AI-Driven Development of New Open Buildings Dataset Unveiled
April 10, 2025
HarryGonzález
38
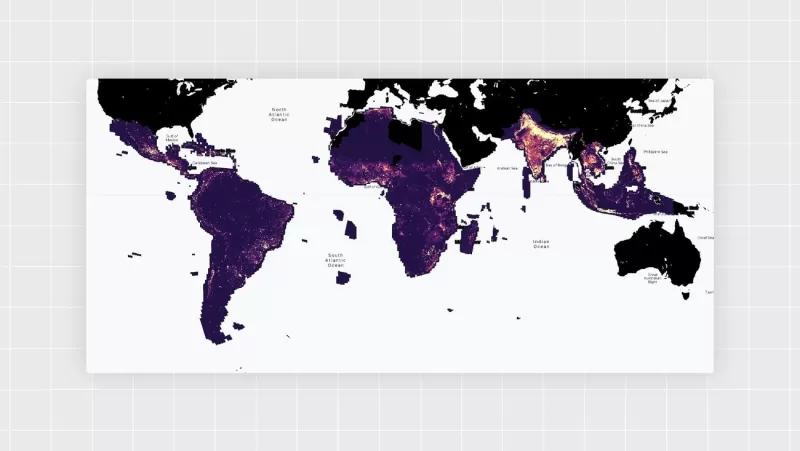
In 2021, the Google Research Africa team kicked off Open Buildings, an open-source dataset that maps building footprints across the Global South using AI and high-resolution satellite imagery. Their goal was pretty straightforward: to plug a big hole in the data about population and density in developing countries. Now, with the third version out, their dataset boasts polygons for a whopping 1.8 billion buildings spread over 58 million km² in Africa, South and Southeast Asia, Latin America, and the Caribbean.
A bunch of folks, from governments to the UN, and even researchers and nonprofits, have been using Open Buildings to get a handle on population size and distribution. This has helped them plan better for things like vaccination drives and disaster response. Plus, it's even beefed up Google Maps by adding millions of buildings that weren't mapped before.
The team, based in Ghana but with members scattered across places like Tel Aviv and Zurich, has been on a mission to make the project even more useful. "We're always in hackathon mode, trying out new ideas and tackling challenges," says Google Research program manager Abdoulaye Diack. "One thing we couldn't do with the original dataset was show how areas change over time—it was static. And that's something our partners really wanted."
Commercial satellite image providers usually focus on areas that bring in the bucks, leaving about 40% of the world, mostly the Global South, without regular high-res coverage. Some remote spots and informal settlements don't get any coverage at all. Meanwhile, open-source imagery from the European Space Agency's Sentinel-2 satellite, which snaps a global pic every five days, was thought to be too low-res for building detection.
But the team figured it might not be as big a deal as they thought, so they gave it a shot.
First, they fed a single low-res frame from Sentinel-2 into their model and asked it to draw building polygons. "It was tough, but we saw potential," Abdoulaye says. "So we told the model to just give us the building masks—binary pixel data tied to specific spots. It did okay, and we thought, 'Hey, we can do this.'"
After a year of tweaking the model, they rolled out the Open Buildings 2.5D Temporal Dataset last month. It covers 2016 to 2023 and gives an annual snapshot of building presence and counts across much of the Global South, plus building heights. This shows how cities change due to development, disasters, and other factors. Users can pick a region, flip through the years, and watch the world grow and shrink in a colorful display of shapes.
"By 2050, about 2.5 billion more people might move to cities, mostly in the Global South. This dataset could be a game-changer for governments and organizations dealing with that growth," says Google Research product manager Olivia Graham. "If a city's planning where to put essential services like healthcare and education, or where to build infrastructure like water and energy supplies, this dataset shows which areas are growing fast."
On September 28, 2018, a massive 7.4 magnitude earthquake off Indonesia's coast triggered a tsunami, impacting around 1.5 million people on Sulawesi. The dataset shows how the built area pulled back from the coast after the disaster. You can check it out in our interactive Earth Engine app.
You can also see the construction of New Cairo, Egypt, in the Open Buildings 2.5D Temporal Dataset demo.
So, how did the team manage to get their model to read Sentinel-2's fuzzy satellite images and confidently detect buildings? They started by sharpening things up.
"We used a teacher-student model setup to both 'super-resolve' the low-res images and pull out the building footprints," says Google Research software engineer Krishna Sapkota. "The teacher model learns to spot buildings in high-res images and gives labels to the student model. The student model, which actually creates the dataset, learns from the teacher's output. It can then take low-res images from Sentinel-2 and guess what a higher-res version would look like."
The teacher model gives high-res training labels to the student model, which then figures out building presence from low-res imagery.
To get the detail needed for building footprints, the model uses up to 32 frames of Sentinel-2 images of a spot for any prediction. Each frame is a bit different from the others, thanks to the tiny time gap between captures, which helps boost resolution—kind of like how Pixel phones use multiple shots for sharper images.
Unlike the original dataset, which gave precise polygonal outlines of buildings, the new temporal dataset uses raster data for building footprints. It also predicts building heights, crucial for estimating population density, with an error of just 1.5 meters, or less than one story.
Before its official release, the temporal dataset was shared with trusted partners like Ugandan nonprofit Sunbird AI. "About 73% of Ugandans don't have electricity, and Sunbird AI used our original database to help the government figure out where to put microgrids or solar panels," Olivia says. "With the new dataset, they're looking at Jinja and Fort Portal, creating visuals that help city councils see where growth is happening fast and adjust their plans. It shows how both datasets can be part of a bigger toolkit to understand a population and how it's changing."
The same curiosity that led to the temporal dataset is pushing the team to keep improving it.
"I live in Ghana and see the impact our work is having and can have," Abdoulaye says. "Many places here struggle with resources, which leads to data gaps with big consequences. Being part of a team working to fix that and make a difference is a real honor."
Related article
Debates over AI benchmarking have reached Pokémon
Even the beloved world of Pokémon isn't immune to the drama surrounding AI benchmarks. A recent viral post on X stirred up quite the buzz, claiming that Google's latest Gemini model had outpaced Anthropic's leading Claude model in the classic Pokémon video game trilogy. According to the post, Gemini
Top 10 AI Marketing Tools for April 2025
Artificial intelligence (AI) is shaking up industries left and right, and marketing is no exception. From small startups to big corporations, businesses are increasingly turning to AI marketing tools to boost their brand visibility and drive their growth. Incorporating these tools into your business
Wikipedia is giving AI developers its data to fend off bot scrapers
Wikipedia's New Strategy to Manage AI Data Scraping
Wikipedia, through the Wikimedia Foundation, is taking a proactive step to manage the impact of AI data scraping on its servers. On Wednesday, they announced a collaboration with Kaggle, a platform owned by Google and dedicated to data science and
Comments (25)
0/200
StevenSanchez
April 14, 2025 at 12:10:04 AM GMT
This dataset is a game-changer for urban planning in the Global South! It's amazing how AI can help map building footprints so accurately. Only downside is it's a bit tricky to navigate the dataset if you're not tech-savvy. Still, a must-have for researchers!
0
DonaldGonzález
April 13, 2025 at 9:52:40 PM GMT
グローバルサウスの都市計画に革命をもたらすデータセットです!AIが建物のフットプリントをこれほど正確にマッピングできるなんて驚きです。唯一の欠点は、技術に詳しくないとデータセットの操作が少し難しいことです。それでも、研究者にとって必須のツールです!
0
WillLopez
April 11, 2025 at 10:31:22 PM GMT
글로벌 사우스의 도시 계획에 혁신을 가져오는 데이터셋입니다! AI가 건물의 발자취를 이렇게 정확하게 매핑할 수 있다는 것이 놀랍습니다. 단점은 기술에 익숙하지 않으면 데이터셋을 다루기가 조금 어렵다는 점입니다. 그래도 연구자에게는 필수 도구입니다!
0
JustinJackson
April 13, 2025 at 11:49:41 AM GMT
Este conjunto de dados é um divisor de águas para o planejamento urbano no Sul Global! É incrível como a IA pode mapear as pegadas dos edifícios com tanta precisão. A única desvantagem é que pode ser um pouco complicado navegar pelo conjunto de dados se você não for muito técnico. Ainda assim, uma ferramenta essencial para pesquisadores!
0
RaymondRodriguez
April 12, 2025 at 12:51:42 AM GMT
¡Este conjunto de datos es un cambio de juego para la planificación urbana en el Sur Global! Es increíble cómo la IA puede mapear las huellas de los edificios con tanta precisión. La única desventaja es que puede ser un poco complicado navegar por el conjunto de datos si no eres muy técnico. Aún así, una herramienta imprescindible para los investigadores!
0
AnthonyMartinez
April 11, 2025 at 7:26:38 PM GMT
The Open Buildings dataset is a game-changer for urban planning in the Global South! It's amazing how AI and satellite imagery can map out building footprints so accurately. My only gripe is that the data updates aren't as frequent as I'd like. Still, it's a fantastic resource! 🌍
0






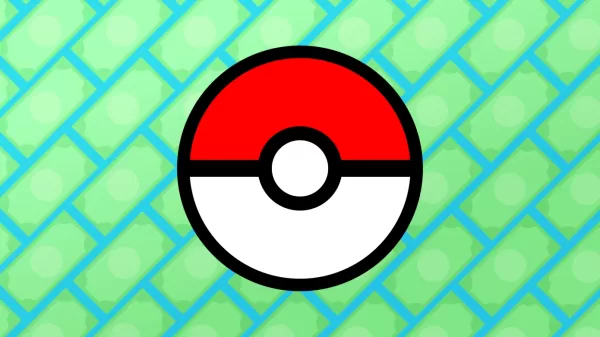
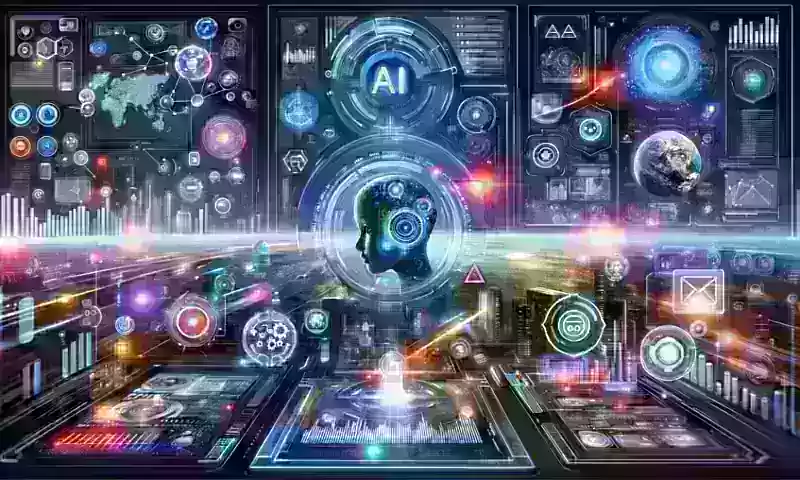
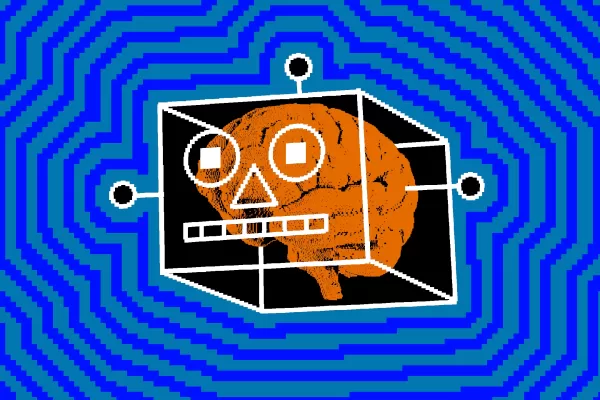

This dataset is a game-changer for urban planning in the Global South! It's amazing how AI can help map building footprints so accurately. Only downside is it's a bit tricky to navigate the dataset if you're not tech-savvy. Still, a must-have for researchers!




グローバルサウスの都市計画に革命をもたらすデータセットです!AIが建物のフットプリントをこれほど正確にマッピングできるなんて驚きです。唯一の欠点は、技術に詳しくないとデータセットの操作が少し難しいことです。それでも、研究者にとって必須のツールです!




글로벌 사우스의 도시 계획에 혁신을 가져오는 데이터셋입니다! AI가 건물의 발자취를 이렇게 정확하게 매핑할 수 있다는 것이 놀랍습니다. 단점은 기술에 익숙하지 않으면 데이터셋을 다루기가 조금 어렵다는 점입니다. 그래도 연구자에게는 필수 도구입니다!




Este conjunto de dados é um divisor de águas para o planejamento urbano no Sul Global! É incrível como a IA pode mapear as pegadas dos edifícios com tanta precisão. A única desvantagem é que pode ser um pouco complicado navegar pelo conjunto de dados se você não for muito técnico. Ainda assim, uma ferramenta essencial para pesquisadores!




¡Este conjunto de datos es un cambio de juego para la planificación urbana en el Sur Global! Es increíble cómo la IA puede mapear las huellas de los edificios con tanta precisión. La única desventaja es que puede ser un poco complicado navegar por el conjunto de datos si no eres muy técnico. Aún así, una herramienta imprescindible para los investigadores!




The Open Buildings dataset is a game-changer for urban planning in the Global South! It's amazing how AI and satellite imagery can map out building footprints so accurately. My only gripe is that the data updates aren't as frequent as I'd like. Still, it's a fantastic resource! 🌍



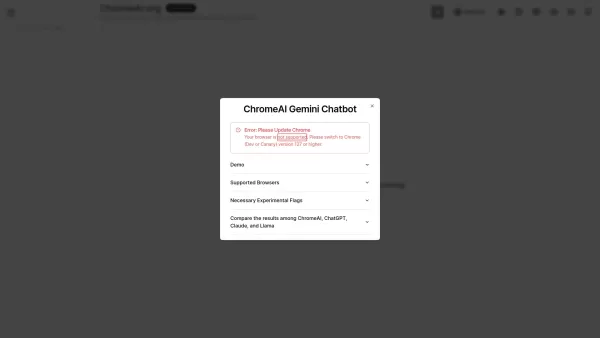
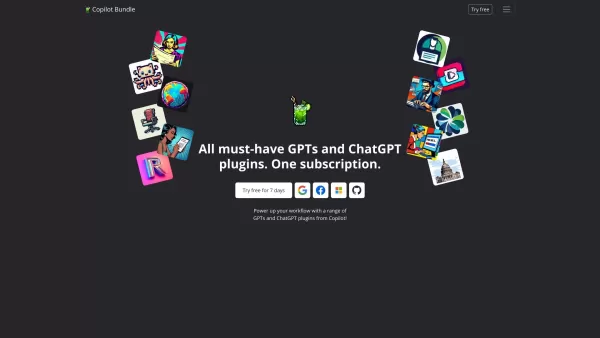
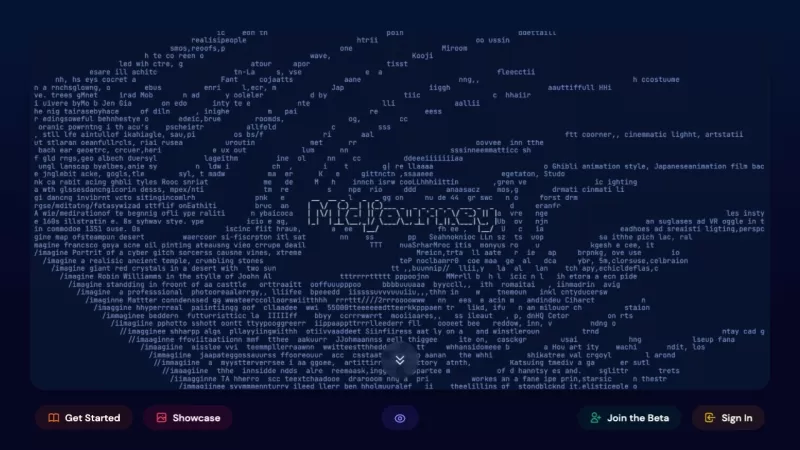
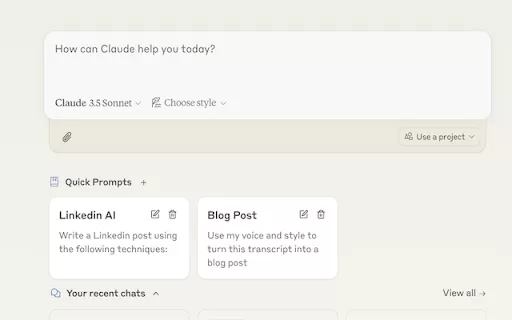
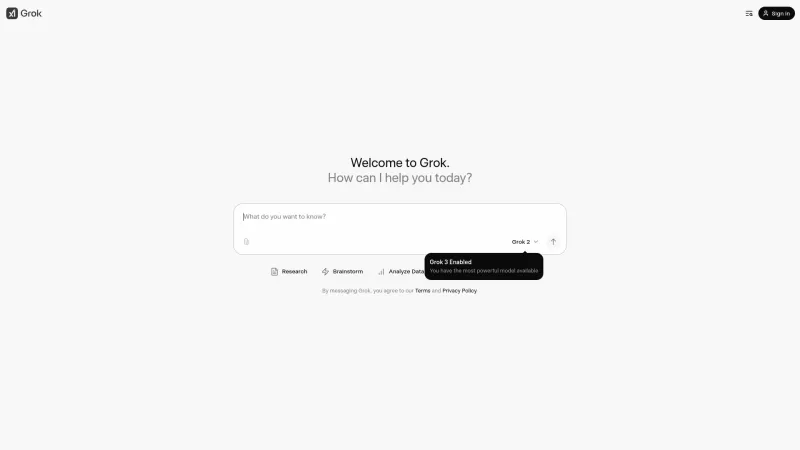
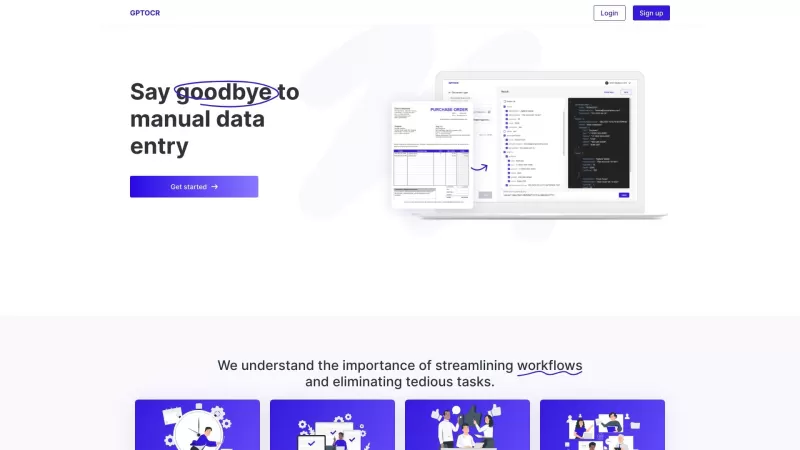
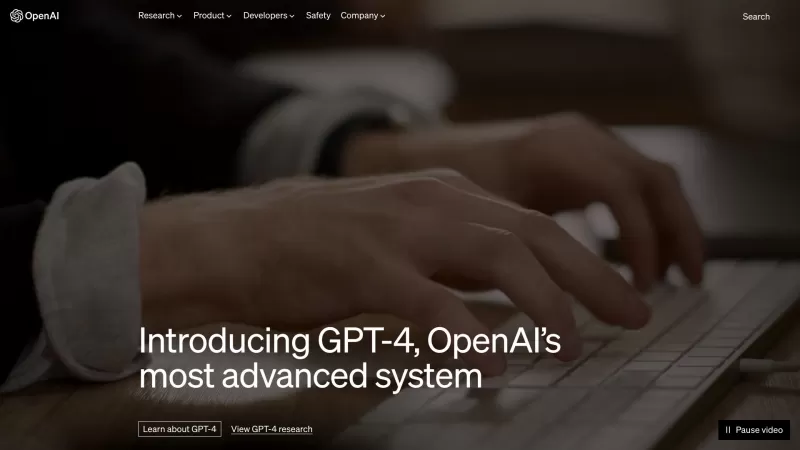
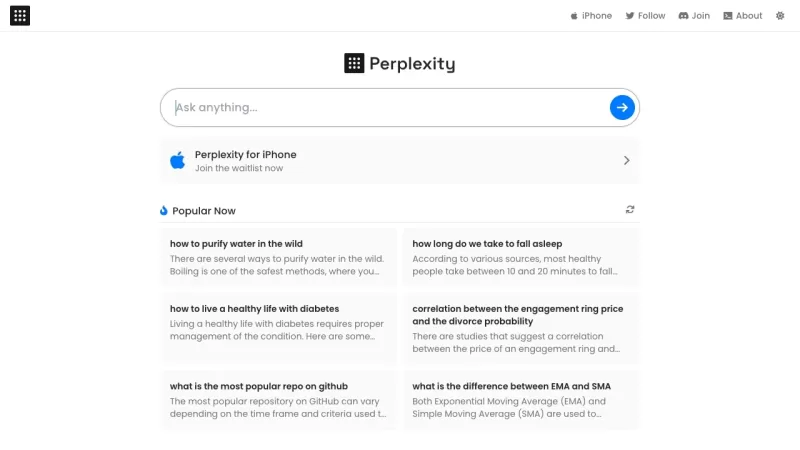
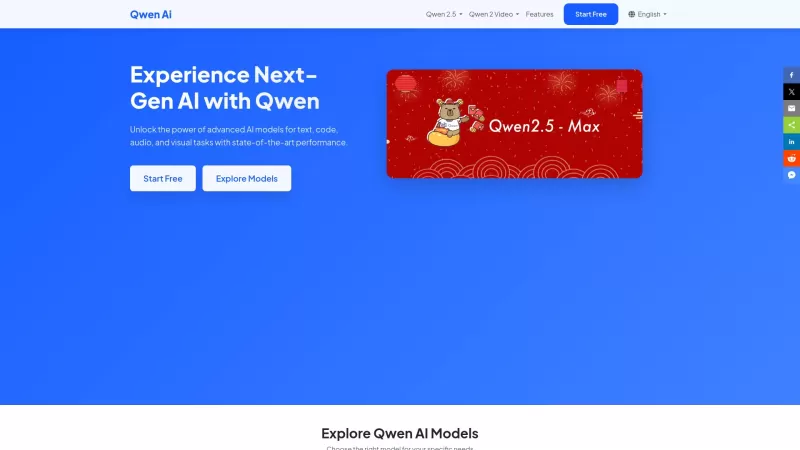
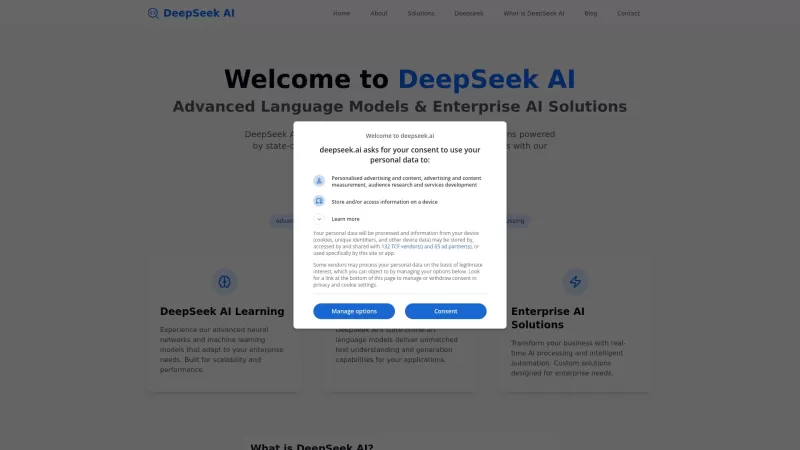