DeepSeek Shakes AI Industry: Next AI Leap May Depend on Increased Compute at Inference, Not More Data
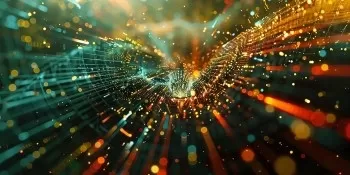
The AI industry is in a state of constant flux, with 2025 bringing some game-changing developments that are shaking things up. One major shakeup came when the Chinese AI lab, DeepSeek, dropped a bombshell with a new model that caused a 17% dip in Nvidia's stock and affected other AI data center stocks. The buzz around DeepSeek's model? It's delivering top-notch performance at a fraction of what it costs other U.S. competitors, stirring up a storm about what this means for the future of AI data centers.
But to really get what DeepSeek's doing, we need to zoom out and look at the bigger picture. The AI world is grappling with a scarcity of training data. The big players have already chewed through most of the public internet data, which means we're hitting a wall in pre-training improvements. As a result, the industry's shifting gears towards "test-time compute" (TTC). Think of it as AI models taking a moment to "think" before answering, like with OpenAI's "o" series. There's hope that TTC can offer the same kind of scaling improvements that pre-training once did, potentially ushering in the next big wave of AI breakthroughs.
These shifts are signaling two big changes: first, smaller-budget labs are now in the game, putting out cutting-edge models. Second, TTC is becoming the new frontier for driving AI forward. Let's break down these trends and what they could mean for the AI landscape and market.
Implications for the AI Industry
We believe the move to TTC and the ramp-up in competition among reasoning models could reshape the AI landscape across several fronts: hardware, cloud platforms, foundation models, and enterprise software.
1. Hardware (GPUs, Dedicated Chips, and Compute Infrastructure)
The shift to TTC might change what hardware AI companies need and how they manage it. Instead of pouring money into ever-larger GPU clusters for training, they might start focusing more on beefing up their inference capabilities to handle TTC demands. While GPUs will still be crucial for inference, the difference between training and inference workloads could affect how these chips are set up and used. With inference workloads being more unpredictable and "spikey," planning for capacity might get trickier.
We also think this shift could boost the market for hardware specifically designed for low-latency inference, like ASICs. As TTC becomes more crucial than training capacity, the reign of general-purpose GPUs might start to wane, opening doors for specialized inference chip makers.
2. Cloud Platforms: Hyperscalers (AWS, Azure, GCP) and Cloud Compute
One major hurdle for AI adoption in businesses, aside from accuracy issues, is the unreliability of inference APIs. Things like inconsistent response times, rate limits, and trouble with concurrent requests can be a real headache. TTC could make these problems even worse. In this scenario, a cloud provider that can guarantee a high quality of service (QoS) to tackle these issues could have a big leg up.
Interestingly, even though new methods might make AI more efficient, they might not reduce the demand for hardware. Following the Jevons Paradox, where more efficiency leads to more consumption, more efficient inference models could drive more developers to use reasoning models, ramping up the need for computing power. We think recent model improvements might spur more demand for cloud AI compute, both for inference and smaller, specialized model training.
3. Foundation Model Providers (OpenAI, Anthropic, Cohere, DeepSeek, Mistral)
If new entrants like DeepSeek can go toe-to-toe with the big guns at a fraction of the cost, the stronghold of proprietary pre-trained models might start to crumble. We can also expect more innovations in TTC for transformer models, and as DeepSeek has shown, these innovations can come from unexpected places outside the usual suspects in AI.
4. Enterprise AI Adoption and SaaS (Application Layer)
Given DeepSeek's roots in China, there's bound to be ongoing scrutiny of their products from a security and privacy standpoint. Their China-based API and chatbot services are unlikely to catch on with enterprise AI customers in the U.S., Canada, or other Western countries. Many companies are already blocking DeepSeek's website and apps. Even when hosted by third parties in Western data centers, DeepSeek's models might face scrutiny, which could limit their adoption in the enterprise. Researchers are flagging issues like jailbreaking, bias, and harmful content generation. While some businesses might experiment with DeepSeek's models, widespread adoption seems unlikely due to these concerns.
On another note, vertical specialization is gaining ground. In the past, vertical applications built on foundation models were all about creating tailored workflows. Techniques like retrieval-augmented generation (RAG), model routing, function calling, and guardrails have been key in tweaking generalized models for these specific use cases. But there's always been the worry that major improvements to the underlying models could make these applications obsolete. Sam Altman once warned that a big leap in model capabilities could "steamroll" these innovations.
However, if we're seeing a plateau in train-time compute gains, the threat of being quickly overtaken lessens. In a world where model performance improvements come from TTC optimizations, new opportunities might emerge for application-layer players. Innovations like structured prompt optimization, latency-aware reasoning strategies, and efficient sampling techniques could offer big performance boosts in specific verticals.
These improvements are particularly relevant for reasoning-focused models like OpenAI's GPT-4o and DeepSeek-R1, which can take several seconds to respond. In real-time applications, cutting down latency and enhancing inference quality within a specific domain could give a competitive edge. As a result, companies with deep domain knowledge might play a crucial role in optimizing inference efficiency and fine-tuning outputs.
DeepSeek's work shows that we're moving away from relying solely on more pre-training to improve model quality. Instead, TTC is becoming increasingly important. While it's unclear whether DeepSeek's models will be widely adopted in enterprise software due to scrutiny, their influence on improving other models is becoming more evident.
We believe DeepSeek's innovations are pushing established AI labs to adopt similar techniques, complementing their existing hardware advantages. The predicted drop in model costs seems to be driving more model usage, fitting the Jevons Paradox pattern.
Pashootan Vaezipoor is technical lead at Georgian.
Related article
DeepSeek-GRM: Revolutionizing Scalable, Cost-Efficient AI for Businesses
If you're running a business, you know how tough it can be to integrate Artificial Intelligence (AI) into your operations. The high costs and technical complexity often put advance
New Technique Enables DeepSeek and Other Models to Respond to Sensitive Queries
Removing bias and censorship from large language models (LLMs) like China's DeepSeek is a complex challenge that has caught the attention of U.S. policymakers and business leaders, who see it as a potential national security threat. A recent report from a U.S. Congress select committee labeled DeepS
Former DeepSeeker and collaborators release new method for training reliable AI agents: RAGEN
The Year of AI Agents: A Closer Look at 2025's Expectations and Realities2025 was heralded by many experts as the year when AI agents—specialized AI systems powered by advanced large language and multimodal models from companies like OpenAI, Anthropic, Google, and DeepSeek—would finally take center
Comments (30)
0/200
JohnRoberts
April 18, 2025 at 12:00:00 AM GMT
DeepSeek's new model is shaking things up, but I'm not sure if it's all that. It's interesting how they're focusing on compute at inference, but I'm still waiting to see real-world results. 🤔💻
0
WalterWhite
April 18, 2025 at 12:00:00 AM GMT
DeepSeekの新しいモデルは話題になっていますが、正直よくわかりません。推論時の計算に焦点を当てているのは面白いですが、実際の結果を見るまで待ちます。🤔💻
0
RogerPerez
April 18, 2025 at 12:00:00 AM GMT
DeepSeek의 새로운 모델이 화제가 되고 있지만, 솔직히 잘 모르겠어요. 추론 시의 계산에 집중하는 건 흥미롭지만, 실제 결과를 보기 전까지는 기다려야 할 것 같아요. 🤔💻
0
PatrickMartinez
April 18, 2025 at 12:00:00 AM GMT
O novo modelo da DeepSeek está causando um impacto, mas não tenho certeza se é tudo isso. É interessante focar no cálculo durante a inferência, mas ainda estou esperando pelos resultados reais. 🤔💻
0
ScottPerez
April 18, 2025 at 12:00:00 AM GMT
El nuevo modelo de DeepSeek está dando que hablar, pero no estoy seguro de que sea para tanto. Es interesante que se enfoquen en el cálculo durante la inferencia, pero aún espero ver resultados reales. 🤔💻
0
SophiaCampbell
April 19, 2025 at 12:00:00 AM GMT
DeepSeek really shook the AI world with their new model! Nvidia's stock took a hit, but honestly, it's exciting to see such big moves. It's like watching a sci-fi movie unfold in real-time. Can't wait to see where this leads, but more compute at inference? Sounds pricey! 🚀
0
The AI industry is in a state of constant flux, with 2025 bringing some game-changing developments that are shaking things up. One major shakeup came when the Chinese AI lab, DeepSeek, dropped a bombshell with a new model that caused a 17% dip in Nvidia's stock and affected other AI data center stocks. The buzz around DeepSeek's model? It's delivering top-notch performance at a fraction of what it costs other U.S. competitors, stirring up a storm about what this means for the future of AI data centers.
But to really get what DeepSeek's doing, we need to zoom out and look at the bigger picture. The AI world is grappling with a scarcity of training data. The big players have already chewed through most of the public internet data, which means we're hitting a wall in pre-training improvements. As a result, the industry's shifting gears towards "test-time compute" (TTC). Think of it as AI models taking a moment to "think" before answering, like with OpenAI's "o" series. There's hope that TTC can offer the same kind of scaling improvements that pre-training once did, potentially ushering in the next big wave of AI breakthroughs.
These shifts are signaling two big changes: first, smaller-budget labs are now in the game, putting out cutting-edge models. Second, TTC is becoming the new frontier for driving AI forward. Let's break down these trends and what they could mean for the AI landscape and market.
Implications for the AI Industry
We believe the move to TTC and the ramp-up in competition among reasoning models could reshape the AI landscape across several fronts: hardware, cloud platforms, foundation models, and enterprise software.
1. Hardware (GPUs, Dedicated Chips, and Compute Infrastructure)
The shift to TTC might change what hardware AI companies need and how they manage it. Instead of pouring money into ever-larger GPU clusters for training, they might start focusing more on beefing up their inference capabilities to handle TTC demands. While GPUs will still be crucial for inference, the difference between training and inference workloads could affect how these chips are set up and used. With inference workloads being more unpredictable and "spikey," planning for capacity might get trickier.
We also think this shift could boost the market for hardware specifically designed for low-latency inference, like ASICs. As TTC becomes more crucial than training capacity, the reign of general-purpose GPUs might start to wane, opening doors for specialized inference chip makers.
2. Cloud Platforms: Hyperscalers (AWS, Azure, GCP) and Cloud Compute
One major hurdle for AI adoption in businesses, aside from accuracy issues, is the unreliability of inference APIs. Things like inconsistent response times, rate limits, and trouble with concurrent requests can be a real headache. TTC could make these problems even worse. In this scenario, a cloud provider that can guarantee a high quality of service (QoS) to tackle these issues could have a big leg up.
Interestingly, even though new methods might make AI more efficient, they might not reduce the demand for hardware. Following the Jevons Paradox, where more efficiency leads to more consumption, more efficient inference models could drive more developers to use reasoning models, ramping up the need for computing power. We think recent model improvements might spur more demand for cloud AI compute, both for inference and smaller, specialized model training.
3. Foundation Model Providers (OpenAI, Anthropic, Cohere, DeepSeek, Mistral)
If new entrants like DeepSeek can go toe-to-toe with the big guns at a fraction of the cost, the stronghold of proprietary pre-trained models might start to crumble. We can also expect more innovations in TTC for transformer models, and as DeepSeek has shown, these innovations can come from unexpected places outside the usual suspects in AI.
4. Enterprise AI Adoption and SaaS (Application Layer)
Given DeepSeek's roots in China, there's bound to be ongoing scrutiny of their products from a security and privacy standpoint. Their China-based API and chatbot services are unlikely to catch on with enterprise AI customers in the U.S., Canada, or other Western countries. Many companies are already blocking DeepSeek's website and apps. Even when hosted by third parties in Western data centers, DeepSeek's models might face scrutiny, which could limit their adoption in the enterprise. Researchers are flagging issues like jailbreaking, bias, and harmful content generation. While some businesses might experiment with DeepSeek's models, widespread adoption seems unlikely due to these concerns.
On another note, vertical specialization is gaining ground. In the past, vertical applications built on foundation models were all about creating tailored workflows. Techniques like retrieval-augmented generation (RAG), model routing, function calling, and guardrails have been key in tweaking generalized models for these specific use cases. But there's always been the worry that major improvements to the underlying models could make these applications obsolete. Sam Altman once warned that a big leap in model capabilities could "steamroll" these innovations.
However, if we're seeing a plateau in train-time compute gains, the threat of being quickly overtaken lessens. In a world where model performance improvements come from TTC optimizations, new opportunities might emerge for application-layer players. Innovations like structured prompt optimization, latency-aware reasoning strategies, and efficient sampling techniques could offer big performance boosts in specific verticals.
These improvements are particularly relevant for reasoning-focused models like OpenAI's GPT-4o and DeepSeek-R1, which can take several seconds to respond. In real-time applications, cutting down latency and enhancing inference quality within a specific domain could give a competitive edge. As a result, companies with deep domain knowledge might play a crucial role in optimizing inference efficiency and fine-tuning outputs.
DeepSeek's work shows that we're moving away from relying solely on more pre-training to improve model quality. Instead, TTC is becoming increasingly important. While it's unclear whether DeepSeek's models will be widely adopted in enterprise software due to scrutiny, their influence on improving other models is becoming more evident.
We believe DeepSeek's innovations are pushing established AI labs to adopt similar techniques, complementing their existing hardware advantages. The predicted drop in model costs seems to be driving more model usage, fitting the Jevons Paradox pattern.
Pashootan Vaezipoor is technical lead at Georgian.
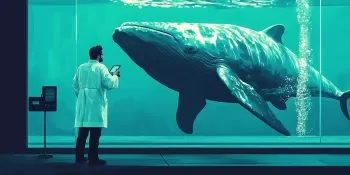
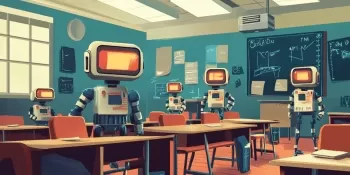

DeepSeek's new model is shaking things up, but I'm not sure if it's all that. It's interesting how they're focusing on compute at inference, but I'm still waiting to see real-world results. 🤔💻




DeepSeekの新しいモデルは話題になっていますが、正直よくわかりません。推論時の計算に焦点を当てているのは面白いですが、実際の結果を見るまで待ちます。🤔💻




DeepSeek의 새로운 모델이 화제가 되고 있지만, 솔직히 잘 모르겠어요. 추론 시의 계산에 집중하는 건 흥미롭지만, 실제 결과를 보기 전까지는 기다려야 할 것 같아요. 🤔💻




O novo modelo da DeepSeek está causando um impacto, mas não tenho certeza se é tudo isso. É interessante focar no cálculo durante a inferência, mas ainda estou esperando pelos resultados reais. 🤔💻




El nuevo modelo de DeepSeek está dando que hablar, pero no estoy seguro de que sea para tanto. Es interesante que se enfoquen en el cálculo durante la inferencia, pero aún espero ver resultados reales. 🤔💻




DeepSeek really shook the AI world with their new model! Nvidia's stock took a hit, but honestly, it's exciting to see such big moves. It's like watching a sci-fi movie unfold in real-time. Can't wait to see where this leads, but more compute at inference? Sounds pricey! 🚀



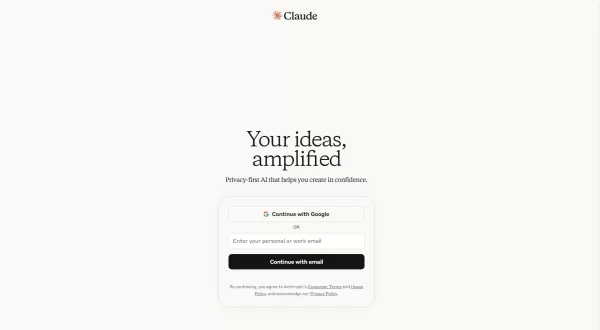
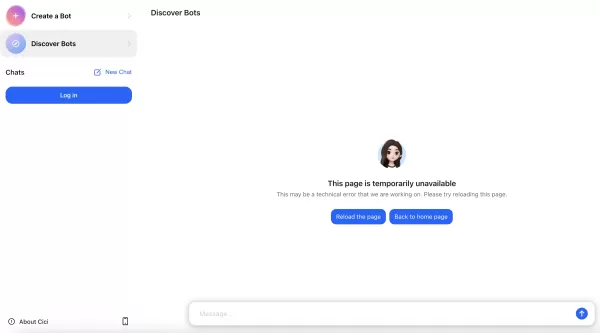
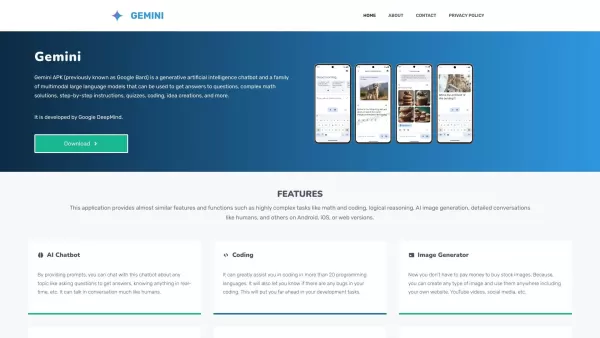
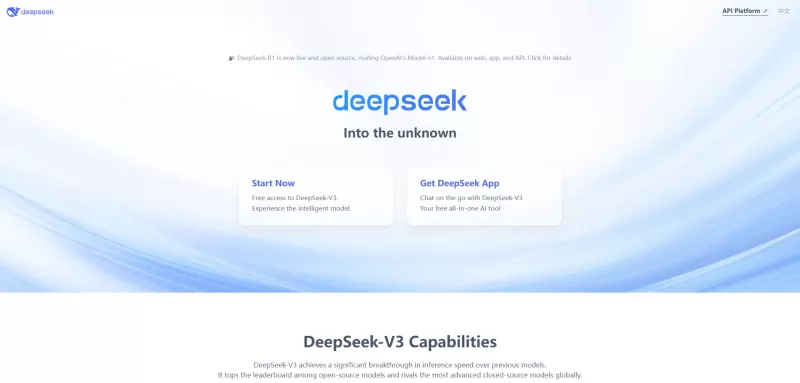
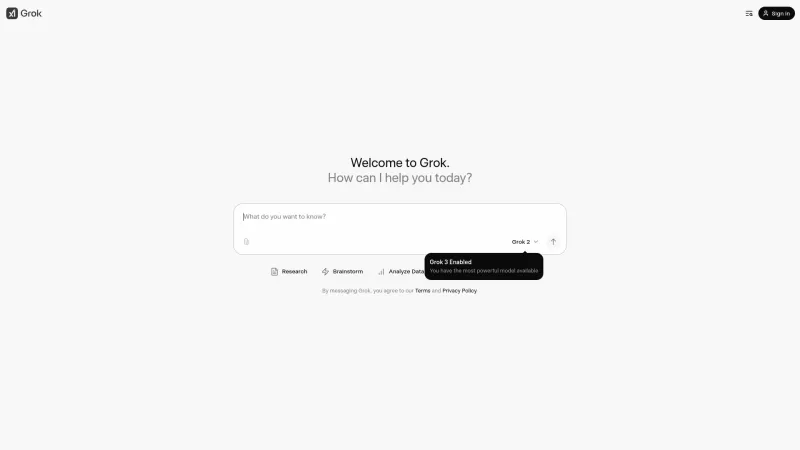
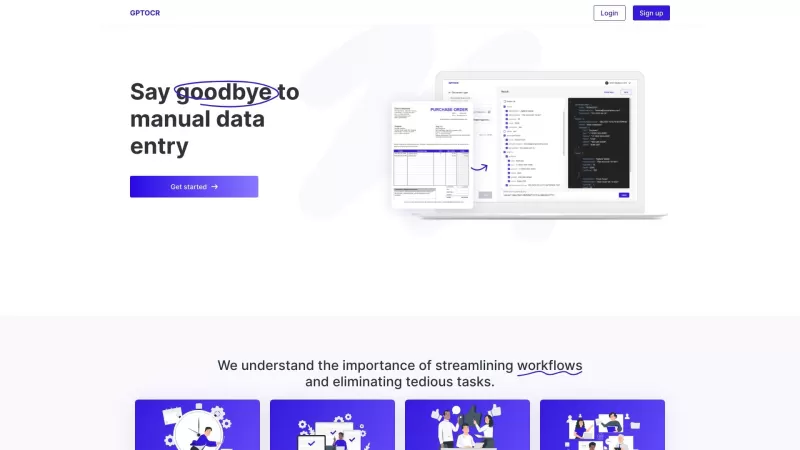
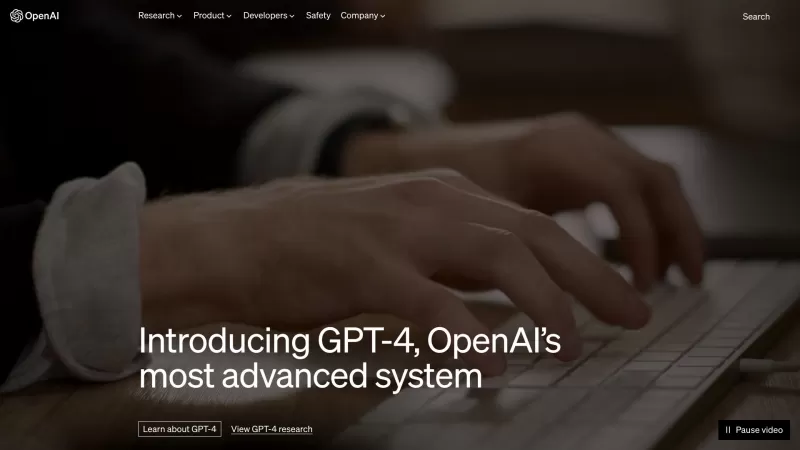
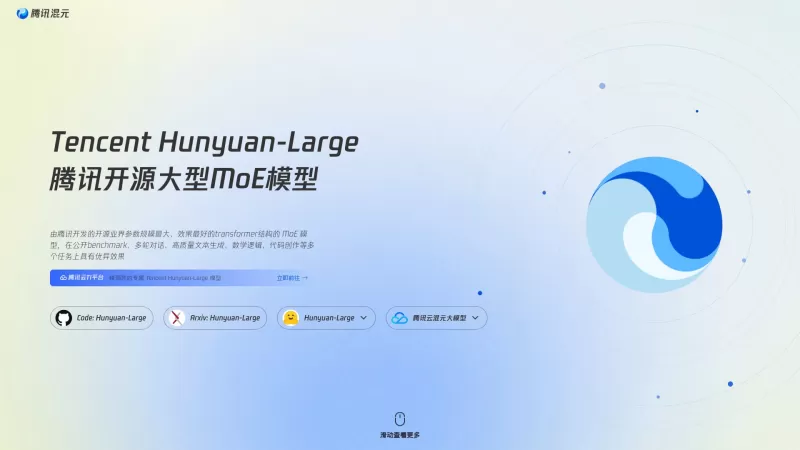
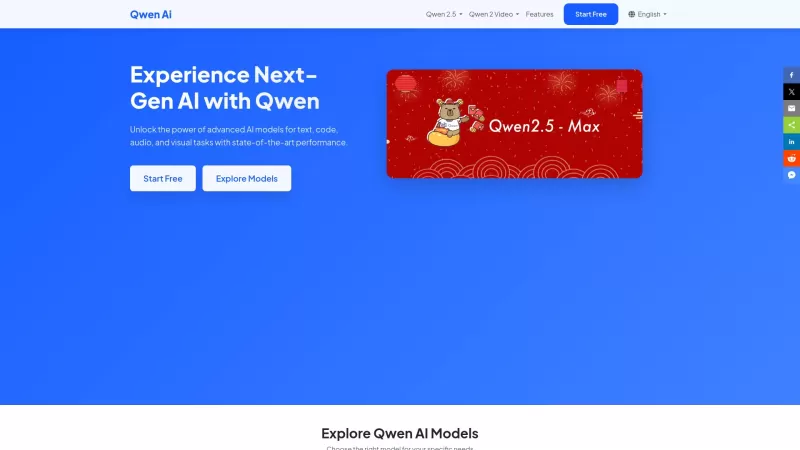
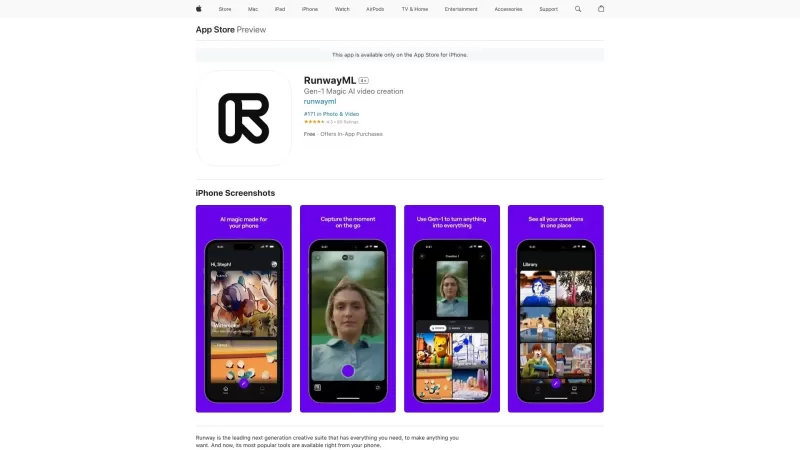