DeepSeek's AIs Uncover True Human Desires
DeepSeek's Breakthrough in AI Reward Models: Enhancing AI Reasoning and Response
Chinese AI startup DeepSeek, in collaboration with Tsinghua University, has achieved a significant milestone in AI research. Their innovative approach to AI reward models promises to revolutionize how AI systems learn from human preferences, potentially leading to more responsive and aligned AI systems. This breakthrough, detailed in their paper "Inference-Time Scaling for Generalist Reward Modeling," showcases a method that outperforms existing reward modeling techniques.
Understanding AI Reward Models
AI reward models play a crucial role in the field of reinforcement learning, particularly for large language models (LLMs). These models act as digital educators, providing feedback that steers AI systems towards outcomes that align with human desires. The DeepSeek paper emphasizes that "Reward modeling is a process that guides an LLM towards human preferences," highlighting its significance as AI applications expand into more complex domains.
Traditional reward models excel in scenarios with clear, verifiable criteria but falter when faced with the diverse and nuanced demands of general domains. DeepSeek's innovation tackles this issue head-on, aiming to refine the accuracy of reward signals across various contexts.
DeepSeek's Innovative Approach
DeepSeek's method integrates two novel techniques:
- Generative Reward Modeling (GRM): This approach allows for greater flexibility and scalability during inference, offering a more detailed representation of rewards through language, rather than relying on simpler scalar or semi-scalar methods.
- Self-Principlized Critique Tuning (SPCT): This learning method enhances GRMs by fostering scalable reward generation through online reinforcement learning, dynamically generating principles that align with the input and responses.
According to Zijun Liu, a researcher from Tsinghua University and DeepSeek-AI, this dual approach enables "principles to be generated based on the input query and responses, adaptively aligning the reward generation process." Moreover, the technique supports "inference-time scaling," allowing performance improvements by leveraging additional computational resources at inference time.
Impact on the AI Industry
DeepSeek's advancement arrives at a pivotal moment in AI development, as reinforcement learning becomes increasingly integral to enhancing large language models. The implications of this breakthrough are profound:
- Enhanced AI Feedback: More precise reward models lead to more accurate feedback, refining AI responses over time.
- Increased Adaptability: The ability to scale performance during inference allows AI systems to adapt to varying computational environments.
- Wider Application: Improved reward modeling in general domains expands the potential applications of AI systems.
- Efficient Resource Use: DeepSeek's method suggests that enhancing inference-time scaling can be more effective than increasing model size during training, allowing smaller models to achieve comparable performance with the right resources.
DeepSeek's Rising Influence
Since its founding in 2023 by entrepreneur Liang Wenfeng, DeepSeek has quickly risen to prominence in the global AI landscape. The company's recent upgrade to its V3 model (DeepSeek-V3-0324) boasts "enhanced reasoning capabilities, optimized front-end web development, and upgraded Chinese writing proficiency." Committed to open-source AI, DeepSeek has released five code repositories, fostering collaboration and innovation in the community.
While rumors swirl about the potential release of DeepSeek-R2, the successor to their R1 reasoning model, the company remains tight-lipped on official channels.
The Future of AI Reward Models
DeepSeek plans to open-source their GRM models, though a specific timeline remains undisclosed. This move is expected to accelerate advancements in reward modeling by enabling wider experimentation and collaboration.
As reinforcement learning continues to shape the future of AI, DeepSeek's work with Tsinghua University represents a significant step forward. By focusing on the quality and scalability of feedback, they are tackling one of the core challenges in creating AI systems that better understand and align with human preferences.
This focus on how and when models learn, rather than just their size, underscores the importance of innovative approaches in AI development. DeepSeek's efforts are narrowing the global technology divide and pushing the boundaries of what AI can achieve.
Related article
Authentic Focusing System Developed for Affordable Augmented Reality
Revolutionizing Projection-Based Augmented RealityResearchers from the prestigious Institute of Electrical and Electronics Engineers (IEEE) have made a groundbreaking leap forward
DeepSeek-GRM: Revolutionizing Scalable, Cost-Efficient AI for Businesses
If you're running a business, you know how tough it can be to integrate Artificial Intelligence (AI) into your operations. The high costs and technical complexity often put advance
New Technique Enables DeepSeek and Other Models to Respond to Sensitive Queries
Removing bias and censorship from large language models (LLMs) like China's DeepSeek is a complex challenge that has caught the attention of U.S. policymakers and business leaders, who see it as a potential national security threat. A recent report from a U.S. Congress select committee labeled DeepS
Comments (0)
0/200
DeepSeek's Breakthrough in AI Reward Models: Enhancing AI Reasoning and Response
Chinese AI startup DeepSeek, in collaboration with Tsinghua University, has achieved a significant milestone in AI research. Their innovative approach to AI reward models promises to revolutionize how AI systems learn from human preferences, potentially leading to more responsive and aligned AI systems. This breakthrough, detailed in their paper "Inference-Time Scaling for Generalist Reward Modeling," showcases a method that outperforms existing reward modeling techniques.
Understanding AI Reward Models
AI reward models play a crucial role in the field of reinforcement learning, particularly for large language models (LLMs). These models act as digital educators, providing feedback that steers AI systems towards outcomes that align with human desires. The DeepSeek paper emphasizes that "Reward modeling is a process that guides an LLM towards human preferences," highlighting its significance as AI applications expand into more complex domains.
Traditional reward models excel in scenarios with clear, verifiable criteria but falter when faced with the diverse and nuanced demands of general domains. DeepSeek's innovation tackles this issue head-on, aiming to refine the accuracy of reward signals across various contexts.
DeepSeek's Innovative Approach
DeepSeek's method integrates two novel techniques:
- Generative Reward Modeling (GRM): This approach allows for greater flexibility and scalability during inference, offering a more detailed representation of rewards through language, rather than relying on simpler scalar or semi-scalar methods.
- Self-Principlized Critique Tuning (SPCT): This learning method enhances GRMs by fostering scalable reward generation through online reinforcement learning, dynamically generating principles that align with the input and responses.
According to Zijun Liu, a researcher from Tsinghua University and DeepSeek-AI, this dual approach enables "principles to be generated based on the input query and responses, adaptively aligning the reward generation process." Moreover, the technique supports "inference-time scaling," allowing performance improvements by leveraging additional computational resources at inference time.
Impact on the AI Industry
DeepSeek's advancement arrives at a pivotal moment in AI development, as reinforcement learning becomes increasingly integral to enhancing large language models. The implications of this breakthrough are profound:
- Enhanced AI Feedback: More precise reward models lead to more accurate feedback, refining AI responses over time.
- Increased Adaptability: The ability to scale performance during inference allows AI systems to adapt to varying computational environments.
- Wider Application: Improved reward modeling in general domains expands the potential applications of AI systems.
- Efficient Resource Use: DeepSeek's method suggests that enhancing inference-time scaling can be more effective than increasing model size during training, allowing smaller models to achieve comparable performance with the right resources.
DeepSeek's Rising Influence
Since its founding in 2023 by entrepreneur Liang Wenfeng, DeepSeek has quickly risen to prominence in the global AI landscape. The company's recent upgrade to its V3 model (DeepSeek-V3-0324) boasts "enhanced reasoning capabilities, optimized front-end web development, and upgraded Chinese writing proficiency." Committed to open-source AI, DeepSeek has released five code repositories, fostering collaboration and innovation in the community.
While rumors swirl about the potential release of DeepSeek-R2, the successor to their R1 reasoning model, the company remains tight-lipped on official channels.
The Future of AI Reward Models
DeepSeek plans to open-source their GRM models, though a specific timeline remains undisclosed. This move is expected to accelerate advancements in reward modeling by enabling wider experimentation and collaboration.
As reinforcement learning continues to shape the future of AI, DeepSeek's work with Tsinghua University represents a significant step forward. By focusing on the quality and scalability of feedback, they are tackling one of the core challenges in creating AI systems that better understand and align with human preferences.
This focus on how and when models learn, rather than just their size, underscores the importance of innovative approaches in AI development. DeepSeek's efforts are narrowing the global technology divide and pushing the boundaries of what AI can achieve.
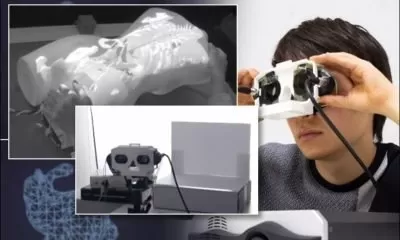
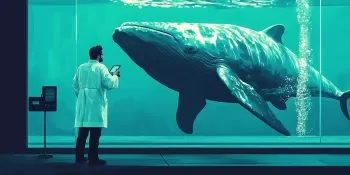
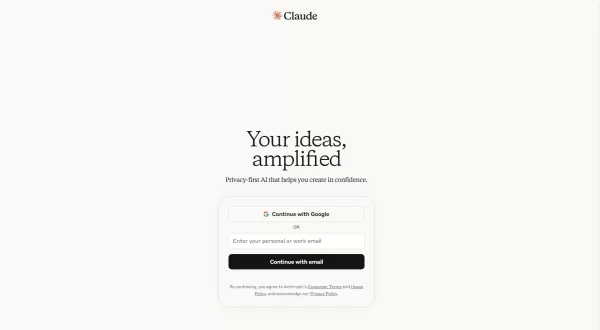
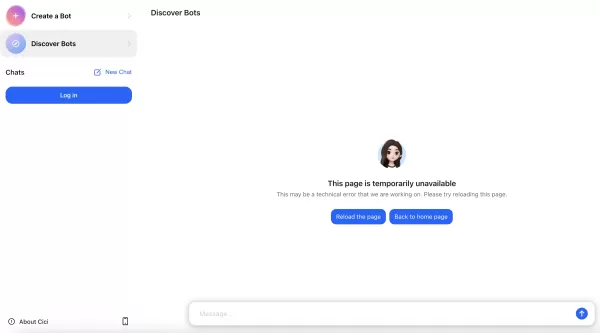
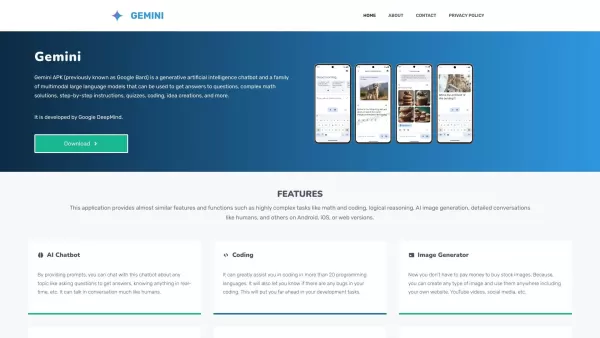
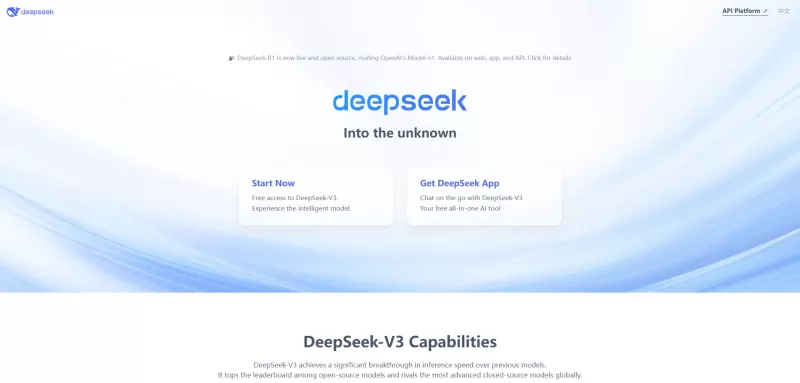
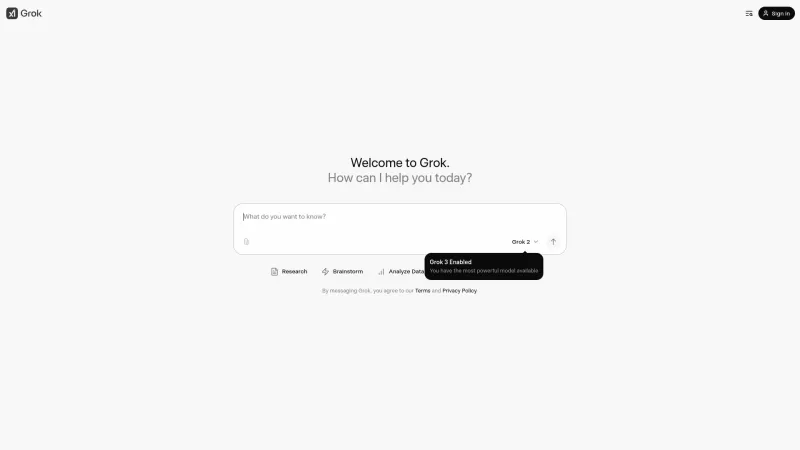
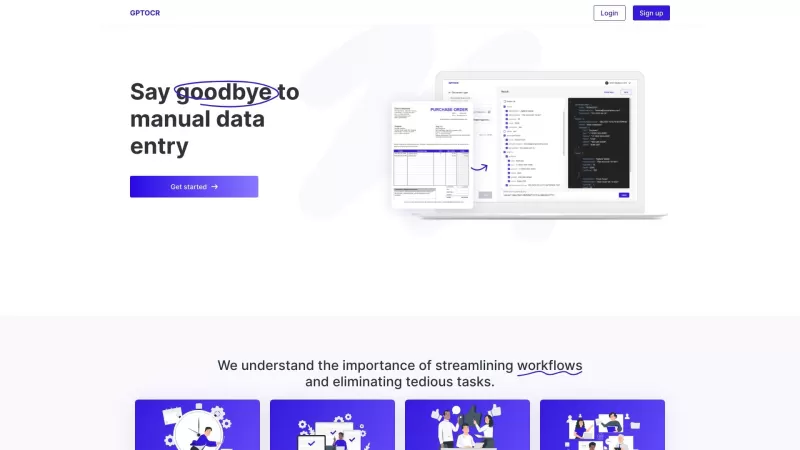
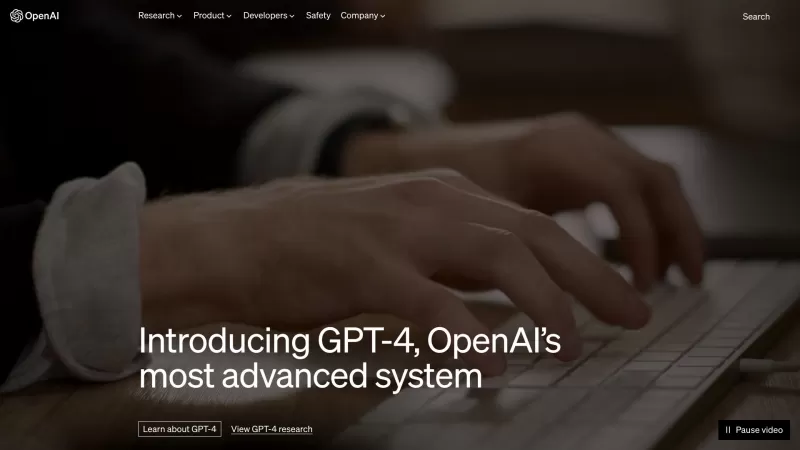
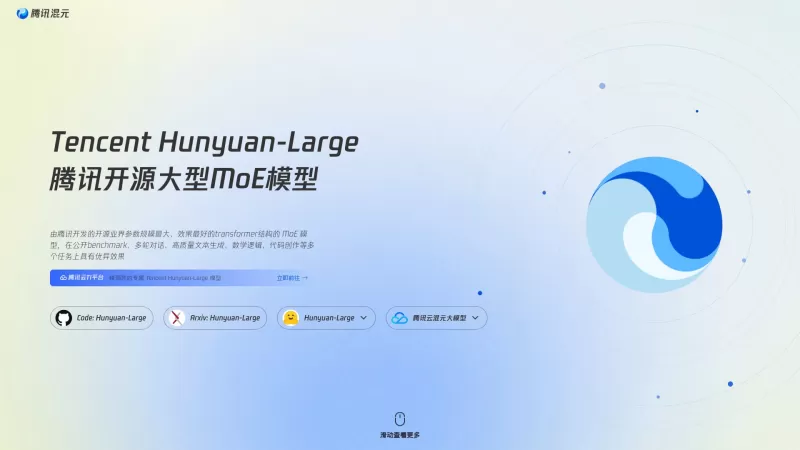
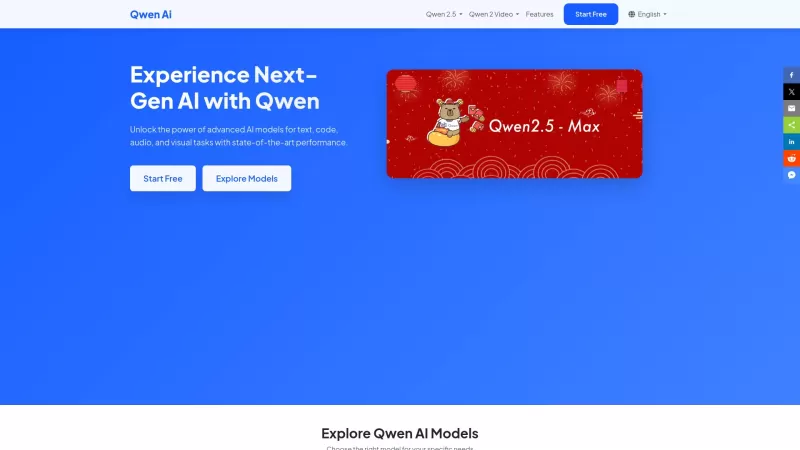
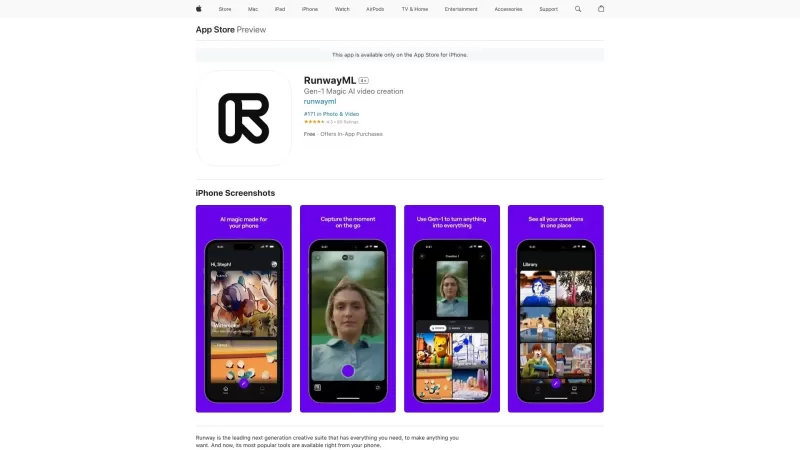