 😅




クラウドソーシングAIベンチマークの問題点についての議論を追っていますが、正直、混乱しています。専門家が指摘する欠陥は正しいと思いますが、代替案は何ですか?漏れ続ける船をさらに穴だらけにするようなものです。それでも、興味深い読み物で、AI倫理の未来について考えさせられます。これに興味があるなら、試してみてください!😅




크라우드소싱 AI 벤치마크의 문제점에 대한 논의를 따라가고 있는데, 솔직히 혼란스럽습니다. 전문가들이 지적하는 결함은 맞다고 생각하지만, 대안은 무엇인가요? 구멍이 더 나는 배를 고치는 것 같아요. 그래도 흥미로운 읽을거리이고, AI 윤리의 미래에 대해 생각하게 합니다. 이런 것에 관심이 있으면 해보세요! 😅




Estou acompanhando o debate sobre benchmarks de IA crowdsourced e, honestamente, é uma bagunça. Os especialistas têm razão ao apontar as falhas, mas qual é a alternativa? É como tentar consertar um barco que vaza com mais buracos. Ainda assim, é uma leitura interessante e certamente faz você pensar sobre o futuro da ética em IA. Experimente se você gosta desse tipo de coisa! 😅




He estado siguiendo el debate sobre los benchmarks de IA crowdsourced y, sinceramente, es un desastre. Los expertos tienen razón al señalar las fallas, pero ¿cuál es la alternativa? Es como intentar arreglar un barco que hace agua con más agujeros. Aún así, es una lectura interesante y definitivamente te hace pensar en el futuro de la ética en IA. Pruébalo si te interesa este tipo de cosas! 😅




I get the concerns about crowdsourced benchmarks, but this tool really lays it out there. It's eye-opening to see the flaws in these systems, though it's a bit heavy on the ethical side. Could use more on how to improve them, but definitely a must-read for anyone in AI! 📚



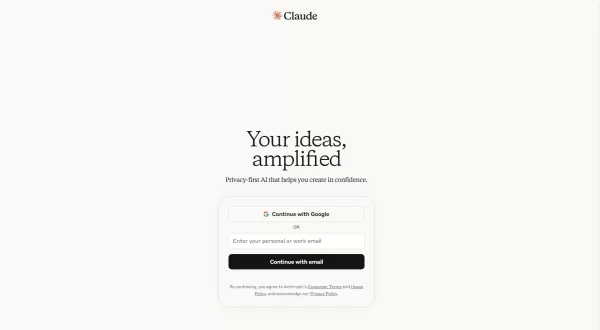
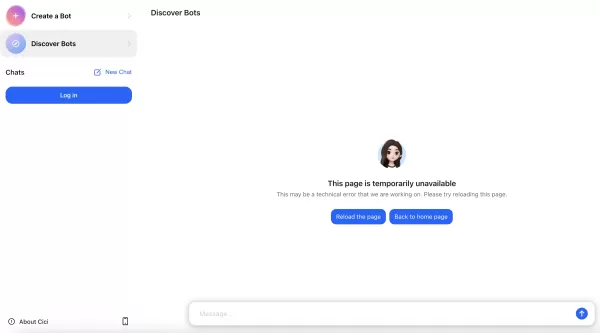
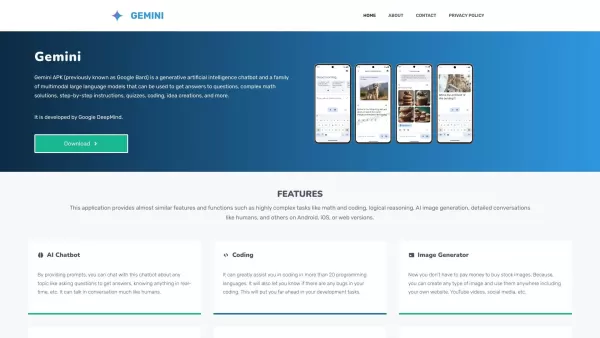
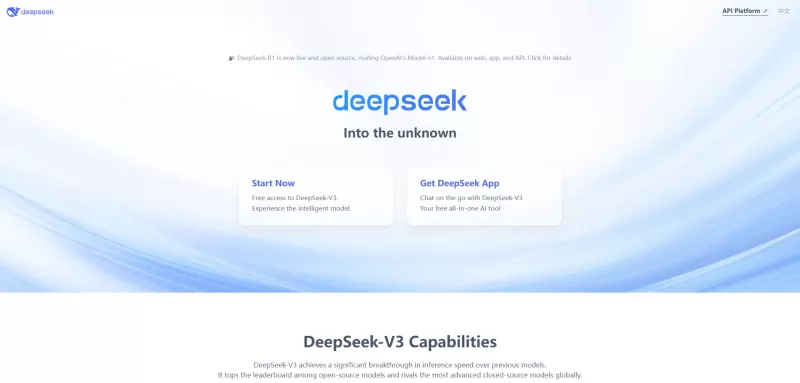
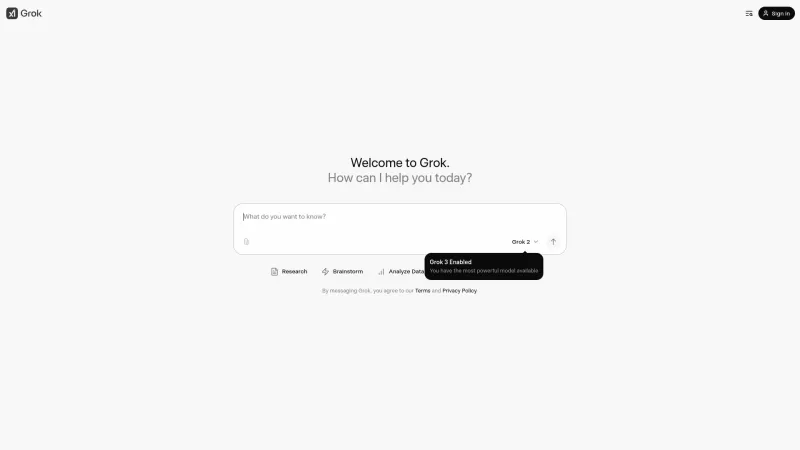
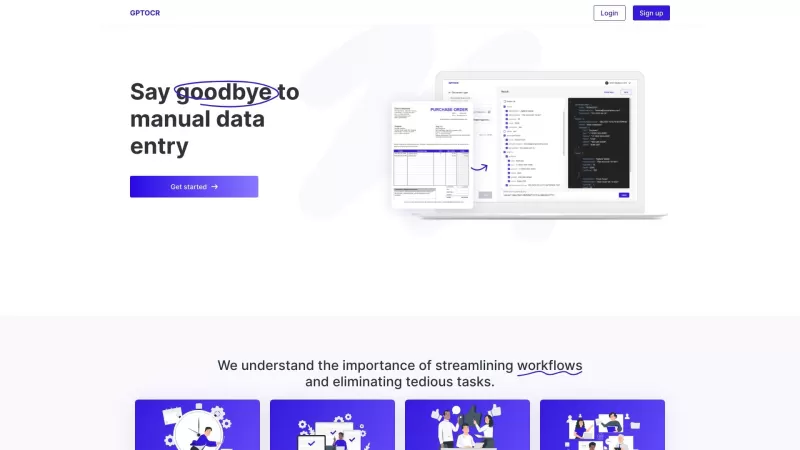
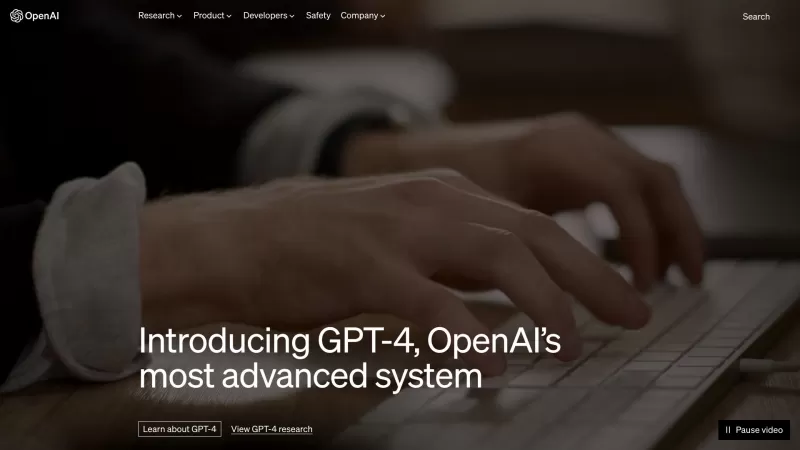
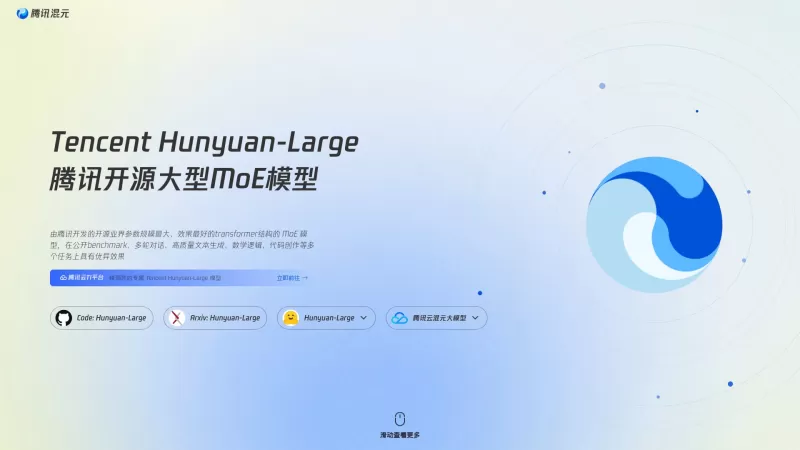
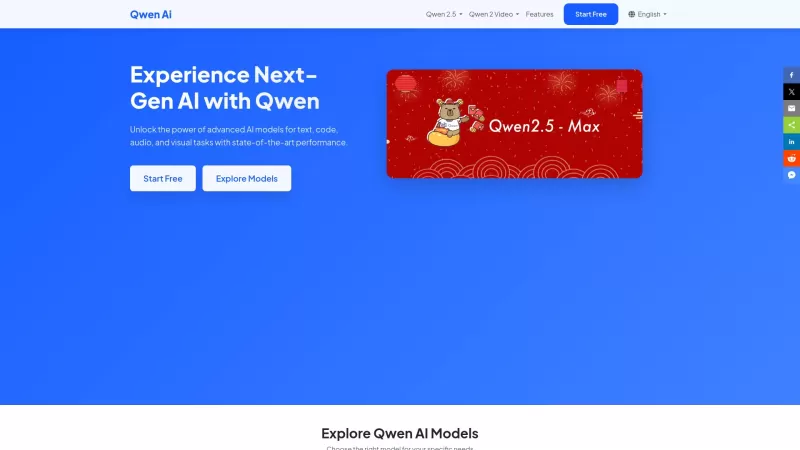
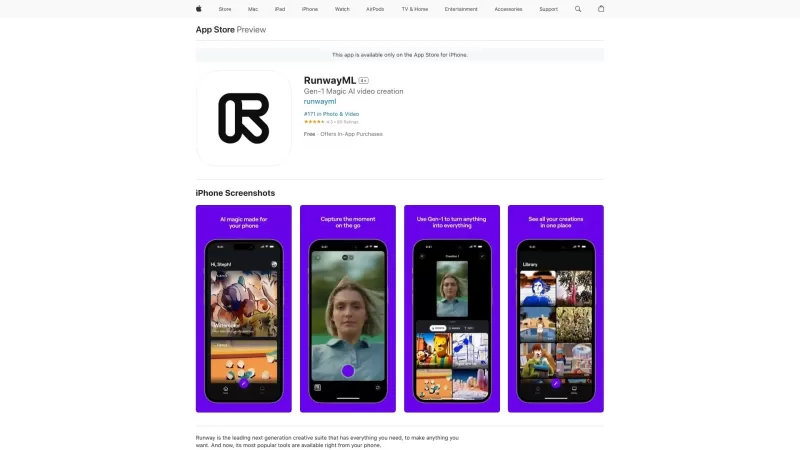