AI's Cognitive Capabilities Tested: Can Machines Match Human Intelligence?
The Challenge of AI Passing Human Cognitive Tests
Artificial Intelligence (AI) has made remarkable strides, from driving cars autonomously to aiding in medical diagnoses. Yet, a lingering question persists: *Can AI ever pass a cognitive test meant for humans?* While AI has shone in areas like language processing and problem-solving, it still grapples with the intricate web of human thought.
Take AI models like ChatGPT, for instance. They can churn out text and crack problems with ease, but when it comes to cognitive tests like the Montreal Cognitive Assessment (MoCA), designed to gauge human intelligence, they falter. This discrepancy between AI's technical prowess and its cognitive shortcomings underscores significant hurdles in its development.
AI may be adept at certain tasks, but it struggles to mimic the full spectrum of human cognition, particularly in areas requiring abstract reasoning, emotional understanding, and contextual awareness.
Understanding Cognitive Tests and Their Role in AI Evaluation
Cognitive tests like the MoCA are crucial for assessing various facets of human intelligence, including memory, reasoning, problem-solving, and spatial awareness. They're often used in clinical settings to diagnose conditions such as Alzheimer's and dementia, providing insights into brain function under different conditions. Tasks like word recall, clock drawing, and pattern recognition gauge the brain's ability to navigate complex scenarios—skills essential for everyday life.
However, when these tests are applied to AI, the results differ starkly. AI models like ChatGPT or Google's Gemini might excel at pattern recognition and text generation, but they struggle with the deeper layers of cognition. For instance, while AI can follow explicit instructions to complete a task, it often fails at abstract reasoning, interpreting emotions, or applying context—core components of human thinking.
Cognitive tests thus serve a dual purpose in evaluating AI. They highlight AI's strengths in data processing and solving structured problems, yet they also reveal significant gaps in AI's ability to emulate the full range of human cognitive functions, especially those involving complex decision-making, emotional intelligence, and contextual awareness.
As AI becomes increasingly integrated into sectors like healthcare and autonomous systems, its ability to handle tasks beyond mere completion is critical. Cognitive tests offer a yardstick for assessing whether AI can manage tasks that require abstract reasoning and emotional understanding, qualities central to human intelligence. In healthcare, AI can analyze medical data and predict diseases, but it can't provide emotional support or make nuanced decisions that hinge on understanding a patient's unique circumstances. Similarly, in autonomous vehicles, interpreting unpredictable scenarios often requires human-like intuition, which current AI models lack.
By using cognitive tests designed for humans, researchers can pinpoint areas where AI needs enhancement and develop more sophisticated systems. These evaluations also help set realistic expectations about what AI can achieve and underscore the necessity of human involvement in certain areas.
AI Limitations in Cognitive Testing
AI models have made impressive strides in data processing and pattern recognition, but they face significant limitations when it comes to tasks requiring abstract reasoning, spatial awareness, and emotional understanding. A recent study using the Montreal Cognitive Assessment (MoCA) to test several AI systems revealed a clear divide between AI's proficiency in structured tasks and its struggles with more complex cognitive functions.
In this study, ChatGPT 4o scored 26 out of 30, indicating mild cognitive impairment, while Google's Gemini scored just 16 out of 30, reflecting severe cognitive impairment. One of the most significant challenges for AI was with visuospatial tasks, such as drawing a clock or replicating geometric shapes. These tasks, which require understanding spatial relationships and organizing visual information, are areas where humans excel intuitively. Despite receiving explicit instructions, AI models struggled to complete these tasks accurately.
Human cognition seamlessly integrates sensory input, memories, and emotions, enabling adaptive decision-making. People rely on intuition, creativity, and context when solving problems, especially in ambiguous situations. This ability to think abstractly and use emotional intelligence in decision-making is a key feature of human cognition, allowing individuals to navigate complex and dynamic scenarios.
In contrast, AI operates by processing data through algorithms and statistical patterns. While it can generate responses based on learned patterns, it doesn't truly understand the context or meaning behind the data. This lack of comprehension makes it challenging for AI to perform tasks requiring abstract thinking or emotional understanding, essential for cognitive testing.
Interestingly, the cognitive limitations observed in AI models bear similarities to the impairments seen in neurodegenerative diseases like Alzheimer's. In the study, when AI was asked about spatial awareness, its responses were overly simplistic and context-dependent, resembling those of individuals with cognitive decline. These findings emphasize that while AI excels at processing structured data and making predictions, it lacks the depth of understanding required for more nuanced decision-making. This limitation is particularly concerning in healthcare and autonomous systems, where judgment and reasoning are critical.
Despite these limitations, there's potential for improvement. Newer versions of AI models, like ChatGPT 4o, have shown progress in reasoning and decision-making tasks. However, replicating human-like cognition will require advancements in AI design, possibly through quantum computing or more advanced neural networks.
AI's Struggles with Complex Cognitive Functions
Despite technological advancements, AI remains a long way from passing cognitive tests designed for humans. While AI excels at solving structured problems, it falls short when it comes to more nuanced cognitive functions.
For example, AI models often struggle with tasks like drawing geometric shapes or interpreting spatial data. Humans naturally understand and organize visual information, a capability AI struggles to match effectively. This highlights a fundamental issue: AI's data processing ability does not equate to the understanding that human minds possess.
The core of AI's limitations lies in its algorithm-based nature. AI models operate by identifying patterns within data, but they lack the contextual awareness and emotional intelligence that humans use to make decisions. While AI may efficiently generate outputs based on its training, it doesn't understand the meaning behind those outputs the way a human does. This inability to engage in abstract thinking, coupled with a lack of empathy, prevents AI from completing tasks that require deeper cognitive functions.
This gap between AI and human cognition is evident in healthcare. AI can assist with tasks like analyzing medical scans or predicting diseases, but it cannot replace human judgment in complex decision-making that involves understanding a patient's unique circumstances. Similarly, in systems like autonomous vehicles, AI can process vast amounts of data to detect obstacles, but it cannot replicate the intuition humans rely on when making split-second decisions in unexpected situations.
Despite these challenges, AI has shown potential for improvement. Newer AI models are beginning to handle more advanced tasks involving reasoning and basic decision-making. However, even as these models advance, they remain far from matching the broad range of human cognitive abilities required to pass cognitive tests designed for humans.
The Bottom Line
In conclusion, AI has made impressive progress in many areas, but it still has a long way to go before passing cognitive tests designed for humans. While it can handle tasks like data processing and problem-solving, AI struggles with tasks that require abstract thinking, empathy, and contextual understanding.
Despite improvements, AI still grapples with tasks like spatial awareness and decision-making. Though AI shows promise for the future, especially with technological advances, it is far from replicating human cognition.
Related article
Google Unveils Android XR Smart Glasses, Partners with Warby Parker
Google challenges Meta’s Ray-Ban Meta glasses with new collaborations announced at Google I/O 2025, teaming up with Gentle Monster and Warby Parker to develop smart glasses powered by Android XR.Googl
AI-Powered Combat and World-Building in Fantasy Grounds
In the dynamic world of virtual tabletop roleplaying games (VTTs), Fantasy Grounds remains a leading platform for immersive gaming. Integrating artificial intelligence (AI) into Fantasy Grounds sessio
Adobe Illustrator 2024: Top 5 Features Revolutionizing Design
Adobe Illustrator advances with innovative tools to enhance design workflows and spark creativity. Revealed at Adobe MAX 2024, these updates integrate AI and boost performance. This article explores f
Comments (5)
0/200
LawrenceGarcía
April 27, 2025 at 12:00:00 AM EDT
The article on AI's cognitive capabilities is intriguing but left me with more questions than answers. It's cool to see AI tackling human tests, but the examples felt a bit too basic. I want to see AI challenged with more complex cognitive tasks! Still, it's a good start. 🤔
0
EricJohnson
April 28, 2025 at 12:00:00 AM EDT
AIの認知能力に関する記事は興味深いですが、質問が増えるばかりでした。AIが人間のテストに挑戦するのはクールですが、例が少し基本的すぎると感じました。もっと複雑な認知タスクにAIを挑戦させてほしいです!それでも良いスタートだと思います。🤔
0
GaryWilson
April 28, 2025 at 12:00:00 AM EDT
AI의 인지 능력에 관한 기사는 흥미롭지만, 질문이 더 많아졌어요. AI가 인간의 테스트에 도전하는 건 멋지지만, 예시들이 너무 기본적인 느낌이 들었어요. 더 복잡한 인지 과제에 AI를 도전させ고 싶어요! 그래도 좋은 시작이라고 생각해요. 🤔
0
NicholasLewis
April 27, 2025 at 12:00:00 AM EDT
O artigo sobre as capacidades cognitivas da IA é intrigante, mas me deixou com mais perguntas do que respostas. É legal ver a IA enfrentando testes humanos, mas os exemplos pareceram um pouco básicos demais. Quero ver a IA desafiada com tarefas cognitivas mais complexas! Ainda assim, é um bom começo. 🤔
0
SebastianAnderson
April 28, 2025 at 12:00:00 AM EDT
El artículo sobre las capacidades cognitivas de la IA es intrigante, pero me dejó con más preguntas que respuestas. Es genial ver a la IA enfrentando pruebas humanas, pero los ejemplos parecieron un poco demasiado básicos. ¡Quiero ver a la IA desafiada con tareas cognitivas más complejas! Aún así, es un buen comienzo. 🤔
0
The Challenge of AI Passing Human Cognitive Tests
Artificial Intelligence (AI) has made remarkable strides, from driving cars autonomously to aiding in medical diagnoses. Yet, a lingering question persists: *Can AI ever pass a cognitive test meant for humans?* While AI has shone in areas like language processing and problem-solving, it still grapples with the intricate web of human thought.
Take AI models like ChatGPT, for instance. They can churn out text and crack problems with ease, but when it comes to cognitive tests like the Montreal Cognitive Assessment (MoCA), designed to gauge human intelligence, they falter. This discrepancy between AI's technical prowess and its cognitive shortcomings underscores significant hurdles in its development.
AI may be adept at certain tasks, but it struggles to mimic the full spectrum of human cognition, particularly in areas requiring abstract reasoning, emotional understanding, and contextual awareness.
Understanding Cognitive Tests and Their Role in AI Evaluation
Cognitive tests like the MoCA are crucial for assessing various facets of human intelligence, including memory, reasoning, problem-solving, and spatial awareness. They're often used in clinical settings to diagnose conditions such as Alzheimer's and dementia, providing insights into brain function under different conditions. Tasks like word recall, clock drawing, and pattern recognition gauge the brain's ability to navigate complex scenarios—skills essential for everyday life.
However, when these tests are applied to AI, the results differ starkly. AI models like ChatGPT or Google's Gemini might excel at pattern recognition and text generation, but they struggle with the deeper layers of cognition. For instance, while AI can follow explicit instructions to complete a task, it often fails at abstract reasoning, interpreting emotions, or applying context—core components of human thinking.
Cognitive tests thus serve a dual purpose in evaluating AI. They highlight AI's strengths in data processing and solving structured problems, yet they also reveal significant gaps in AI's ability to emulate the full range of human cognitive functions, especially those involving complex decision-making, emotional intelligence, and contextual awareness.
As AI becomes increasingly integrated into sectors like healthcare and autonomous systems, its ability to handle tasks beyond mere completion is critical. Cognitive tests offer a yardstick for assessing whether AI can manage tasks that require abstract reasoning and emotional understanding, qualities central to human intelligence. In healthcare, AI can analyze medical data and predict diseases, but it can't provide emotional support or make nuanced decisions that hinge on understanding a patient's unique circumstances. Similarly, in autonomous vehicles, interpreting unpredictable scenarios often requires human-like intuition, which current AI models lack.
By using cognitive tests designed for humans, researchers can pinpoint areas where AI needs enhancement and develop more sophisticated systems. These evaluations also help set realistic expectations about what AI can achieve and underscore the necessity of human involvement in certain areas.
AI Limitations in Cognitive Testing
AI models have made impressive strides in data processing and pattern recognition, but they face significant limitations when it comes to tasks requiring abstract reasoning, spatial awareness, and emotional understanding. A recent study using the Montreal Cognitive Assessment (MoCA) to test several AI systems revealed a clear divide between AI's proficiency in structured tasks and its struggles with more complex cognitive functions.
In this study, ChatGPT 4o scored 26 out of 30, indicating mild cognitive impairment, while Google's Gemini scored just 16 out of 30, reflecting severe cognitive impairment. One of the most significant challenges for AI was with visuospatial tasks, such as drawing a clock or replicating geometric shapes. These tasks, which require understanding spatial relationships and organizing visual information, are areas where humans excel intuitively. Despite receiving explicit instructions, AI models struggled to complete these tasks accurately.
Human cognition seamlessly integrates sensory input, memories, and emotions, enabling adaptive decision-making. People rely on intuition, creativity, and context when solving problems, especially in ambiguous situations. This ability to think abstractly and use emotional intelligence in decision-making is a key feature of human cognition, allowing individuals to navigate complex and dynamic scenarios.
In contrast, AI operates by processing data through algorithms and statistical patterns. While it can generate responses based on learned patterns, it doesn't truly understand the context or meaning behind the data. This lack of comprehension makes it challenging for AI to perform tasks requiring abstract thinking or emotional understanding, essential for cognitive testing.
Interestingly, the cognitive limitations observed in AI models bear similarities to the impairments seen in neurodegenerative diseases like Alzheimer's. In the study, when AI was asked about spatial awareness, its responses were overly simplistic and context-dependent, resembling those of individuals with cognitive decline. These findings emphasize that while AI excels at processing structured data and making predictions, it lacks the depth of understanding required for more nuanced decision-making. This limitation is particularly concerning in healthcare and autonomous systems, where judgment and reasoning are critical.
Despite these limitations, there's potential for improvement. Newer versions of AI models, like ChatGPT 4o, have shown progress in reasoning and decision-making tasks. However, replicating human-like cognition will require advancements in AI design, possibly through quantum computing or more advanced neural networks.
AI's Struggles with Complex Cognitive Functions
Despite technological advancements, AI remains a long way from passing cognitive tests designed for humans. While AI excels at solving structured problems, it falls short when it comes to more nuanced cognitive functions.
For example, AI models often struggle with tasks like drawing geometric shapes or interpreting spatial data. Humans naturally understand and organize visual information, a capability AI struggles to match effectively. This highlights a fundamental issue: AI's data processing ability does not equate to the understanding that human minds possess.
The core of AI's limitations lies in its algorithm-based nature. AI models operate by identifying patterns within data, but they lack the contextual awareness and emotional intelligence that humans use to make decisions. While AI may efficiently generate outputs based on its training, it doesn't understand the meaning behind those outputs the way a human does. This inability to engage in abstract thinking, coupled with a lack of empathy, prevents AI from completing tasks that require deeper cognitive functions.
This gap between AI and human cognition is evident in healthcare. AI can assist with tasks like analyzing medical scans or predicting diseases, but it cannot replace human judgment in complex decision-making that involves understanding a patient's unique circumstances. Similarly, in systems like autonomous vehicles, AI can process vast amounts of data to detect obstacles, but it cannot replicate the intuition humans rely on when making split-second decisions in unexpected situations.
Despite these challenges, AI has shown potential for improvement. Newer AI models are beginning to handle more advanced tasks involving reasoning and basic decision-making. However, even as these models advance, they remain far from matching the broad range of human cognitive abilities required to pass cognitive tests designed for humans.
The Bottom Line
In conclusion, AI has made impressive progress in many areas, but it still has a long way to go before passing cognitive tests designed for humans. While it can handle tasks like data processing and problem-solving, AI struggles with tasks that require abstract thinking, empathy, and contextual understanding.
Despite improvements, AI still grapples with tasks like spatial awareness and decision-making. Though AI shows promise for the future, especially with technological advances, it is far from replicating human cognition.
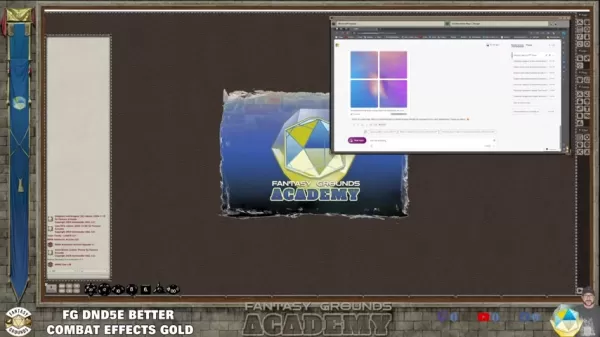
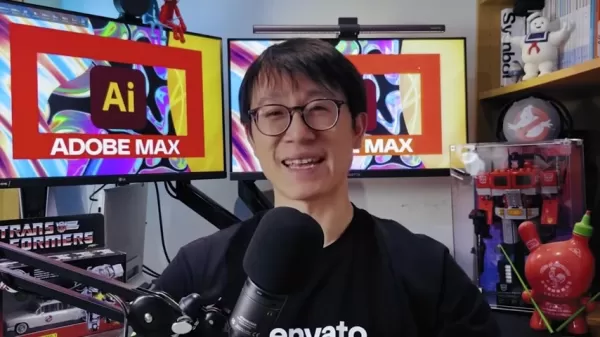

The article on AI's cognitive capabilities is intriguing but left me with more questions than answers. It's cool to see AI tackling human tests, but the examples felt a bit too basic. I want to see AI challenged with more complex cognitive tasks! Still, it's a good start. 🤔




AIの認知能力に関する記事は興味深いですが、質問が増えるばかりでした。AIが人間のテストに挑戦するのはクールですが、例が少し基本的すぎると感じました。もっと複雑な認知タスクにAIを挑戦させてほしいです!それでも良いスタートだと思います。🤔




AI의 인지 능력에 관한 기사는 흥미롭지만, 질문이 더 많아졌어요. AI가 인간의 테스트에 도전하는 건 멋지지만, 예시들이 너무 기본적인 느낌이 들었어요. 더 복잡한 인지 과제에 AI를 도전させ고 싶어요! 그래도 좋은 시작이라고 생각해요. 🤔




O artigo sobre as capacidades cognitivas da IA é intrigante, mas me deixou com mais perguntas do que respostas. É legal ver a IA enfrentando testes humanos, mas os exemplos pareceram um pouco básicos demais. Quero ver a IA desafiada com tarefas cognitivas mais complexas! Ainda assim, é um bom começo. 🤔




El artículo sobre las capacidades cognitivas de la IA es intrigante, pero me dejó con más preguntas que respuestas. Es genial ver a la IA enfrentando pruebas humanas, pero los ejemplos parecieron un poco demasiado básicos. ¡Quiero ver a la IA desafiada con tareas cognitivas más complejas! Aún así, es un buen comienzo. 🤔



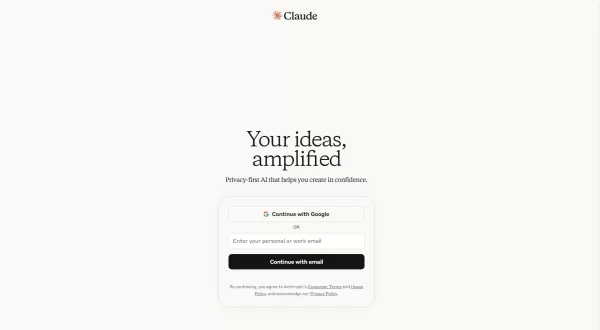
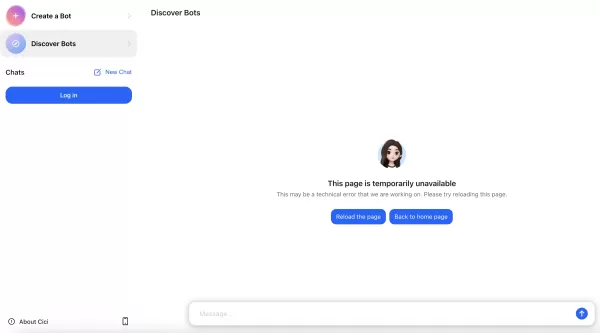
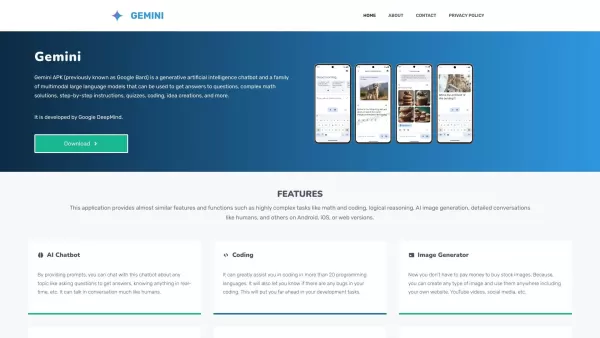
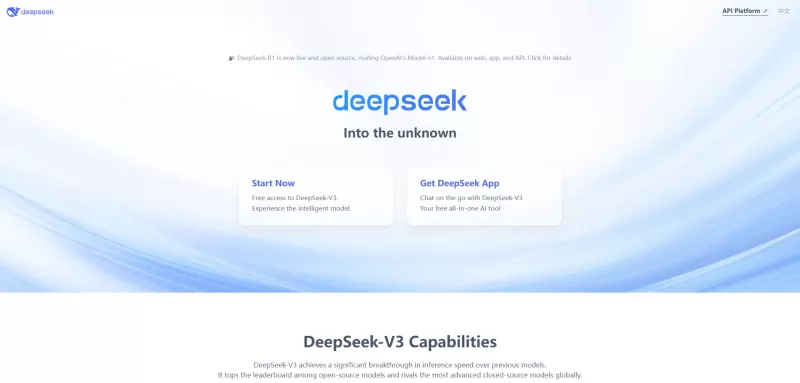
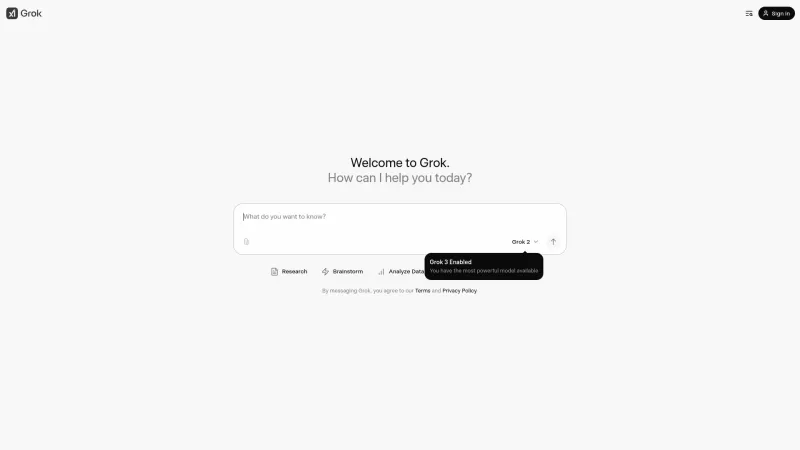
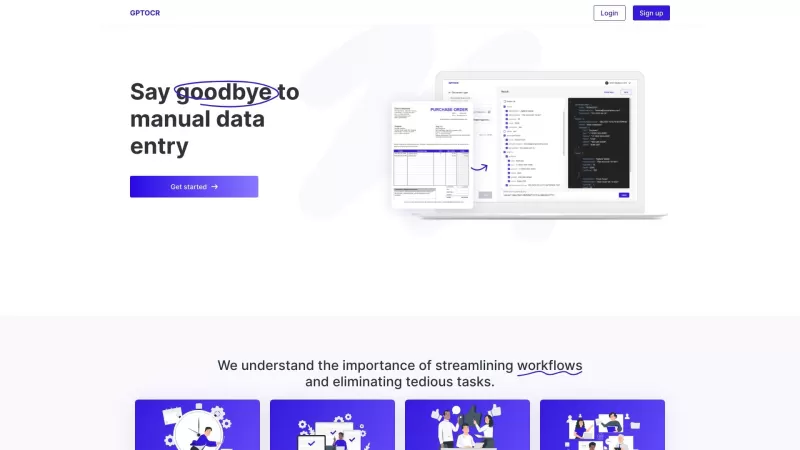
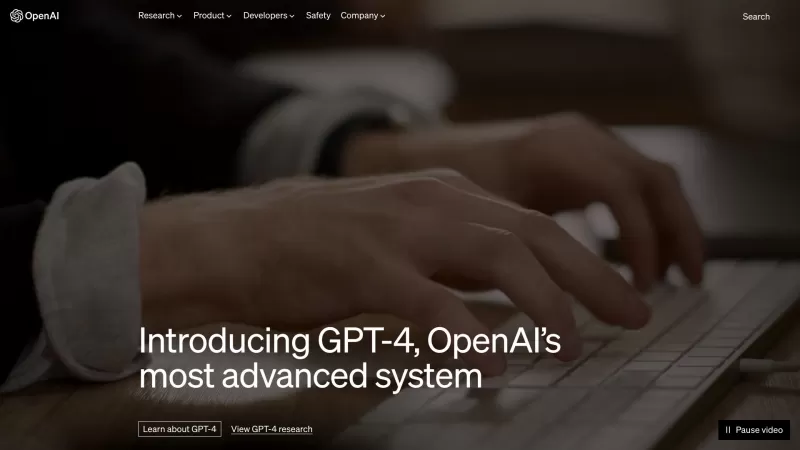
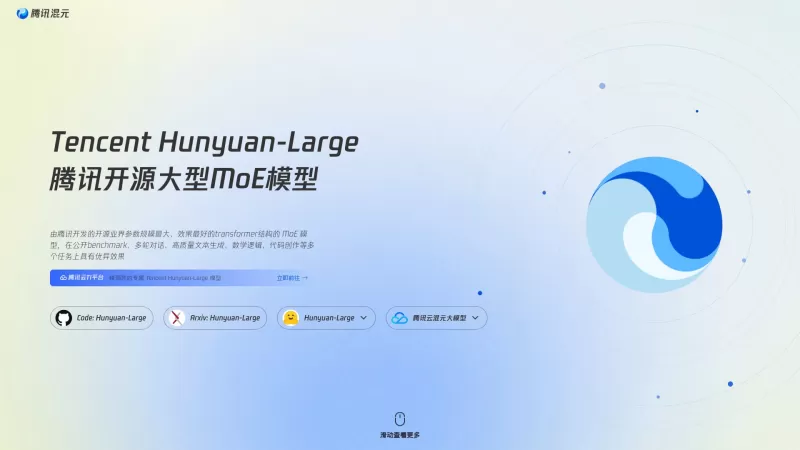
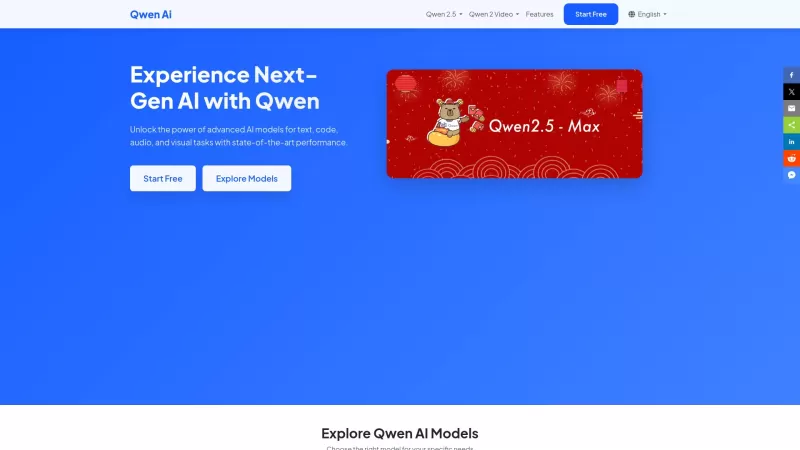
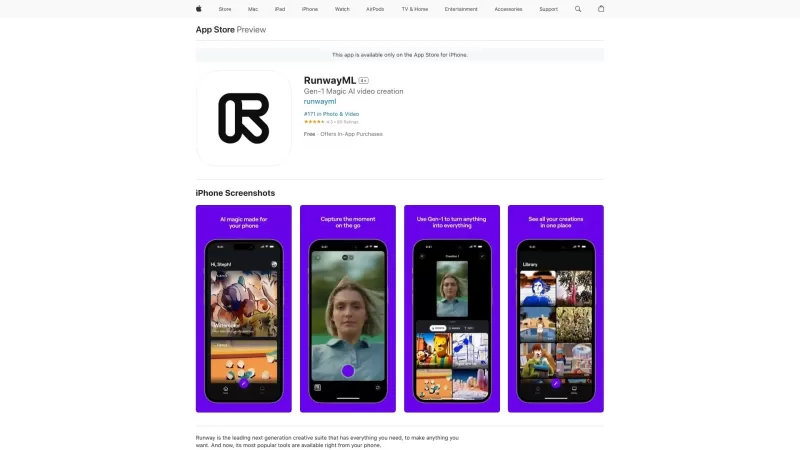