Researchers Develop Open-Source Rival to OpenAI's $50 'Reasoning' Model for Under $50
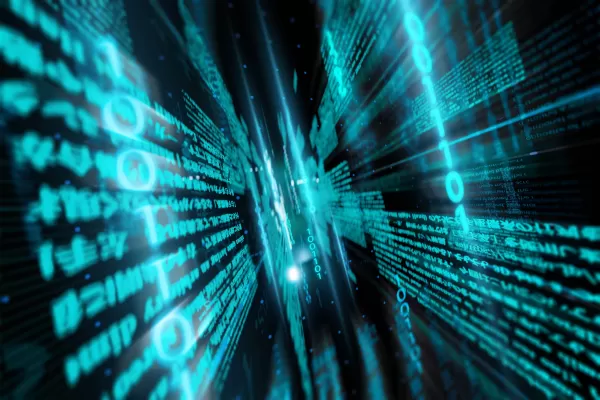
Last Friday, a groundbreaking research paper from AI experts at Stanford and the University of Washington hit the scene, revealing that they managed to develop an AI "reasoning" model, dubbed s1, for under $50 in cloud compute credits. This revelation is shaking up the AI world, as s1 holds its own against top-tier models like OpenAI's o1 and DeepSeek's R1 when it comes to tackling math and coding challenges.
The s1 model, along with all the juicy details of its training data and code, is now up for grabs on GitHub. The team kicked things off with a run-of-the-mill base model and then put it through the wringer with a technique called distillation. This process involves squeezing out the "reasoning" juice from another AI model by training on its responses. In this case, s1 got its smarts from Google's Gemini 2.0 Flash Thinking Experimental model. It's a similar tactic to what Berkeley researchers used to whip up their own AI reasoning model for around $450 just last month.
For some, the idea that a small team of researchers can still make waves in the AI field without a massive budget is thrilling. But s1's emergence also sparks some serious questions about the future of AI model development. If a model that rivals those built with millions can be replicated on a shoestring budget, what's to stop everyone from doing the same?
Not surprisingly, the big players in AI aren't thrilled. OpenAI, for instance, has pointed fingers at DeepSeek, accusing them of using their API data to fuel model distillation. Meanwhile, the s1 team was focused on finding the most straightforward way to achieve solid reasoning performance and something called "test-time scaling," where an AI model gets more time to think before answering. These are the same innovations that OpenAI's o1 model brought to the table, which others like DeepSeek have tried to mimic with their own methods.
The s1 paper suggests that you can distill reasoning models with a relatively small dataset using a technique known as supervised fine-tuning (SFT). This involves training the AI model to copy specific behaviors from a dataset, and it's cheaper than the large-scale reinforcement learning that DeepSeek used for their R1 model, which competes with OpenAI's o1.
Google makes Gemini 2.0 Flash Thinking Experimental available for free through its Google AI Studio platform, though with daily limits. But there's a catch—Google's terms don't allow reverse-engineering its models to create competing services. We're waiting to hear back from Google on this.
The s1 model itself started life as a modest, off-the-shelf AI model from Alibaba's Qwen lab, which anyone can download for free. To train s1, the researchers put together a dataset of just 1,000 carefully chosen questions, along with answers and the "thinking" process behind each one, courtesy of Google's Gemini 2.0. The whole training process took less than 30 minutes on 16 Nvidia H100 GPUs. According to Niklas Muennighoff, a Stanford researcher involved in the project, you could pull this off today for about $20 in compute costs.
The researchers also pulled a clever move to make s1 double-check its work and extend its "thinking" time—they simply told it to "wait." Adding this word during s1's reasoning process helped it come up with slightly more accurate answers, according to the paper.
Looking ahead to 2025, tech giants like Meta, Google, and Microsoft are set to pour hundreds of billions into AI infrastructure, much of which will go toward training the next wave of AI models. While distillation proves to be an effective way to recreate AI capabilities on the cheap, it's not going to lead to the creation of brand-new, groundbreaking AI models anytime soon.
Related article
OpenAI Enhances AI Model Behind Its Operator Agent
OpenAI Takes Operator to the Next LevelOpenAI is giving its autonomous AI agent, Operator, a major upgrade. The upcoming changes mean Operator will soon rely on a model based on o3
OpenAI’s o3 AI model scores lower on a benchmark than the company initially implied
Why Benchmark Discrepancies Matter in AIWhen it comes to AI, numbers often tell the story—and sometimes, those numbers don’t quite add up. Take OpenAI’s o3 model, for instance. The
Ziff Davis, Owner of IGN and CNET, Files Lawsuit Against OpenAI
Ziff Davis Files Copyright Infringement Lawsuit Against OpenAIIn a move that’s sent ripples through the tech and publishing worlds, Ziff Davis—a massive conglomerate behind brands
Comments (5)
0/200
HarryRoberts
April 23, 2025 at 12:00:00 AM GMT
This s1 model is insane! For under $50, you get a reasoning model that rivals OpenAI's? That's a game changer for sure. I'm excited to see how this shakes up the AI world. Can't wait to try it out myself! 🚀
0
DonaldGonzález
April 23, 2025 at 12:00:00 AM GMT
s1モデルが50ドル未満で開発されたなんて信じられない!OpenAIのモデルに匹敵するなんて、これはAI業界に革命をもたらすかもしれないね。早く試してみたい!🚀
0
GregoryAdams
April 22, 2025 at 12:00:00 AM GMT
s1 모델이 50달러도 안 되는 비용으로 개발되었다니 놀랍네요! OpenAI의 모델과 견줄 만하다니, AI 업계에 큰 변화가 있을 것 같아요. 빨리 사용해 보고 싶어요! 🚀
0
JackPerez
April 21, 2025 at 12:00:00 AM GMT
O modelo s1 por menos de $50 é incrível! Rivalizar com o modelo da OpenAI por esse preço é uma revolução. Estou ansioso para ver como isso vai mudar o mundo da IA. Mal posso esperar para testar! 🚀
0
HenryWalker
April 23, 2025 at 12:00:00 AM GMT
Модель s1 за менее чем 50 долларов - это безумие! Соперничать с моделью OpenAI за такую цену - это революция. Жду не дождусь увидеть, как это изменит мир ИИ. Хочу попробовать! 🚀
0
Last Friday, a groundbreaking research paper from AI experts at Stanford and the University of Washington hit the scene, revealing that they managed to develop an AI "reasoning" model, dubbed s1, for under $50 in cloud compute credits. This revelation is shaking up the AI world, as s1 holds its own against top-tier models like OpenAI's o1 and DeepSeek's R1 when it comes to tackling math and coding challenges.
The s1 model, along with all the juicy details of its training data and code, is now up for grabs on GitHub. The team kicked things off with a run-of-the-mill base model and then put it through the wringer with a technique called distillation. This process involves squeezing out the "reasoning" juice from another AI model by training on its responses. In this case, s1 got its smarts from Google's Gemini 2.0 Flash Thinking Experimental model. It's a similar tactic to what Berkeley researchers used to whip up their own AI reasoning model for around $450 just last month.
For some, the idea that a small team of researchers can still make waves in the AI field without a massive budget is thrilling. But s1's emergence also sparks some serious questions about the future of AI model development. If a model that rivals those built with millions can be replicated on a shoestring budget, what's to stop everyone from doing the same?
Not surprisingly, the big players in AI aren't thrilled. OpenAI, for instance, has pointed fingers at DeepSeek, accusing them of using their API data to fuel model distillation. Meanwhile, the s1 team was focused on finding the most straightforward way to achieve solid reasoning performance and something called "test-time scaling," where an AI model gets more time to think before answering. These are the same innovations that OpenAI's o1 model brought to the table, which others like DeepSeek have tried to mimic with their own methods.
The s1 paper suggests that you can distill reasoning models with a relatively small dataset using a technique known as supervised fine-tuning (SFT). This involves training the AI model to copy specific behaviors from a dataset, and it's cheaper than the large-scale reinforcement learning that DeepSeek used for their R1 model, which competes with OpenAI's o1.
Google makes Gemini 2.0 Flash Thinking Experimental available for free through its Google AI Studio platform, though with daily limits. But there's a catch—Google's terms don't allow reverse-engineering its models to create competing services. We're waiting to hear back from Google on this.
The s1 model itself started life as a modest, off-the-shelf AI model from Alibaba's Qwen lab, which anyone can download for free. To train s1, the researchers put together a dataset of just 1,000 carefully chosen questions, along with answers and the "thinking" process behind each one, courtesy of Google's Gemini 2.0. The whole training process took less than 30 minutes on 16 Nvidia H100 GPUs. According to Niklas Muennighoff, a Stanford researcher involved in the project, you could pull this off today for about $20 in compute costs.
The researchers also pulled a clever move to make s1 double-check its work and extend its "thinking" time—they simply told it to "wait." Adding this word during s1's reasoning process helped it come up with slightly more accurate answers, according to the paper.
Looking ahead to 2025, tech giants like Meta, Google, and Microsoft are set to pour hundreds of billions into AI infrastructure, much of which will go toward training the next wave of AI models. While distillation proves to be an effective way to recreate AI capabilities on the cheap, it's not going to lead to the creation of brand-new, groundbreaking AI models anytime soon.
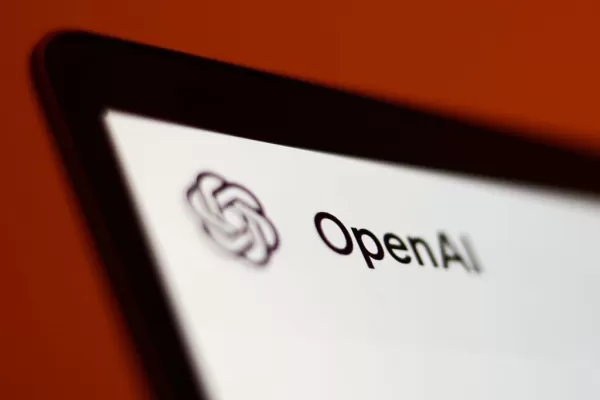
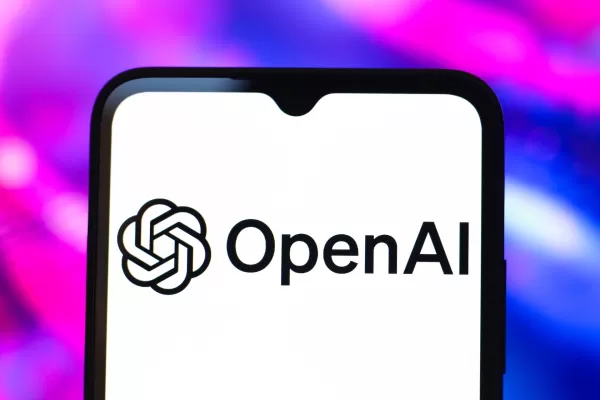
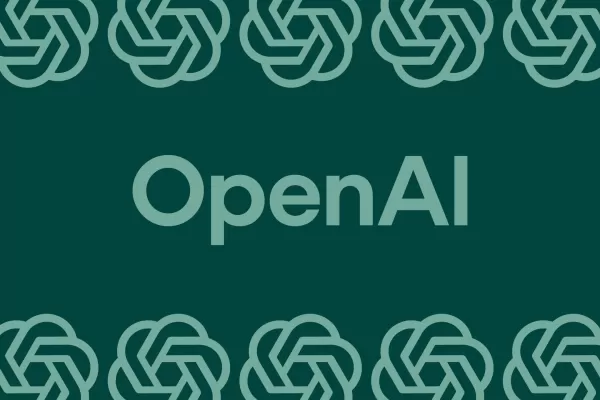

This s1 model is insane! For under $50, you get a reasoning model that rivals OpenAI's? That's a game changer for sure. I'm excited to see how this shakes up the AI world. Can't wait to try it out myself! 🚀




s1モデルが50ドル未満で開発されたなんて信じられない!OpenAIのモデルに匹敵するなんて、これはAI業界に革命をもたらすかもしれないね。早く試してみたい!🚀




s1 모델이 50달러도 안 되는 비용으로 개발되었다니 놀랍네요! OpenAI의 모델과 견줄 만하다니, AI 업계에 큰 변화가 있을 것 같아요. 빨리 사용해 보고 싶어요! 🚀




O modelo s1 por menos de $50 é incrível! Rivalizar com o modelo da OpenAI por esse preço é uma revolução. Estou ansioso para ver como isso vai mudar o mundo da IA. Mal posso esperar para testar! 🚀




Модель s1 за менее чем 50 долларов - это безумие! Соперничать с моделью OpenAI за такую цену - это революция. Жду не дождусь увидеть, как это изменит мир ИИ. Хочу попробовать! 🚀



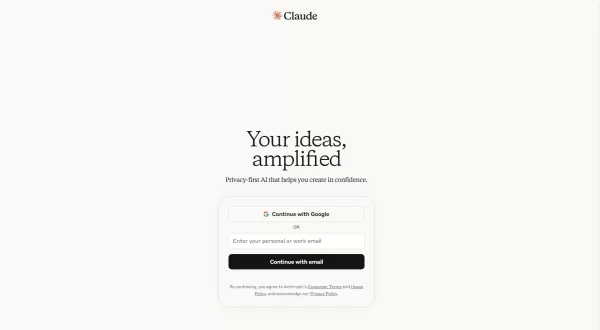
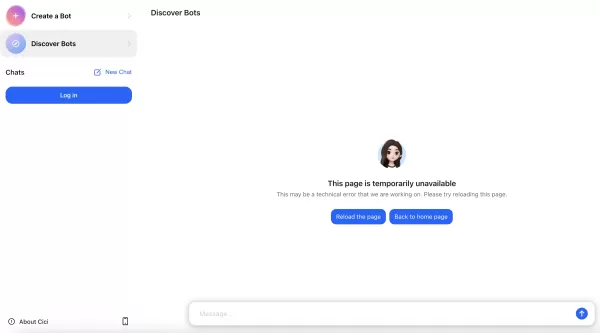
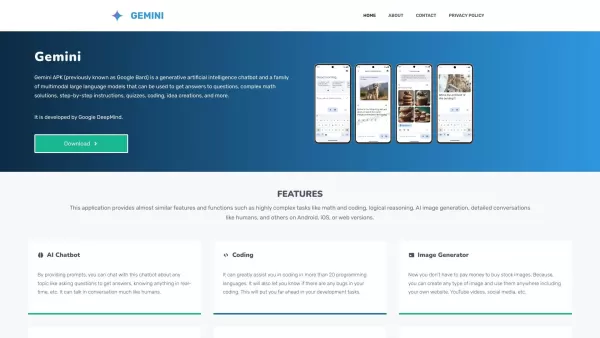
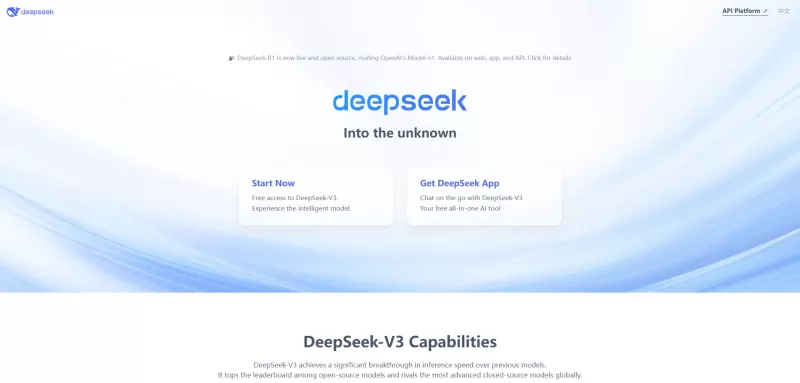
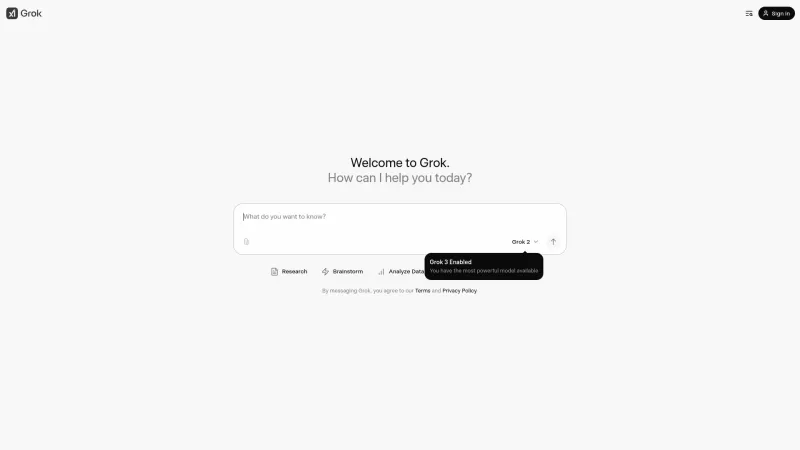
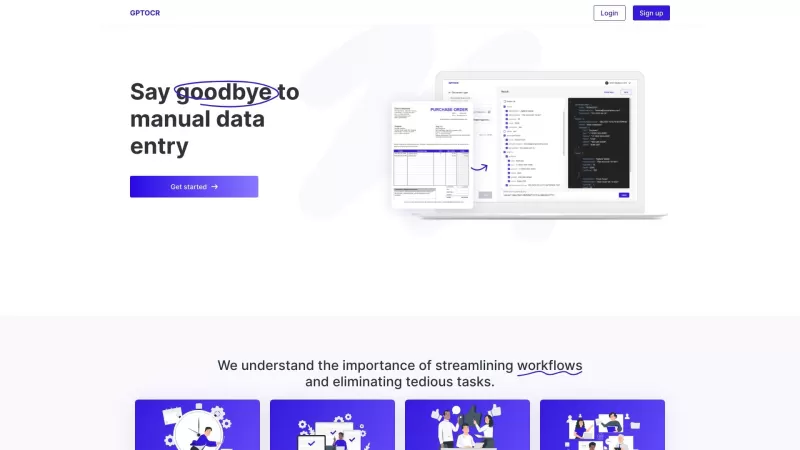
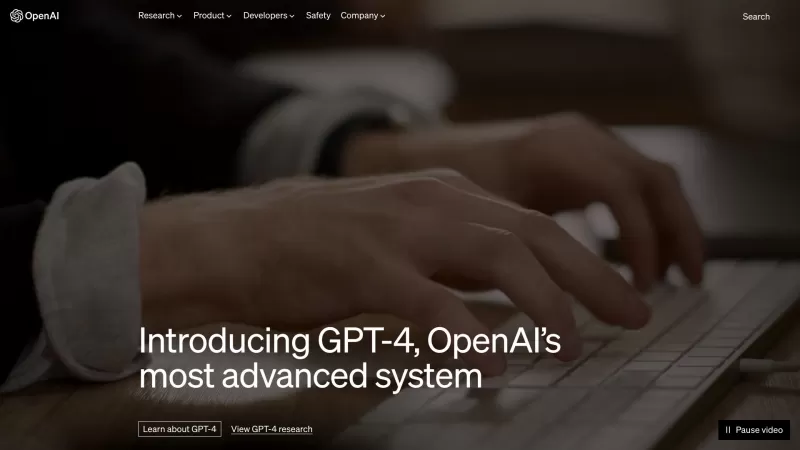
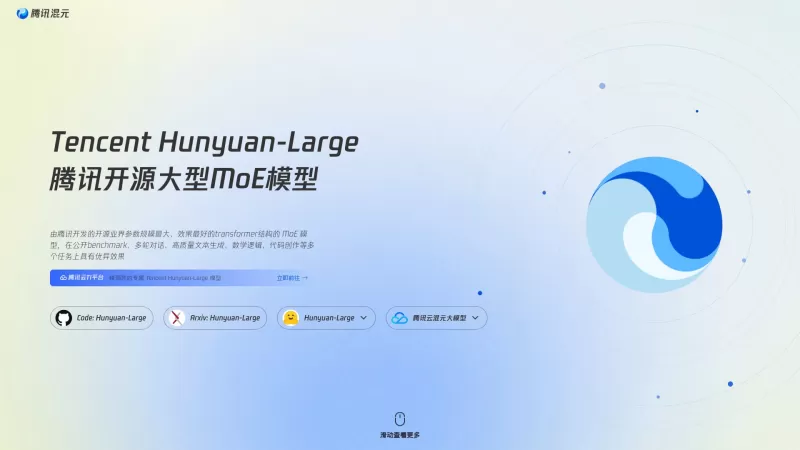
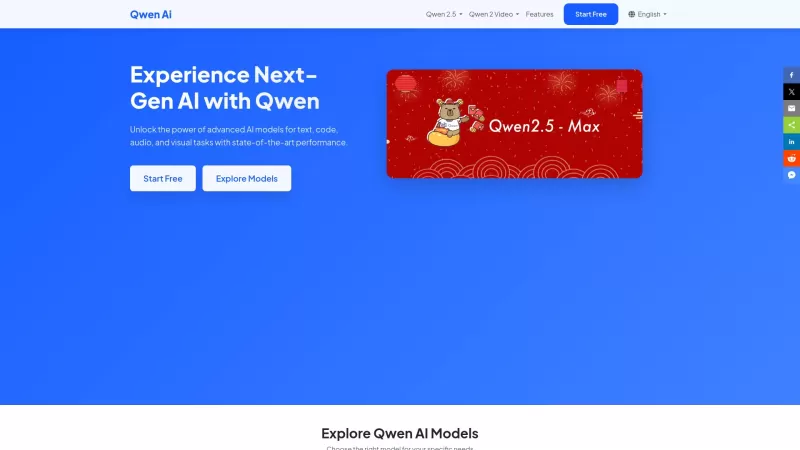
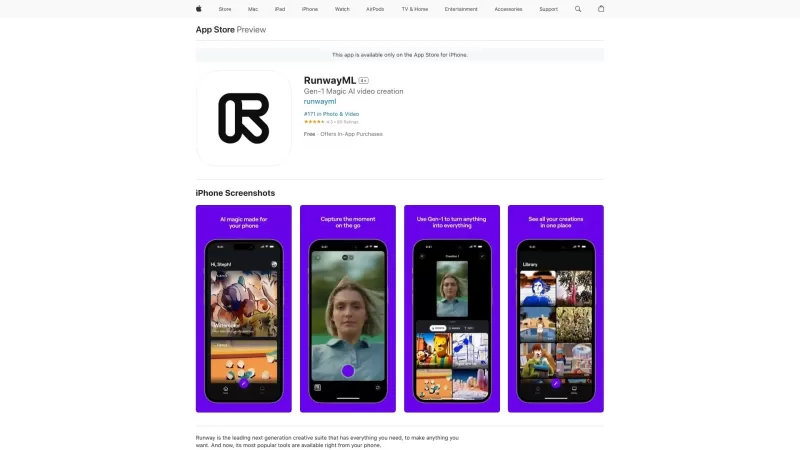