Data Engineering Interview Prep: Ultimate Guide and Cheat Sheet
April 30, 2025
FrankJackson
0
Navigating the World of Data Engineering Interviews
Embarking on the journey of data engineering interviews can feel like stepping into a maze of technologies and concepts. It's easy to feel overwhelmed, but with the right focus, you can channel your energy effectively. This guide serves as your compass, offering a structured approach to preparing for data engineering interviews. From essential technical skills to understanding the project lifecycle, it's designed to empower both seasoned data professionals and newcomers to the field with the knowledge and confidence needed to succeed.
Key Points to Master
- Grasp the core concepts of data engineering, such as ETL, data modeling, and data warehousing.
- Sharpen your technical skills, including SQL, Python, and proficiency with cloud services like AWS, Azure, and GCP.
- Build a portfolio showcasing your data engineering projects to demonstrate your expertise and experience.
- Prepare for typical interview questions, with a focus on problem-solving and system design.
- Understand the full cycle of a data engineering project, from gathering requirements to creating dashboards.
Focus on Data Modeling and Data Design
Becoming proficient in ETL and data pipelines is crucial. These skills form the backbone of effective data management and are highly valued in the field.
Understanding the Fundamentals of Data Engineering
What is Data Engineering?
Data engineering is the unsung hero behind data-driven organizations. It's the art and science of transforming raw data into a usable format for data scientists, business analysts, and stakeholders. Data engineers are the architects who design, build, and maintain the infrastructure that enables organizations to collect, process, store, and analyze massive volumes of data.
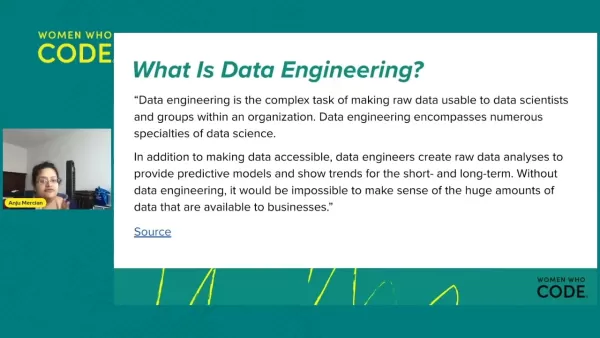
The field encompasses a range of specialties, from data ingestion and extraction to transformation, cleaning, storage, warehousing, pipeline development, automation, and security governance. Data engineers craft robust, scalable, and reliable data systems that fuel informed decision-making. Beyond making data accessible, they also dive into raw data analysis to uncover trends and build predictive models that shape short- and long-term business strategies. Without data engineering, navigating the vast sea of data would be nearly impossible.
The Role of a Data Engineer
Data engineers operate in various environments, constructing systems that collect, manage, and convert raw data into actionable insights for data scientists and business analysts. Their primary mission is to make data accessible and useful for organizations to evaluate and enhance their performance.
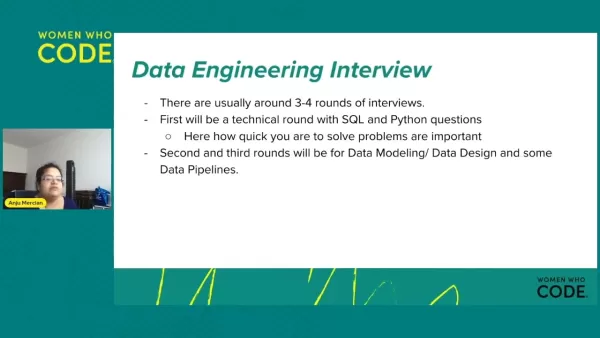
Their key responsibilities include:
- Developing and maintaining data pipelines.
- Building and optimizing data warehouses and data lakes.
- Ensuring data quality and integrity.
- Automating data processing tasks.
- Collaborating with data scientists and business analysts to meet their data needs.
- Implementing data security measures.
- Troubleshooting data-related issues.
Key Technical Skills for Data Engineers
To thrive in data engineering, certain technical skills are non-negotiable:
- SQL: The cornerstone for querying, manipulating, and managing data in relational databases.
- Python: A versatile language used extensively for data processing, automation, and scripting.
- Cloud Computing: Proficiency with platforms like AWS, Azure, or GCP for data storage, processing, and analytics.
- ETL Tools: Familiarity with tools like Apache Airflow for orchestrating data pipelines.
- Spark: A powerful framework for processing large datasets.
Navigating the Data Engineering Project Lifecycle
Data Engineering Project Steps
Understanding the different stages of a data engineering project is vital for success. Here's a typical project lifecycle:
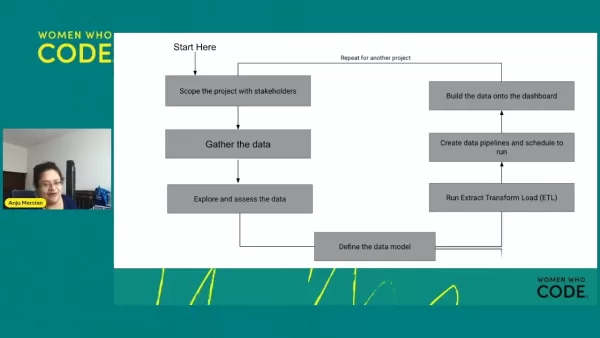
- Scope the Project with Stakeholders: Start by defining project goals and requirements. It's crucial to understand business needs and technical constraints to achieve a successful outcome.
- Gather the Data: Collect data from various sources, both internal and external, such as databases, APIs, or data streams.
- Explore and Assess the Data: Analyze the data to understand its structure, quality, and potential issues, which helps identify what needs to be cleaned and transformed.
- Define the Data Model: Design the data model to structure and store data efficiently. This involves choosing data types, defining relationships, and optimizing for query performance.
- Run Extract Transform Load (ETL): Implement the ETL process to extract data, transform it into a usable format, and load it into the target data warehouse or data lake.
- Create Data Pipelines and Schedule to Run: Develop automated data pipelines to ensure regular data processing and updates, including scheduling tasks, monitoring data flow, and handling errors.
- Build the Data onto the Dashboard: Create dashboards and visualizations to present the processed data in an understandable format, allowing stakeholders to gain insights and make informed decisions. Note that data analysts often handle this stage.
Data Engineering Interview Preparation
Understanding Data Engineering Interview Structure
Data engineering interviews usually follow a structure similar to software engineering interviews, typically consisting of three to four rounds:
- Technical Round(s): These focus on core technical skills like SQL and Python, with coding challenges, problem-solving questions, and discussions about data structures and algorithms.
- Data Modeling and Design: This assesses your ability to design data models, understand data warehousing concepts, and create efficient data pipelines.
- Data Pipelines: This evaluates your skills in designing and maintaining data pipelines.
Prepare for Technical Interviews: SQL and Python
To ace technical interviews, focus on SQL and Python:
SQL:
- Master the Basics: Review SQL syntax, data types, and common query patterns.
- Practice Complex Queries: Work on writing queries to filter, aggregate, and join data from multiple tables, with an eye on query optimization and performance.
- Understand Data Warehousing Concepts: Familiarize yourself with star schemas, snowflake schemas, and other data warehousing concepts.
Python:
- Data Manipulation Libraries: Become proficient with libraries like Pandas and NumPy for data manipulation, cleaning, and analysis.
- Scripting and Automation: Practice writing scripts to automate common data engineering tasks, such as data ingestion, transformation, and loading.
- Data Structures and Algorithms: Refresh your knowledge of fundamental data structures and algorithms to ensure efficient data processing.
Mastering Data Modeling
For data modeling and design, consider the following:
- Dimensional Modeling: Understand the principles of dimensional modeling, including identifying facts, dimensions, and measures.
- Data Normalization: Learn about data normalization techniques to reduce redundancy and improve data integrity.
- Data Warehousing Concepts: Review concepts like star schemas, snowflake schemas, and data vault modeling.
- Real-World Scenarios: Practice designing data models for real-world scenarios, considering factors like data volume, query patterns, and business requirements.
- Understanding Common Data Formats: Learn about structured, semi-structured, and unstructured data formats, including CSV, JSON, XML, and others.
Interview Tips: Common Question Types
Be ready for various types of questions in data engineering interviews:
- Behavioral Questions: Prepare to discuss your experience, problem-solving skills, and teamwork abilities. Use the STAR method (Situation, Task, Action, Result) to structure your responses.
- Technical Questions: Expect questions on coding, SQL, and data pipeline designs.
- Data Cleaning and Transformation: Be ready to discuss techniques for handling missing data, outliers, and inconsistencies.
- Data Pipeline Design: Prepare to design end-to-end data pipelines, considering factors like data volume, latency, and error handling.
For real-world data modeling, be prepared to discuss your experience with cloud infrastructure and other proprietary information.
Benefits and Challenges in Data Engineering
Pros
- High Demand and Competitive Salaries: Data engineers are in high demand, which translates to competitive salaries.
- Intellectually Stimulating Work: The role involves tackling complex technical challenges and working with cutting-edge technologies.
- Impactful Contributions: Data engineers play a crucial role in enabling businesses to make data-driven decisions and gain a competitive edge.
- Career Growth Opportunities: The field offers numerous career paths, including specialized roles in data warehousing, data pipelines, and data governance.
Cons
- Technical Complexity: The job requires a deep understanding of various technologies and concepts.
- Constant Learning: The landscape of data engineering is ever-evolving, necessitating continuous learning and skill development.
- High Pressure: Data engineers are responsible for the reliability and performance of critical data systems.
- Collaboration Challenges: The role often involves working with diverse teams, requiring strong communication and collaboration skills.
Frequently Asked Questions
What is the difference between data engineering and data science?
Data engineers focus on building and maintaining the infrastructure that manages data, while data scientists analyze that data to extract insights and build predictive models. In essence, data engineers prepare the data, and data scientists use it.
Is a computer science degree necessary to become a data engineer?
While a computer science degree can be helpful, it's not always required. What's essential is a strong grasp of programming, databases, and data structures, which can be acquired through various educational paths.
What are some popular data engineering tools?
Popular tools in data engineering include Apache Airflow, Apache Spark, Hadoop, Kafka, and cloud-based services like AWS, Azure, and GCP.
How can I build a data engineering portfolio?
Building a portfolio can be achieved by working on personal data projects, contributing to open-source projects, and participating in data engineering competitions.
Related Questions
What are the top skills needed for a data engineer in 2025?
In 2025, data engineers will need a blend of technical prowess, business acumen, and soft skills to excel. Here's a detailed look:
Technical Proficiency:
- Cloud Computing: Expertise in AWS, Azure, or GCP is crucial. Familiarity with services like AWS S3 for storage and Redshift for data warehousing, or Azure Synapse Analytics, Data Lake Storage, and Data Factory, is highly valued.
- SQL and NoSQL Databases: Proficiency in SQL is fundamental, while experience with NoSQL databases like MongoDB or Cassandra is increasingly important for handling unstructured data.
- Programming Languages: Python is the go-to language, followed by Java and Scala, essential for data processing, automation, and scripting.
- Big Data Technologies: Mastery of technologies like Apache Spark and Hadoop for processing and analyzing large datasets is essential.
- Data Pipeline Tools: Proficiency in tools like Apache Airflow, Jenkins, and Kubeflow for orchestrating and automating data workflows is crucial.
- Data Modeling: A strong understanding of data modeling techniques, including dimensional modeling and data normalization, is vital for designing efficient data warehouses.
- ETL/ELT Processes: Knowledge of ETL and ELT processes is essential for data integration and transformation.
Business Acumen:
- Data Governance: Understanding data governance principles, including data quality, security, and compliance, is crucial for ensuring data integrity.
- Data Literacy: The ability to understand, interpret, and communicate data insights effectively is becoming increasingly important.
- Business Intelligence (BI) Tools: Familiarity with BI tools like Tableau or Power BI can be beneficial for building dashboards and visualizing data.
Soft Skills:
- Communication: Effective communication is key for collaborating with stakeholders and conveying technical information.
- Problem-Solving: The ability to quickly identify and solve data-related issues is essential for maintaining system reliability.
- Teamwork: Working in cross-functional teams requires strong teamwork and collaboration skills.
- Adaptability: The ever-changing nature of data engineering demands adaptability and a willingness to learn new technologies.
Related article
Pixar Unveils AI Animal Character Creation Style Guide
Artificial intelligence is revolutionizing the creative world, making it possible for anyone to conjure up breathtaking visuals with just a few words. This includes crafting animal characters in the iconic Pixar style, known for its charm and emotional depth. This guide will walk you through using A
Virbo Tutorial: Mastering AI Video Creation for High-Quality Outputs
In today's digital world, video content is king. But let's face it, making engaging videos can be a real time and money sink. What if you could harness the power of artificial intelligence to whip up professional-quality videos quickly and efficiently? Enter Virbo, an AI-powered platform that promis
Huawei's AI Hardware Breakthrough Poses Challenge to Nvidia's Dominance
Huawei's Bold Move in the Global AI Chip Race
Huawei, the Chinese tech giant, has taken a significant step forward that could shake up the global AI chip race. They've introduced a new computing system called the CloudMatrix 384 Supernode, which, according to local media, outperforms similar techno
Comments (0)
0/200






Navigating the World of Data Engineering Interviews
Embarking on the journey of data engineering interviews can feel like stepping into a maze of technologies and concepts. It's easy to feel overwhelmed, but with the right focus, you can channel your energy effectively. This guide serves as your compass, offering a structured approach to preparing for data engineering interviews. From essential technical skills to understanding the project lifecycle, it's designed to empower both seasoned data professionals and newcomers to the field with the knowledge and confidence needed to succeed.
Key Points to Master
- Grasp the core concepts of data engineering, such as ETL, data modeling, and data warehousing.
- Sharpen your technical skills, including SQL, Python, and proficiency with cloud services like AWS, Azure, and GCP.
- Build a portfolio showcasing your data engineering projects to demonstrate your expertise and experience.
- Prepare for typical interview questions, with a focus on problem-solving and system design.
- Understand the full cycle of a data engineering project, from gathering requirements to creating dashboards.
Focus on Data Modeling and Data Design
Becoming proficient in ETL and data pipelines is crucial. These skills form the backbone of effective data management and are highly valued in the field.
Understanding the Fundamentals of Data Engineering
What is Data Engineering?
Data engineering is the unsung hero behind data-driven organizations. It's the art and science of transforming raw data into a usable format for data scientists, business analysts, and stakeholders. Data engineers are the architects who design, build, and maintain the infrastructure that enables organizations to collect, process, store, and analyze massive volumes of data.
The field encompasses a range of specialties, from data ingestion and extraction to transformation, cleaning, storage, warehousing, pipeline development, automation, and security governance. Data engineers craft robust, scalable, and reliable data systems that fuel informed decision-making. Beyond making data accessible, they also dive into raw data analysis to uncover trends and build predictive models that shape short- and long-term business strategies. Without data engineering, navigating the vast sea of data would be nearly impossible.
The Role of a Data Engineer
Data engineers operate in various environments, constructing systems that collect, manage, and convert raw data into actionable insights for data scientists and business analysts. Their primary mission is to make data accessible and useful for organizations to evaluate and enhance their performance.
Their key responsibilities include:
- Developing and maintaining data pipelines.
- Building and optimizing data warehouses and data lakes.
- Ensuring data quality and integrity.
- Automating data processing tasks.
- Collaborating with data scientists and business analysts to meet their data needs.
- Implementing data security measures.
- Troubleshooting data-related issues.
Key Technical Skills for Data Engineers
To thrive in data engineering, certain technical skills are non-negotiable:
- SQL: The cornerstone for querying, manipulating, and managing data in relational databases.
- Python: A versatile language used extensively for data processing, automation, and scripting.
- Cloud Computing: Proficiency with platforms like AWS, Azure, or GCP for data storage, processing, and analytics.
- ETL Tools: Familiarity with tools like Apache Airflow for orchestrating data pipelines.
- Spark: A powerful framework for processing large datasets.
Navigating the Data Engineering Project Lifecycle
Data Engineering Project Steps
Understanding the different stages of a data engineering project is vital for success. Here's a typical project lifecycle:
- Scope the Project with Stakeholders: Start by defining project goals and requirements. It's crucial to understand business needs and technical constraints to achieve a successful outcome.
- Gather the Data: Collect data from various sources, both internal and external, such as databases, APIs, or data streams.
- Explore and Assess the Data: Analyze the data to understand its structure, quality, and potential issues, which helps identify what needs to be cleaned and transformed.
- Define the Data Model: Design the data model to structure and store data efficiently. This involves choosing data types, defining relationships, and optimizing for query performance.
- Run Extract Transform Load (ETL): Implement the ETL process to extract data, transform it into a usable format, and load it into the target data warehouse or data lake.
- Create Data Pipelines and Schedule to Run: Develop automated data pipelines to ensure regular data processing and updates, including scheduling tasks, monitoring data flow, and handling errors.
- Build the Data onto the Dashboard: Create dashboards and visualizations to present the processed data in an understandable format, allowing stakeholders to gain insights and make informed decisions. Note that data analysts often handle this stage.
Data Engineering Interview Preparation
Understanding Data Engineering Interview Structure
Data engineering interviews usually follow a structure similar to software engineering interviews, typically consisting of three to four rounds:
- Technical Round(s): These focus on core technical skills like SQL and Python, with coding challenges, problem-solving questions, and discussions about data structures and algorithms.
- Data Modeling and Design: This assesses your ability to design data models, understand data warehousing concepts, and create efficient data pipelines.
- Data Pipelines: This evaluates your skills in designing and maintaining data pipelines.
Prepare for Technical Interviews: SQL and Python
To ace technical interviews, focus on SQL and Python:
SQL:
- Master the Basics: Review SQL syntax, data types, and common query patterns.
- Practice Complex Queries: Work on writing queries to filter, aggregate, and join data from multiple tables, with an eye on query optimization and performance.
- Understand Data Warehousing Concepts: Familiarize yourself with star schemas, snowflake schemas, and other data warehousing concepts.
Python:
- Data Manipulation Libraries: Become proficient with libraries like Pandas and NumPy for data manipulation, cleaning, and analysis.
- Scripting and Automation: Practice writing scripts to automate common data engineering tasks, such as data ingestion, transformation, and loading.
- Data Structures and Algorithms: Refresh your knowledge of fundamental data structures and algorithms to ensure efficient data processing.
Mastering Data Modeling
For data modeling and design, consider the following:
- Dimensional Modeling: Understand the principles of dimensional modeling, including identifying facts, dimensions, and measures.
- Data Normalization: Learn about data normalization techniques to reduce redundancy and improve data integrity.
- Data Warehousing Concepts: Review concepts like star schemas, snowflake schemas, and data vault modeling.
- Real-World Scenarios: Practice designing data models for real-world scenarios, considering factors like data volume, query patterns, and business requirements.
- Understanding Common Data Formats: Learn about structured, semi-structured, and unstructured data formats, including CSV, JSON, XML, and others.
Interview Tips: Common Question Types
Be ready for various types of questions in data engineering interviews:
- Behavioral Questions: Prepare to discuss your experience, problem-solving skills, and teamwork abilities. Use the STAR method (Situation, Task, Action, Result) to structure your responses.
- Technical Questions: Expect questions on coding, SQL, and data pipeline designs.
- Data Cleaning and Transformation: Be ready to discuss techniques for handling missing data, outliers, and inconsistencies.
- Data Pipeline Design: Prepare to design end-to-end data pipelines, considering factors like data volume, latency, and error handling.
For real-world data modeling, be prepared to discuss your experience with cloud infrastructure and other proprietary information.
Benefits and Challenges in Data Engineering
Pros
- High Demand and Competitive Salaries: Data engineers are in high demand, which translates to competitive salaries.
- Intellectually Stimulating Work: The role involves tackling complex technical challenges and working with cutting-edge technologies.
- Impactful Contributions: Data engineers play a crucial role in enabling businesses to make data-driven decisions and gain a competitive edge.
- Career Growth Opportunities: The field offers numerous career paths, including specialized roles in data warehousing, data pipelines, and data governance.
Cons
- Technical Complexity: The job requires a deep understanding of various technologies and concepts.
- Constant Learning: The landscape of data engineering is ever-evolving, necessitating continuous learning and skill development.
- High Pressure: Data engineers are responsible for the reliability and performance of critical data systems.
- Collaboration Challenges: The role often involves working with diverse teams, requiring strong communication and collaboration skills.
Frequently Asked Questions
What is the difference between data engineering and data science?
Data engineers focus on building and maintaining the infrastructure that manages data, while data scientists analyze that data to extract insights and build predictive models. In essence, data engineers prepare the data, and data scientists use it.
Is a computer science degree necessary to become a data engineer?
While a computer science degree can be helpful, it's not always required. What's essential is a strong grasp of programming, databases, and data structures, which can be acquired through various educational paths.
What are some popular data engineering tools?
Popular tools in data engineering include Apache Airflow, Apache Spark, Hadoop, Kafka, and cloud-based services like AWS, Azure, and GCP.
How can I build a data engineering portfolio?
Building a portfolio can be achieved by working on personal data projects, contributing to open-source projects, and participating in data engineering competitions.
Related Questions
What are the top skills needed for a data engineer in 2025?
In 2025, data engineers will need a blend of technical prowess, business acumen, and soft skills to excel. Here's a detailed look:
Technical Proficiency:
- Cloud Computing: Expertise in AWS, Azure, or GCP is crucial. Familiarity with services like AWS S3 for storage and Redshift for data warehousing, or Azure Synapse Analytics, Data Lake Storage, and Data Factory, is highly valued.
- SQL and NoSQL Databases: Proficiency in SQL is fundamental, while experience with NoSQL databases like MongoDB or Cassandra is increasingly important for handling unstructured data.
- Programming Languages: Python is the go-to language, followed by Java and Scala, essential for data processing, automation, and scripting.
- Big Data Technologies: Mastery of technologies like Apache Spark and Hadoop for processing and analyzing large datasets is essential.
- Data Pipeline Tools: Proficiency in tools like Apache Airflow, Jenkins, and Kubeflow for orchestrating and automating data workflows is crucial.
- Data Modeling: A strong understanding of data modeling techniques, including dimensional modeling and data normalization, is vital for designing efficient data warehouses.
- ETL/ELT Processes: Knowledge of ETL and ELT processes is essential for data integration and transformation.
Business Acumen:
- Data Governance: Understanding data governance principles, including data quality, security, and compliance, is crucial for ensuring data integrity.
- Data Literacy: The ability to understand, interpret, and communicate data insights effectively is becoming increasingly important.
- Business Intelligence (BI) Tools: Familiarity with BI tools like Tableau or Power BI can be beneficial for building dashboards and visualizing data.
Soft Skills:
- Communication: Effective communication is key for collaborating with stakeholders and conveying technical information.
- Problem-Solving: The ability to quickly identify and solve data-related issues is essential for maintaining system reliability.
- Teamwork: Working in cross-functional teams requires strong teamwork and collaboration skills.
- Adaptability: The ever-changing nature of data engineering demands adaptability and a willingness to learn new technologies.
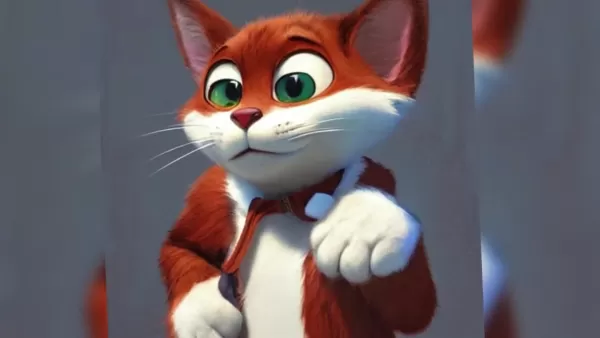
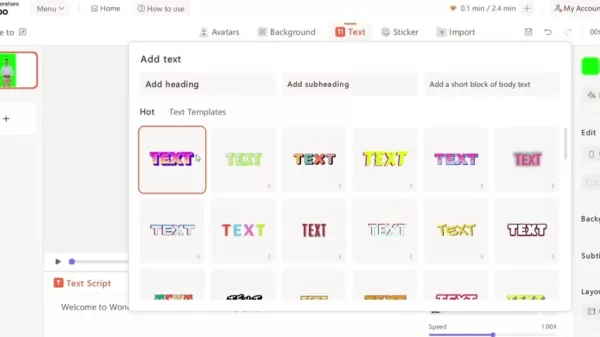
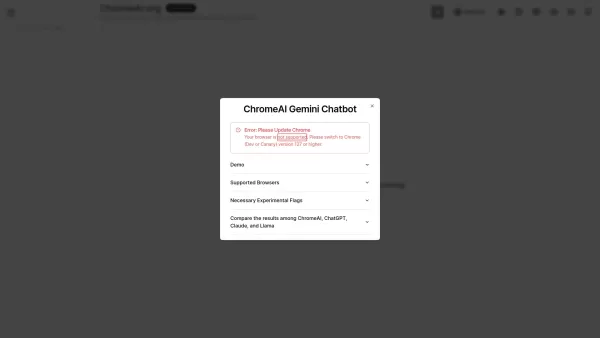
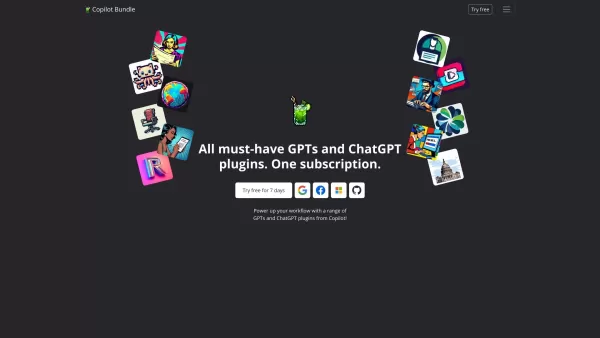
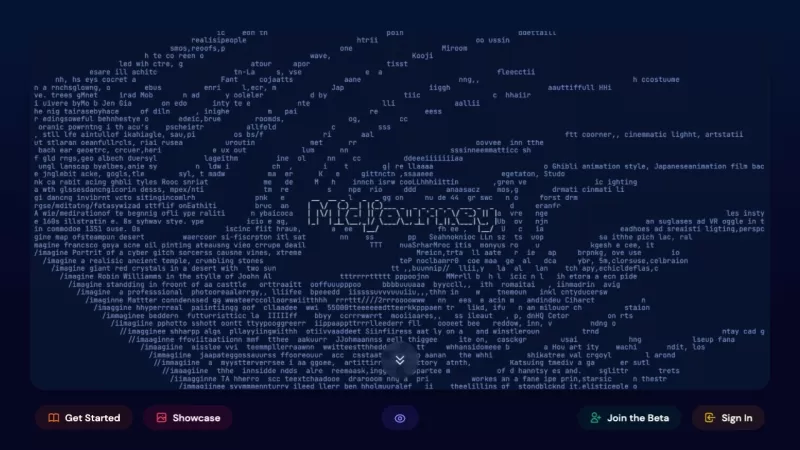
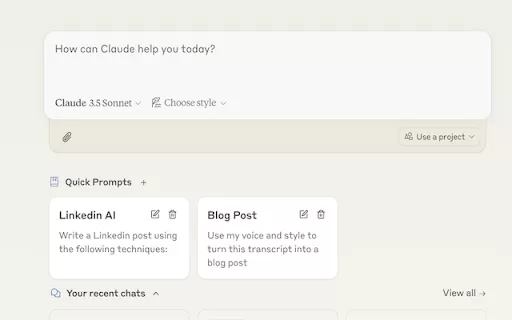
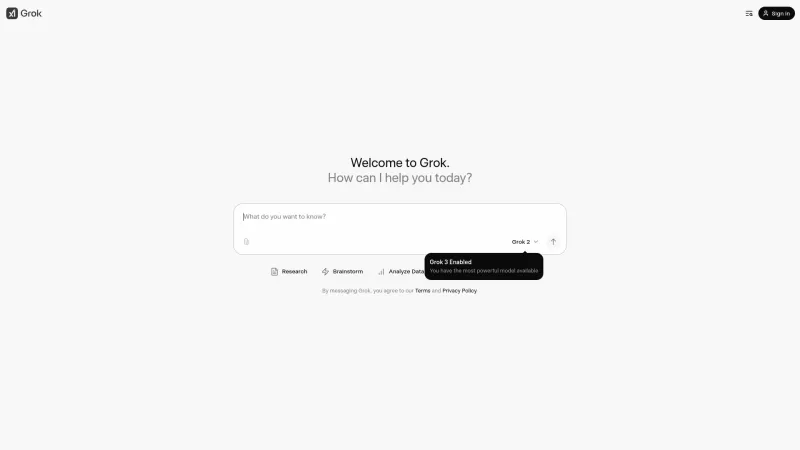
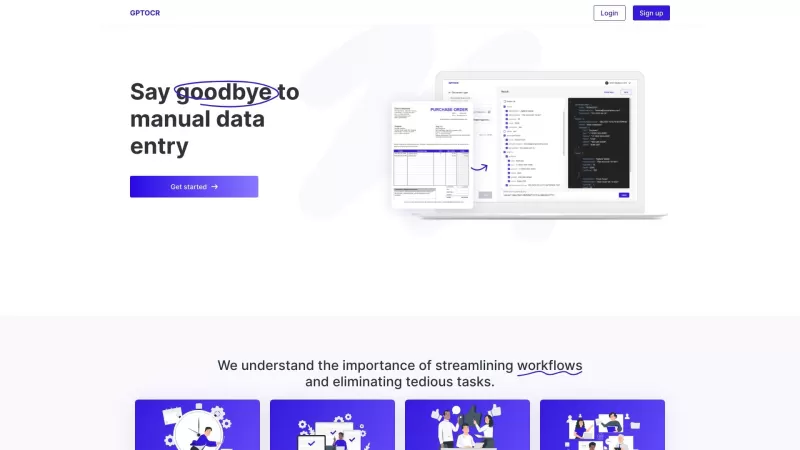
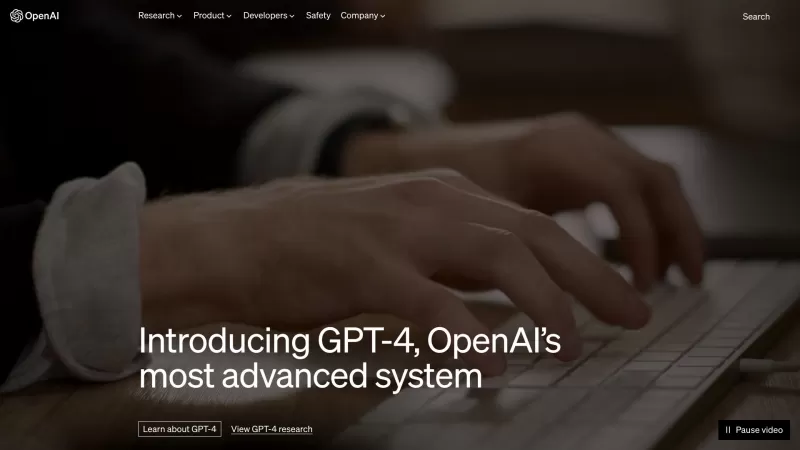
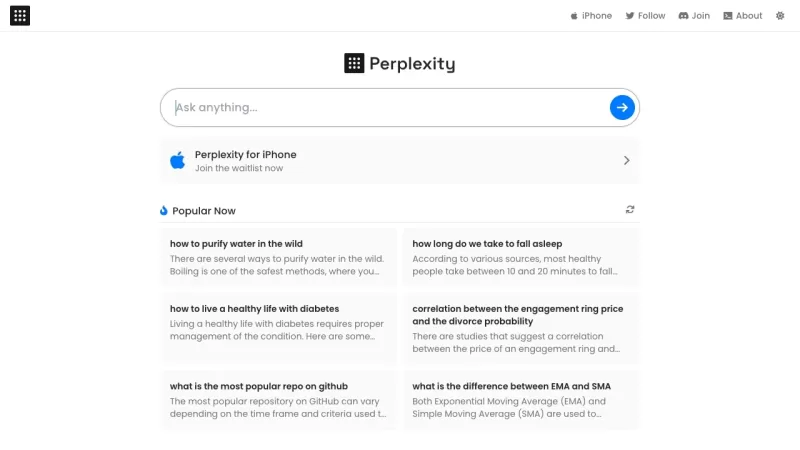
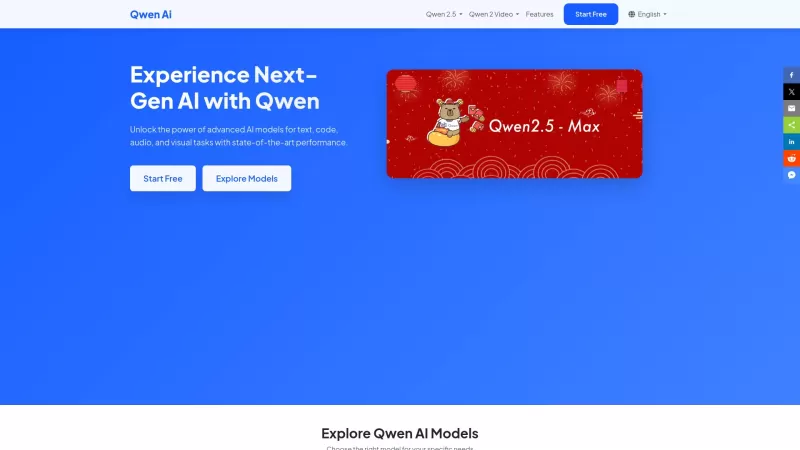
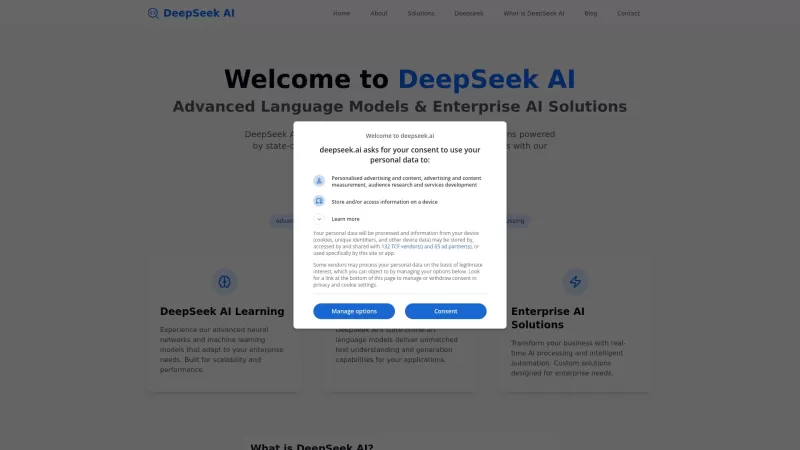